Developing and validating a prediction model for in-hospital mortality in patients with ventilator-associated pneumonia in the ICU
Introduction
Ventilator-associated pneumonia (VAP) is a very common nosocomial infection in the intensive care unit (ICU) (1-3). VAP is defined as pneumonia occurring in patients who were subject to invasive mechanical ventilation at least 48 hours before the onset of infection (4,5), and its incidence is reported to be 5–40% of patients receiving invasive mechanical ventilation (2,6). VAP is associated with some adverse events, such as atelectasis, aspiration, pulmonary edema, venous thromboembolism, delirium, and acute respiratory distress syndrome (ARDS), leading to continuing high morbidity and mortality in the ICU (7,8).
Previous clinical studies have investigated a wide range of risk factors associated with the mortality of patients with VAP, including age, inappropriate initial treatment, duration of mechanical ventilation, length of hospital stay, comorbidities, and invasive operations (9-15). However, the judgment criteria of these risk factors vary between studies and cannot be applied directly to clinical practice, which requires a scoring system convenient for clinical use. At present, only a few scoring systems for predicting the mortality risk of VAP are recognized as effective, such as the Acute Physiology and Chronic Health Evaluation (APACHE II), the Simplified Acute Physiology Score (SAPS II), and the Sequential Organ Failure Assessment (SOFA). These models have limitations when used for predicting the risk of VAP mortality. APACHE II and SAPS II are both time-consuming to use and require large amounts of data for accurate analysis (16). In addition, APACHE II does not include the effects of mechanical ventilation and the use of vasopressor drugs and so may not be appropriate for identifying organ dysfunction and mortality associated with VAP (17). Moreover, Gaudet et al. reported that SOFA has low sensitivity, poor accuracy, and cannot be used to distinguish ventilator-associated tracheobronchitis (VAT) from VAP (18). Furthermore, the number of measurement time points (19) and the age proportion of the sample (20) affects the discriminative power of these models.
Given the multiple limitations of these prediction models mentioned above, we aimed to develop a comprehensive prediction model with demographic and clinical data in a large sample cohort to assess the in-hospital mortality risk of VAP patients from the Medical Information Mart for Intensive Care (MIMIC-III) database between 2001 and 2012. We present the following article in accordance with the TRIPOD reporting checklist (available at https://apm.amegroups.com/article/view/10.21037/apm-22-502/rc).
Methods
Study design
This was a retrospective cohort study. Using the International Classification of Diseases (ICD-9) diagnosis code (99731: ventilator-associated pneumonia) and keywords (VAP, ventilator-associated pneumonia, or venting-associated pneumonia), we selected adult individuals diagnosed with VAP from the MIMIC-III database. The MIMIC-III database was developed by an interdisciplinary team of data scientists and practicing physicians from the Laboratory for Computational Physiology at Massachusetts Institute of Technology (21). It contains detailed information of 38,597 distinct adult patients and 49,785 hospital admissions downloaded from archives from critical care information systems, hospital electronic health record databases, and the United States Social Security Administration Death Master File. The data are publicly available at https://physionet.org/content/mimiciii/1.4/. The study was conducted in accordance with the Declaration of Helsinki (as revised in 2013).
Potential variables
The demographic and clinical data were extracted including gender; ethnicity; last care unit; oral care; duration of invasive ventilation; comorbidities including chronic obstructive pulmonary disease (COPD), lung cancer, heart failure, diabetes mellitus, hypertension, and septicemia; hospital length of stay (LOS), and ICU LOS. The SOFA and SAPS II scores were also collected to assess the severity of the disease. In addition, we collected the laboratory data from 6 hours before to 24 hours after admission to the ICU, including white blood cell (WBC) count, red blood cell (RBC) count, blood urea nitrogen (BUN), mean arterial pressure (MAP), fraction of inspired oxygen (FIO2), oxygen saturation (SPO2), and bacteria that are common pathogens carried by patients with VAP (Klebsiella pneumonia, Pseudomonas aeruginosa, Acinetobacter baumannii, yeast, Aspergillus fumigatus, Staphylococcus, and microorganism quantity) (22).
Among the variables mentioned above, the categorical variables included gender, ethnicity, last care unit, oral care, comorbidities, lung cancer, heart failure, diabetes mellitus, hypertension, septicemia, Klebsiella pneumoniae, pseudomonas aeruginosa, Acinetobacter baumannii, yeast, Aspergillus fumigatus, Staphylococcus, and microorganism quantity. Continuous variables included invasive ventilation, hospital LOS, ICU LOS, MAP, FIO2, SPO2, WBC, RBC, BUN, SAPS II score, and SOFA score. The primary outcome was in-hospital mortality of hospitalized patients with VAP.
