Red blood cell distribution width as a marker of hyperinflammation and mortality in COVID-19
Introduction
The coronavirus disease 2019 (COVID-19) has resulted in a pandemic that will determine worldwide society probably for generations, and far from being controlled, there are still great gaps in knowledge about its physiopathology and epidemiology (1). Since the initial outbreak in December 2019, risk factors for developing acute respiratory distress syndrome (ARDS), central axis of its mortality and morbidity, have been identified. Besides age and comorbidities, parameters such as neutrophilia, lymphocytopenia and thrombopenia, data suggestive of organ injury [bilirubin, Aspartate Aminotransferase, urea, creatinine or lactate dehydrogenase (LDH)] and coagulation function abnormalities [D-dimer (DD) or prothrombin activity] have been associated with the risk of developing ARDS, intensive care unit (ICU) admission and death (2-4). Furthermore, and in relation to the “cytokine storm” that seems to determine the disease pathophysiology and ARDS, interleukin-6 (IL-6), IL-10, ferritin and C reactive protein (CRP) have been also identified as prognostic factors (5-8). In fact, the key role that IL-6 seems to play in the immunological imbalance and hyperactivation has led to consider its blockage as a specific target in treatment, with satisfying results (9-12).
Among all the processes in which IL-6 participates, including T-cell differentiation, B-cell maturation, synthesis and secretion of immunoglobulins, its main role in the pathophysiology of anemia of chronic disease is of particular interest (13,14). In addition to diminishing the erythropoietin response and reducing erythrocyte survival, IL-6 inhibits erythropoiesis and hemoglobin synthesis in the bone marrow. Moreover, it induces hepatic cells hepcidin release through binding to the STAT3 receptor. Hepcidin is a protein that controls iron metabolism since it regulates the expression of divalent metal transporter (DMT-1) and enhances the breakdown of ferroportin, which leads to blockade of the duodenal iron transfer. As a result, hepcidin blocks intestinal iron absorption and iron recycling by macrophages. In the same way, IL-6 induces the transcription of ferritin, that also leads to increased iron retention and overload within reticulo-endothelial cells (15,16). Accordingly, the elevated ferritin, despite iron overload and increased iron storage, reflects iron dysregulation and the inhibition of erythropoiesis.
Red blood cell distribution width (RDW) is a parameter routinely reported as part of a complete blood count, and measures the size variability of circulating erythrocytes. RDW is calculated as standard deviation of red blood cell volume divided by mean corpuscular volume, expressed as a percentage. Traditionally, it has been considered for the differential diagnosis of iron deficiency anemia and anemia of chronic disease (17). Recently, multiple studies demonstrating that RDW values correlate with inflammation in clinical scenarios as diverse as cardiovascular disease (18), rheumatoid arthritis (19) and systemic lupus erythematosus (20) have been published. Furthermore, RDW has been studied as a mortality risk factor in sepsis and ARDS (21-24). In the COVID-19 setting, other reports have analyzed this high availability parameter as a prognostic marker of severity and death in hospitalized patients with COVID-19 (25,26).
Therefore, and based in the aforementioned pathophysiological considerations, since RDW reflects anisocytosis probably determined by the hyperinflammatory background and the effects of cytokines such as IL-6, we performed the present study with the objective to analyze its role and performance to predict outcome in COVID-19 ARDS. Moreover, we evaluated the relationship between the parameters that have shown a prognostic value and the chronology of their variation during the first days of the cytokine storm, both in patients treated and not treated with tocilizumab. We present the following article in accordance with the STROBE reporting checklist (available at https://apm.amegroups.com/article/view/10.21037/apm-22-119/rc).
Methods
Study design and participants
The study population consisted of the subset of the patients from the TOCICOV Study (described elsewhere) (9) that were included at Hospital Puerta de Hierro-Majadahonda. This was a retrospective observational study designed to compare mortality and ICU admission between patients treated with corticoids and tocilizumab versus controls, during the first wave of the pandemic. The study was approved by the Research Ethics Committee of Hospital Puerta de Hierro Majadahonda (No. FIB-TOC-2020-01) and a waiver for informed consent was granted. The study conformed to the provisions of the Declaration of Helsinki (as revised in 2013).