Development and validation of the prediction model
Random sampling was used to divide the data into training sets and test sets, with 70% of the data forming the training set and the remaining 30% forming the test set. An equilibrium test was performed between the training set and the test set. After ensuring that the 2 groups of data were balanced, univariate logistic regression analysis was used to screen for possible predictors in the training set. According to the results of the univariate logistic regression analysis, factors with P<0.05 were included in the multivariate logistic analysis. The backward selection method was adopted to establish the model in the training set, and internal validation was carried out in the test set. The receiver operating characteristic (ROC) curve and the calibration curve were plotted to evaluate the performance of the model, and the DeLong test was used to compare the area under the curve (AUC) between the single-index models (SAPS II, and SOFA) and the combined model. The value of AUC was over 0.8, indicating the performance of the model was good.
Statistical analysis
Enumeration data were expressed as the number of cases and the constituent ratio [N (%)]. A chi-square test was used for comparison between groups. Measurement data in normal distribution were expressed as mean and standard deviation (mean ± SD), and a t-test was used for comparison between groups. Non-normal distributed measurement data were expressed as median and interquartile range [M (Q1, Q3)], and the Wilcoxon rank-sum test was used for comparison.
Data were randomly grouped by using the “scikit-learn” module in Python 3 (Python Software Foundation, Wilmington, DE, USA), and univariate and multivariate logistic regression analyses were performed using R version 4.0.2 (The R Foundation for Statistical Computing, Vienna, Austria). P<0.05 was considered statistically significant.
Results
Patient characteristics
A total of 8,182 adult individuals diagnosed with VAP were selected from the MIMIC database. Individuals who were missing any information relating to laboratory data (n=1,278), clinical data (n=285), or disease severity data (n=6) were excluded. Finally, 6,613 eligible subjects were enrolled in the dataset.
The whole dataset was divided into a training set (n=4,629) and a test set (n=1,984). In the training set, there were 2,788 (60.23%) males and 1,841 (39.77%) females. Among them, 3,297 (71.22%) subjects were white people, 330 (7.13%) were black people, and 1,002 (21.65%) were of other ethnicities. The median duration of invasive ventilation was 24.908 (9.779, 94.738) hours. The median hospital LOS was 8.70 (5.25, 14.65) days, and the median ICU LOS was 3.24 (1.76, 6.98) days. More details are shown in Table 1. The results suggested that there were no significant differences in the distribution between the training set and the test set (all P>0.05).
Table 1
Variables | Total (n=6,613) | Training set (n=4,629) | Test set (n=1,984) | Statistics | P |
---|---|---|---|---|---|
Gender, n (%) | χ2=2.620 | 0.105 | |||
Male | 4,025 (60.86) | 2,788 (60.23) | 1,237 (62.35) | ||
Female | 2,588 (39.14) | 1,841 (39.77) | 747 (37.65) | ||
Ethnicity, n (%) | χ2=5.282 | 0.071 | |||
White | 4,759 (71.96) | 3,297 (71.22) | 1,462 (73.69) | ||
Black | 447 (6.76) | 330 (7.13) | 117 (5.90) | ||
Other races | 1,407 (21.28) | 1,002 (21.65) | 405 (20.41) | ||
Last care unit, n (%) | χ2=6.521 | 0.164 | |||
Coronary care unit | 401 (6.06) | 298 (6.44) | 103 (5.19) | ||
Cardiac surgery recovery unit | 2,271 (34.34) | 1,566 (33.83) | 705 (35.53) | ||
Medical ICU | 1,848 (27.94) | 1,306 (28.21) | 542 (27.32) | ||
Surgical ICU | 1,119 (16.92) | 792 (17.11) | 327 (16.48) | ||
Trauma/surgical ICU | 974 (14.73) | 667 (14.41) | 307 (15.47) | ||
Oral care, n (%) | χ2=1.034 | 0.596 | |||
Swab | 3,888 (58.79) | 2,740 (59.19) | 1,148 (57.86) | ||
Toothbrush | 2,533 (38.30) | 1,755 (37.91) | 778 (39.21) | ||
None | 192 (2.90) | 134 (2.89) | 58 (2.92) | ||
Comorbidities, n (%) | |||||
COPD | χ2=0.395 | 0.530 | |||
No | 5,894 (89.13) | 4,133 (89.28) | 1,761 (88.76) | ||
Yes | 719 (10.87) | 496 (10.72) | 223 (11.24) | ||
Lung cancer, n (%) | χ2=0.150 | 0.698 | |||
No | 6,509 (98.43) | 4,558 (98.47) | 1,951 (98.34) | ||
Yes | 104 (1.57) | 71 (1.53) | 33 (1.66) | ||
Heart failure, n (%) | χ2=0.120 | 0.729 | |||
No | 4,844 (73.25) | 3,385 (73.13) | 1,459 (73.54) | ||
Yes | 1,769 (26.75) | 1,244 (26.87) | 525 (26.46) | ||
Diabetes mellitus, n (%) | χ2=0.205 | 0.651 | |||
No | 4,942 (74.73) | 3,452 (74.57) | 1,490 (75.10) | ||
Yes | 1,671 (25.27) | 1,177 (25.43) | 494 (24.90) | ||
Hypertension, n (%) | χ2=2.339 | 0.126 | |||
No | 3,108 (47.00) | 2,204 (47.61) | 904 (45.56) | ||
Yes | 3,505 (53.00) | 2,425 (52.39) | 1,080 (54.44) | ||
Septicemia, n (%) | χ2=0.033 | 0.856 | |||
No | 5,401 (81.67) | 3,778 (81.62) | 1,623 (81.80) | ||
Yes | 1,212 (18.33) | 851 (18.38) | 361 (18.20) | ||
Invasive ventilation, hours, M (Q1, Q3) | 26.183 (10.217, 95.95) | 24.908 (9.779, 94.738) | 26.617 (10.517, 97.30) | Z=−0.913 | 0.361 |
Hospital LOS, days, M (Q1, Q3) | 8.70 (5.25, 14.64) | 8.70 (5.25, 14.65) | 8.71 (5.24, 14.62) | Z=0.025 | 0.980 |
ICU LOS, days, M (Q1, Q3) | 3.23 (1.74, 6.95) | 3.24 (1.76, 6.98) | 3.18 (1.69, 6.78) | Z=−1.066 | 0.286 |
Laboratory test | |||||
MAP, mmHg, mean ± SD | 82.28±18.79 | 82.16±18.89 | 82.54±18.57 | t=0.736 | 0.462 |
FIO2, %, M (Q1, Q3) | 100.00 (50.00, 100.00) | 100.00 (50.00, 100.00) | 100.00 (50.00, 100.00) | Z=0.791 | 0.429 |
SPO2, %, mean ± SD | 97.87±4.76 | 97.89±4.57 | 97.82±5.16 | t=−0.554 | 0.579 |
WBC, 109/L, M (Q1, Q3) | 11.70 (8.30, 15.90) | 11.70 (8.20, 15.90) | 11.80 (8.40, 15.90) | Z=0.588 | 0.556 |
RBC, 1012/L, mean ± SD | 3.61±0.80 | 3.62±0.80 | 3.61±0.80 | t=−0.523 | 0.601 |
BUN, mg/dL, M (Q1, Q3) | 19.00 (14.00, 29.00) | 19.00 (14.00, 29.00) | 18.50 (14.00, 29.00) | Z=−0.245 | 0.806 |
Klebsiella pneumoniae, n (%) | χ2=0.035 | 0.852 | |||
No | 6,372 (96.36) | 4,459 (96.33) | 1,913 (96.42) | ||
Yes | 241 (3.64) | 170 (3.67) | 71 (3.58) | ||
Pseudomonas aeruginosa, n (%) | χ2=0.222 | 0.638 | |||
No | 6,338 (95.84) | 4,433 (95.77) | 1,905 (96.02) | ||
Yes | 275 (4.16) | 196 (4.23) | 79 (3.98) | ||
Acinetobacter baumannii, n (%) | χ2=0.355 | 0.551 | |||
No | 6,574 (99.41) | 4,600 (99.37) | 1,974 (99.50) | ||
Yes | 39 (0.59) | 29 (0.63) | 10 (0.50) | ||
Yeast, n (%) | χ2=1.839 | 0.175 | |||
No | 3,439 (52.00) | 2,382 (51.46) | 1,057 (53.28) | ||
Yes | 3,174 (48.00) | 2,247 (48.54) | 927 (46.72) | ||
Aspergillus fumigatus, n (%) | χ2=0.168 | 0.681 | |||
No | 6,590 (99.65) | 4,612 (99.63) | 1,978 (99.70) | ||
Yes | 23 (0.35) | 17 (0.37) | 6 (0.30) | ||
Staphylococcus, n (%) | χ2=1.839 | 0.175 | |||
No | 3,439 (52.00) | 2,382 (51.46) | 1,057 (53.28) | ||
Yes | 3,174 (48.00) | 2,247 (48.54) | 927 (46.72) | ||
Microorganism quantity, n (%) | χ2=2.485 | 0.289 | |||
None | 3,439 (52.00) | 2,382 (51.46) | 1,057 (53.28) | ||
1-2 | 2,156 (32.60) | 1,536 (33.18) | 620 (31.25) | ||
≥3 | 1,018 (15.39) | 711 (15.36) | 307 (15.47) | ||
SAPS II score, M (Q1, Q3) | 39 [31, 51] | 39 [31, 51] | 40 [31, 51] | Z=0.300 | 0.764 |
SOFA score, M (Q1, Q3) | 7 [5, 10] | 7 [5, 10] | 7 [5, 10] | Z=0.297 | 0.766 |
Outcome | |||||
In-hospital mortality, n (%) | χ2=0.000 | 0.990 | |||
No | 5,437 (82.22) | 3,806 (82.22) | 1,631 (82.21) | ||
Yes | 1,176 (17.78) | 823 (17.78) | 353 (17.79) |
COPD, chronic obstructive pulmonary disease; ICU, intensive care unit; LOS, length of stay; MAP, mean arterial pressure; FIO2, fraction of inspired oxygen; SPO2, oxygen saturation; WBC, white blood cell; RBC, red blood cell; BUN, blood urea nitrogen; SOFA, Sequential Organ Failure Assessment; SAPS II, Simplified Acute Physiology Score.
Developing the prediction model
According to the univariate logistic regression analysis, female gender (P=0.003); other races (P<0.001); oral care of toothbrush (P=0.004); comorbidities of COPD (P=0.002), lung cancer (P<0.001), hypertension (P=0.001), and septicemia (P<0.001); hospital LOS (P=0.021); ICU LOS (P<0.001); FIO2 (P=0.012); SPO2 (P<0.001); WBC (P<0.001); BUN (P<0.001); bacteria including Klebsiella pneumonia (P<0.001), Pseudomonas aeruginosa (P<0.001), yeast (P<0.001), Aspergillus fumigatus (P<0.001), and Staphylococcus (P<0.001); microorganism quantity (P<0.001); SAPS II score (P<0.001); SOFA score (P<0.001); and duration of invasive ventilation (P<0.001) were found to be potential predictors of the mortality of VAP (Table 2).
Table 2
Variables | β | OR (95% CI) | P value |
---|---|---|---|
Gender | |||
Male | Ref | ||
Female | 0.230 | 1.258 (1.080, 1.465) | 0.003 |
Ethnicity | |||
White | Ref | ||
Black | −0.001 | 0.999 (0.729, 1.345) | 0.994 |
Other races | 0.414 | 1.513 (1.270, 1.798) | <0.001 |
Oral care | |||
Swab | Ref | ||
Toothbrush | −0.235 | 0.790 (0.672, 0.927) | 0.004 |
None | 0.297 | 1.346 (0.882, 2.001) | 0.154 |
COPD | |||
No | Ref | ||
Yes | 0.352 | 1.422 (1.132, 1.775) | 0.002 |
Lung cancer | |||
No | Ref | ||
Yes | 1.186 | 3.273 (2.009, 5.263) | <0.001 |
Heart failure | |||
No | Ref | ||
Yes | 0.160 | 1.174 (0.993, 1.385) | 0.059 |
Diabetes mellitus | |||
No | Ref | ||
Yes | 0.067 | 1.070 (0.900, 1.268) | 0.440 |
Hypertension | |||
No | Ref | ||
Yes | −0.249 | 0.779 (0.670, 0.906) | 0.001 |
Septicemia | |||
No | Ref | ||
Yes | 1.411 | 4.099 (3.467, 4.845) | <0.001 |
Hospital length of stay, days | −0.009 | 0.991 (0.984, 0.998) | 0.021 |
ICU length of stay, days | 0.036 | 1.036 (1.027, 1.046) | <0.001 |
MAP, mmHg | 0.001 | 1.001 (0.997, 1.005) | 0.744 |
FIO2, % | −0.004 | 0.996 (0.993, 0.999) | 0.012 |
SPO2, % | −0.081 | 0.923 (0.908, 0.937) | <0.001 |
WBC, 109/L | 0.024 | 1.024 (1.014, 1.034) | <0.001 |
RBC, 1012/L | 0.057 | 1.059 (0.965, 1.163) | 0.228 |
BUN, mg/dL | 0.023 | 1.024 (1.020, 1.027) | <0.001 |
Klebsiella pneumoniae | |||
No | Ref | ||
Yes | 0.832 | 2.299 (1.641, 3.183) | <0.001 |
Pseudomonas aeruginosaa | |||
No | Ref | ||
Yes | 0.648 | 1.912 (1.379, 2.616) | <0.001 |
Acinetobacter baumannii | |||
No | Ref | ||
Yes | 0.739 | 2.093 (0.903, 4.482) | 0.067 |
Yeast | |||
No | Ref | ||
Yes | 0.977 | 2.657 (2.267, 3.121) | <0.001 |
Aspergillus fumigatus | |||
No | Ref | ||
Yes | 2.149 | 8.580 (3.255, 24.968) | <0.001 |
Staphylococcus | |||
No | Ref | ||
Yes | 0.977 | 2.657 (2.267, 3.121) | <0.001 |
Microorganism quantity | |||
None | Ref | ||
1−2 | 0.794 | 2.212 (1.855, 2.640) | <0.001 |
≥3 | 1.325 | 3.763 (3.071, 4.610) | <0.001 |
SAPS II score | 0.065 | 1.067 (1.061, 1.073) | <0.001 |
SOFA score | 0.233 | 1.263 (1.235, 1.291) | <0.001 |
Invasive ventilation, hours | 0.002 | 1.002 (1.002, 1.003) | <0.001 |
OR, odds ratio; CI, confidence interval; COPD, chronic obstructive pulmonary disease; ICU, intensive care unit; MAP, mean arterial pressure; FIO2, fraction of inspired oxygen; SPO2, oxygen saturation; WBC, white blood cell; RBC, red blood cell; BUN, blood urea nitrogen; SOFA, Sequential Organ Failure Assessment; SAPS II, Simplified Acute Physiology Score.
All variables with statistical significance were enrolled in the multivariate logistic regression for further analysis. The results showed that other races [odds ratio (OR) =1.637, 95% CI: 1.330–2.011, P<0.001], lung cancer (OR =2.202, 95% CI: 1.226–3.884, P=0.007), septicemia (OR =2.000, 95% CI: 1.620–2.466, P<0.001), hospital LOS (OR =0.928, 95% CI: 0.915–0.941, P<0.001), FIO2 (OR =0.992, 95% CI: 0.988–0.995, P<0.001), SPO2 (OR =0.969, 95% CI: 0.952–0.985, P<0.001), SAPS II score (OR =1.048, 95% CI: 1.041–1.055, P<0.001), SOFA score (OR =1.109, 95% CI: 1.077–1.143, P<0.001), and duration of invasive ventilation (OR =1.004, 95% CI: 1.004–1.005, P<0.001) were all independently associated with the mortality of VAP (Table 3).
Table 3
Variables | β | OR (95% CI) | P |
---|---|---|---|
Intercept | −0.700 | <0.001 | |
Ethnicity | |||
White | Ref | ||
Black | −0.226 | 0.798 (0.553, 1.133) | 0.218 |
Other races | 0.493 | 1.637 (1.330, 2.011) | <0.001 |
Lung cancer | |||
No | Ref | ||
Yes | 0.789 | 2.202 (1.226, 3.884) | 0.007 |
Septicemia | |||
No | Ref | ||
Yes | 0.693 | 2.000 (1.620, 2.466) | <0.001 |
Hospital length of stay, days | −0.074 | 0.928 (0.915, 0.941) | <0.001 |
FIO2, % | −0.008 | 0.992 (0.988, 0.995) | <0.001 |
SPO2, % | −0.032 | 0.969 (0.952, 0.985) | <0.001 |
SAPS II score | 0.047 | 1.048 (1.041, 1.055) | <0.001 |
SOFA score | 0.104 | 1.109 (1.077, 1.143) | <0.001 |
Invasive ventilation, hours | 0.004 | 1.004 (1.004, 1.005) | <0.001 |
OR, odds ratio; CI, confidence interval; FIO2, fraction of inspired oxygen; SPO2, oxygen saturation; SOFA, Sequential Organ Failure Assessment; SAPS II, Simplified Acute Physiology Score.
The algorithm for the mortality risk was as follows: lnP/(1-P) = −0.700 + 0.493 Other Ethnicity + 0.789 Lung Cancer (Yes) + 0.693 Septicemia (Yes) – 0.074 Hospital LOS – 0.008 FIO2 – 0.032 SPO2 + 0.104 SOFA Score + 0.047 SAPS II + 0.004 Invasive Ventilation. The nomogram was also plotted, as shown in Figure 1.
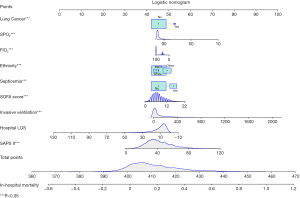
Example
As shown in Figure 2, we randomly took 1 patient from the training set as an example. The patient was Caucasian without lung cancer or septicemia. The duration of invasive ventilation was 38.5 hours, and the hospital LOS was 24.35 days, with an FIO2 of 100% and an SPO2 of 83%. The SOFA score was 9 points, and the SAPS II score was 47 points. The total score of this patient was calculated to be 409 points, and the corresponding predicted probability of death was 0.1416, which was consistent with the fact that the patient reported no in-hospital death.
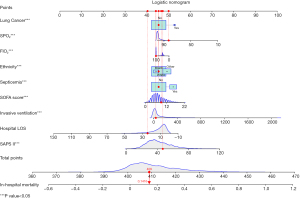
Validating the prediction model
According to the ROC analysis, the AUC in the training set was 0.837 (95% CI: 0.821, 0.853), with a sensitivity of 0.734 (95% CI: 0.704, 0.764) and a specificity of 0.796 (95% CI: 0.783, 0.809), which suggested that the model performed well. The cutoff value was 0.185. In the test set, the AUC was 0.817 (95% CI: 0.791, 0.843), with a sensitivity of 0.657 (95% CI: 0.608, 0.707) and a specificity of 0.797 (95% CI: 0.778, 0.817; Table 4 and Figure 3). The calibration curve also confirmed the good calibration of the model (Figure 4).
Table 4
Indicator | Training set | Test set |
---|---|---|
AUC (95% CI) | 0.837 (0.821, 0.853) | 0.817 (0.791, 0.843) |
Sensitivity (95% CI) | 0.734 (0.704, 0.764) | 0.657 (0.608, 0.707) |
Specificity (95% CI) | 0.796 (0.783, 0.809) | 0.797 (0.778, 0.817) |
PPV (95% CI) | 0.438 (0.412, 0.822) | 0.412 (0.371, 0.838) |
NPV (95% CI) | 0.933 (0.924, 0.941) | 0.915 (0.900, 0.929) |
Accuracy (95% CI) | 0.785 (0.773, 0.797) | 0.772 (0.754, 0.791) |
AUC, area under the curve; CI, confidence interval; PPV, positive predictive value; NPV, negative predictive value.
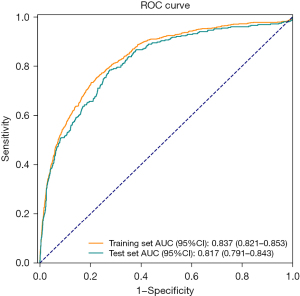
Table 5 presents the result of the comparison between the combined model and the single-index models. The AUCs of SAPS II and SOFA were 0.760 (95% CI: 0.733–0.787) and 0.685 (95% CI: 0.653–0.716), respectively, which were both lower than the AUC of the combined prediction model, indicating that the combined model had a better ability to predict the individual death risk of patients with VAP in the ICU than SAPS II or SOFA.
Table 5
Model | Cutoff | AUC (95% CI) | Accuracy (95% CI) | Specificity (95% CI) | Sensitivity (95% CI) | PPV (95% CI) | NPV (95% CI) |
---|---|---|---|---|---|---|---|
Combined model | 0.185 | 0.817 (0.791, 0.843) | 0.772 (0.754, 0.791) | 0.797 (0.778, 0.817) | 0.657 (0.608, 0.707) | 0.412 (0.371, 0.838) | 0.915 (0.900, 0.929) |
SAPS II | 0.173 | 0.760 (0.733, 0.787)* | 0.710 (0.689, 0.730) | 0.722 (0.701, 0.744) | 0.652 (0.602, 0.701) | 0.337 (0.301, 0.372) | 0.905 (0.890, 0.921) |
SOFA | 0.218 | 0.685 (0.653, 0.716)* | 0.722 (0.702, 0.742) | 0.779 0.759, 0.799) | 0.459 (0.407, 0.511) | 0.310 (0.271, 0.350) | 0.869 (0.852, 0.887) |
*, the difference between this model and the combined model was statistically significant (P<0.05). AUC, area under the curve; PPV, positive predictive value; NPV, negative predictive value; SAPS II, Simplified Acute Physiology Score; SOFA, Sequential Organ Failure Assessment.
Discussion
Currently, VAP is a common cause of nosocomial infections and even death of ICU patients during hospitalization. Therefore, rapid and accurate identification of patients at a higher risk of death from VAP is critical for better prevention and management of VAP. In this study, lung cancer, septicemia, other races, hospital LOS, FIO2, SPO2, SAPS II score, SOFA score, and duration of invasive ventilation were identified as independent predictors of VAP. We developed a prediction model for mortality in patients with VAP using the following algorithm: lnP/(1-P) = −0.700 + 0.493 Other Ethnicity + 0.789 Lung Cancer (Yes) + 0.693 Septicemia (Yes) – 0.074 Hospital LOS – 0.008 FIO2 – 0.032 SPO2 + 0.104 SOFA Score + 0.047 SAPS II + 0.004 Invasive Ventilation. Internal verification confirmed that the model had good predictive value, and that its curve was close to the ideal curves.
Previous studies have reported that nosocomial infection is associated with invasive mechanical ventilation in the ICU, including reintubation, tracheostomy, and fiberoptic bronchoscopy (13,23-25). The present study suggested that the duration of invasive ventilation was a predictor of VAP mortality, which was consistent with previous studies. ICU patients receiving invasive ventilation are easily exposed to stress, which leads to a decrease in patients’ resistance. A longer duration of invasive ventilation may lead to multiple stress responses, which can further reduce the function of the body barrier and increase the risk of respiratory infections (12,26). Furthermore, bacteria colonized in the stomach can be colonized in the pharynx and then enter the lower respiratory tract to cause infection (12). Prolonged mechanical ventilation can also lead to a variety of complications. In our study, lung cancer and septicemia significantly increased the risk of VAP mortality. Studies have reported that comorbidities, such as diabetes, respiratory diseases, and renal failure, etc., might be a risk factor for VAP (15,27,28). These diseases can lead to immune suppression, which can impair vital organs such as the heart, liver, kidney, and lungs and make the patient more vulnerable to infection.
To date, only a few prediction models, such as APACHE II, SAPS II, and SOFA, can effectively score the mortality risk of patients with VAP in the ICU. Čiginskienė et al. observed that the AUCs of APACHE II, SAPS II, and SOFA were 0.62 (95% CI: 0.54–0.84), 0.68 (95% CI: 0.54–0.84), and 0.73 (95% CI: 0.59–0.86), respectively, when predicting in-hospital mortality in drug-resistant patients with VAP caused by Acinetobacter baumannii (29). Studies assessing the discriminative power of the APACHE II score for VAP have reported AUCs of 0.53 (95% CI: 0.47–0.58) (30) and 0.743 (95% CI: 0.628–0.857) (31), while a study by Gursel et al. evaluating the discriminative power of the SOFA score for VAP reported an AUC of 0.71 (95% CI: 0.58–0.84) (32). Compared to these single-index models, our multivariate prediction model had a higher AUC [0.817 (95% CI: 0.791, 0.843)] based on a relatively large sample size, and its accuracy and reliability were confirmed by internal validation. This suggests that our model may perform better than these single-index models when predicting the risk of VAP mortality. In addition, our model contains relatively comprehensive variables (e.g., demographic, clinical, and laboratory data) and predictors that are easy to obtain in clinical practice with high clinical practical value. Therefore, our model might assist in clinical decision-making by predicting patient outcomes, recommending timely intervention measures, and improving the survival and prognosis of patients with VAP.
This study has some limitations. First, our study was a single-center study with a study population from the United States only, which may have affected the general applicability of our results. Secondly, the data of the medical cost and administration time were not available in the database. Thirdly, our model lacks external validation. A multicenter, prospective study including different populations is necessary for further validation.
Conclusions
In this study, we developed and validated a practical VAP prediction model with good performance. This model could provide ancillary data to help clinicians predict the individual death risk of patients with VAP in the ICU and make informed decisions regarding VAP diagnosis.
Acknowledgments
Funding: None.
Footnote
Reporting Checklist: The authors have completed the TRIPOD reporting checklist. Available at https://apm.amegroups.com/article/view/10.21037/apm-22-502/rc
Conflicts of Interest: All authors have completed the ICMJE uniform disclosure form (available at https://apm.amegroups.com/article/view/10.21037/apm-22-502/coif). The authors have no conflicts of interest to declare.
Ethical Statement: The authors are accountable for all aspects of the work in ensuring that questions related to the accuracy or integrity of any part of the work are appropriately investigated and resolved. The study was conducted in accordance with the Declaration of Helsinki (as revised in 2013).
Open Access Statement: This is an Open Access article distributed in accordance with the Creative Commons Attribution-NonCommercial-NoDerivs 4.0 International License (CC BY-NC-ND 4.0), which permits the non-commercial replication and distribution of the article with the strict proviso that no changes or edits are made and the original work is properly cited (including links to both the formal publication through the relevant DOI and the license). See: https://creativecommons.org/licenses/by-nc-nd/4.0/.
References
- Li Z, Ma X, Gao S, et al. Association between hospital and ICU structural factors and patient outcomes in China: a secondary analysis of the National Clinical Improvement System Data in 2019. Crit Care 2022;26:24. [Crossref] [PubMed]
- Papazian L, Klompas M, Luyt CE. Ventilator-associated pneumonia in adults: a narrative review. Intensive Care Med 2020;46:888-906. [Crossref] [PubMed]
- Kock KS, Maurici R. Respiratory mechanics, ventilator-associated pneumonia and outcomes in intensive care unit. World J Crit Care Med 2018;7:24-30. [Crossref] [PubMed]
- Martin-Loeches I, Rodriguez AH, Torres A. New guidelines for hospital-acquired pneumonia/ventilator-associated pneumonia: USA vs. Europe. Curr Opin Crit Care 2018;24:347-52. [Crossref] [PubMed]
- Kalil AC, Metersky ML, Klompas M, et al. Management of Adults With Hospital-acquired and Ventilator-associated Pneumonia: 2016 Clinical Practice Guidelines by the Infectious Diseases Society of America and the American Thoracic Society. Clin Infect Dis 2016;63:e61-e111. [Crossref] [PubMed]
- Razazi K, Arrestier R, Haudebourg AF, et al. Risks of ventilator-associated pneumonia and invasive pulmonary aspergillosis in patients with viral acute respiratory distress syndrome related or not to Coronavirus 19 disease. Crit Care 2020;24:699. [Crossref] [PubMed]
- Ścisło L, Walewska E, Bodys-Cupak I, et al. Nutritional Status Disorders and Selected Risk Factors of Ventilator-Associated Pneumonia (VAP) in Patients Treated in the Intensive Care Ward-A Retrospective Study. Int J Environ Res Public Health 2022;19:602. [Crossref] [PubMed]
- Watson K, Reoch J, Heales LJ, et al. The incidence and characteristics of ventilator-associated pneumonia in a regional nontertiary Australian intensive care unit: A retrospective clinical audit study. Aust Crit Care 2021; [Epub ahead of print]. [PubMed]
- Ribeiro ILA, Bellissimo-Rodrigues WT, Mussolin MG, et al. Impact of a dental care intervention on the hospital mortality of critically ill patients admitted to intensive care units: A quasi-experimental study. Am J Infect Control 2022; [Epub ahead of print]. [Crossref] [PubMed]
- Zhu S, Wang W, Kang Y, et al. Clinical outcomes and risk factors for mortality from ventilator-associated events: A registry-based cohort study among 30,830 intensive care unit patients. Infect Control Hosp Epidemiol 2022;43:48-55. [Crossref] [PubMed]
- Nseir S, Le Gouge A, Pouly O, et al. Relationship Between Obesity and Ventilator-Associated Pneumonia: A Post Hoc Analysis of the NUTRIREA2 Trial. Chest 2021;159:2309-17. [Crossref] [PubMed]
- Wu D, Wu C, Zhang S, et al. Risk Factors of Ventilator-Associated Pneumonia in Critically III Patients. Front Pharmacol 2019;10:482. [Crossref] [PubMed]
- Othman HA, Gamil NM, Elgazzar AEM, et al. Ventilator associated pneumonia, incidence and risk factors in emergency intensive care unit Zagazig university hospitals. Egyptian Journal of Chest Diseases and Tuberculosis 2017;66:703-8. [Crossref]
- Liu Y, Di Y, Fu S. Risk factors for ventilator-associated pneumonia among patients undergoing major oncological surgery for head and neck cancer. Front Med 2017;11:239-46. [Crossref] [PubMed]
- Jiménez-Trujillo I, Jiménez-García R, de Miguel-Díez J, et al. Incidence, characteristic and outcomes of ventilator-associated pneumonia among type 2 diabetes patients: An observational population-based study in Spain. Eur J Intern Med 2017;40:72-8. [Crossref] [PubMed]
- Larsson J, Itenov TS, Bestle MH. Risk prediction models for mortality in patients with ventilator-associated pneumonia: A systematic review and meta-analysis. J Crit Care 2017;37:112-8. [Crossref] [PubMed]
- Karakuzu Z, Iscimen R, Akalin H, et al. Prognostic Risk Factors in Ventilator-Associated Pneumonia. Med Sci Monit 2018;24:1321-8. [Crossref] [PubMed]
- Gaudet A, Devos M, Keignart S, et al. Usefulness of Sepsis-3 in diagnosing and predicting mortality of ventilator-associated lower respiratory tract infections. PLoS One 2021;16:e0245552. [Crossref] [PubMed]
- Boeck L, Eggimann P, Smyrnios N, et al. The Sequential Organ Failure Assessment score and copeptin for predicting survival in ventilator-associated pneumonia. J Crit Care 2012;27:523.e1-9. [Crossref] [PubMed]
- Srinivasan M, Shetty N, Gadekari S, et al. Comparison of the Nosocomial Pneumonia Mortality Prediction (NPMP) model with standard mortality prediction tools. J Hosp Infect 2017;96:250-5. [Crossref] [PubMed]
- Johnson AE, Pollard TJ, Shen L, et al. MIMIC-III, a freely accessible critical care database. Sci Data 2016;3:160035. [Crossref] [PubMed]
- Liu Q, Yang J, Zhang J, et al. Description of Clinical Characteristics of VAP Patients in MIMIC Database. Front Pharmacol 2019;10:62. [Crossref] [PubMed]
- Horan TC, Andrus M, Dudeck MA. CDC/NHSN surveillance definition of health care-associated infection and criteria for specific types of infections in the acute care setting. Am J Infect Control 2008;36:309-32. [Crossref] [PubMed]
- Wałaszek M, Kosiarska A, Gniadek A, et al. The risk factors for hospital-acquired pneumonia in the Intensive Care Unit. Przegl Epidemiol 2016;70:15-20, 107-10. [PubMed]
- Joseph NM, Sistla S, Dutta TK, et al. Ventilator-associated pneumonia in a tertiary care hospital in India: incidence and risk factors. J Infect Dev Ctries 2009;3:771-7. [Crossref] [PubMed]
- Apostolopoulou E, Bakakos P, Katostaras T, et al. Incidence and risk factors for ventilator-associated pneumonia in 4 multidisciplinary intensive care units in Athens, Greece. Respir Care 2003;48:681-8. [PubMed]
- But A, Yetkin MA, Kanyilmaz D, et al. Analysis of epidemiology and risk factors for mortality in ventilator-associated pneumonia attacks in intensive care unit patients. Turk J Med Sci 2017;47:812-6. [Crossref] [PubMed]
- Chang L, Dong Y, Zhou P. Investigation on Risk Factors of Ventilator-Associated Pneumonia in Acute Cerebral Hemorrhage Patients in Intensive Care Unit. Can Respir J 2017;2017:7272080. [Crossref] [PubMed]
- Čiginskienė A, Dambrauskienė A, Rello J, et al. Ventilator-Associated Pneumonia due to Drug-Resistant Acinetobacter baumannii: Risk Factors and Mortality Relation with Resistance Profiles, and Independent Predictors of In-Hospital Mortality. Medicina (Kaunas) 2019;55:49. [Crossref] [PubMed]
- Lisboa T, Diaz E, Sa-Borges M, et al. The ventilator-associated pneumonia PIRO score: a tool for predicting ICU mortality and health-care resources use in ventilator-associated pneumonia. Chest 2008;134:1208-16. [Crossref] [PubMed]
- Mirsaeidi M, Peyrani P, Ramirez JA, et al. Predicting mortality in patients with ventilator-associated pneumonia: The APACHE II score versus the new IBMP-10 score. Clin Infect Dis 2009;49:72-7. [Crossref] [PubMed]
- Gursel G, Demirtas S. Value of APACHE II, SOFA and CPIS scores in predicting prognosis in patients with ventilator-associated pneumonia. Respiration 2006;73:503-8. [Crossref] [PubMed]