Inclusion criteria for the TOCICOV study were as follow: adult patients (≥18 years) with COVID-19, confirmed by polymerase chain reaction (PCR) on nasopharyngeal swab, who were consecutively admitted to our hospital between March 3th and April 20th, hospitalized outside the ICU and presented documented interstitial pneumonia with severe respiratory failure (respiratory severity scale BRESCIA-COVID =2) (27) or rapid deterioration of respiratory exchanges without immediate possibility of invasive ventilation (respiratory severity scale COVID =3) and at least one of the following parameters: IL-6 >40 pg/mL, increasing LDH or LDH > twice upper normal limit, increasing CRP, DD >1,500 ng/mL, lymphocytes <1,200/µL or ferritin >500 ng/mL. Patients who died within 24 hours after admission to the hospital or after developing inclusion criteria were excluded.
Data collection
Clinical, pharmacological, laboratory and radiologic data was extracted from medical records using a standardized data collection form. Patients follow-up, corticosteroid use, blood examinations and serum biochemical tests were done according to clinical practice and each physicians criteria. Data was collected from the first day at hospital admission, at the inclusion in the TOCICOV study according to the criteria specified above (day 0) and days 1, 3, 7 and 15 post-inclusion. All data was included by a primary reviewer and subsequently checked by three senior physicians. Routine blood examinations included a complete blood count, coagulation tests including DD, serum biochemical tests including lactate dehydrogenase, CRP, serum ferritin and IL-6. Chest radiographs or computed tomography scans were also done for all inpatients.
Biomarkers analysis and dynamics
We analyzed the association of RDW and IL-6 with in-hospital mortality during the first 7 days of the study period, the main study outcome. Besides, we considered other blood count parameters, coagulation tests and biomarkers including LDH, CRP and ferritin. In order to measure the parameters accuracy, we determined the area under the curve (AUC)-“receiver operating characteristic”-(ROC). Secondly, we analyzed the influence of tocilizumab treatment on the values of the mentioned parameters, and performed a multivariable analysis considering tocilizumab treatment and hemoglobin values to confirm the independent prognostic ability of the inflammatory parameters. Thirdly, we studied the correlations among the different biomarkers to better understand their role and dynamics as well as the pathophysiology of the cytokine storm. Parameters were considered at admission, at inclusion (day 0) and during the first week of inclusion (days 1, 3 and 7).
Statistical analysis
Data were expressed as mean and standard deviation or number (percentage) as appropriate. The Kolmogorov test was used to evaluate data distribution and as data did not follow a parametric distribution, statistical analysis was performed using Spearman rank’s test to analyze correlations and Mann-Whitney U-test to assess differences between groups. Levene’s test was used for the homogeneity of variance test. The χ2 test (with the two-sided Fisher’s exact test) was used to compare categorical variables. The discrimination ability was evaluated following an approach based on the AUC-ROC. Finally, we performed a multivariable analysis considering tocilizumab treatment and hemoglobin for the parameters associated with mortality in the univariate analysis since IL-6 values can rise after IL-6 blockade and because anemia status could influence RDW values (17). For all analyses, significance was defined as a P value below 0.05. Statistical analysis was performed using SPSS software version 26.0 (IBM Corp., Armonk, NY, USA).
Results
Clinical characteristics
Overall, 173 patients with COVID-19 and respiratory insufficiency or increased inflammatory parameters were included. Their baseline characteristics and comorbidities, in addition to the treatment received according to outcome (survivors versus non-survivors) are shown in Table 1. Mean age was 66.6 years and 67.1% patients were male. Mean time from symptom onset to patient inclusion was 10 days, and mean time from hospital admission to inclusion was 2.8 days. At the time of inclusion, 66.7% were receiving oxygen therapy through nasal cannula or Venturi mask and 31.4% through oxygen mask with reservoir bag; mean oxygen saturation by pulse oximetry (SapO2)/fraction of inspired oxygen (FiO2) ratio (SaO2/FiO2) was 202. The 90.2% of patients received steroids and 57.4% tocilizumab. Among patients treated with tocilizumab, 87.2% received it at the day of inclusion (day 0). Infectious complications were identified and confirmed in 15 patients. Global mortality was 20.8%.
Table 1
Characteristics | Global (n=173) | Survivors (n=137) | Non-survivors (n=36) | P value |
---|---|---|---|---|
Age, mean ± SD | 66.6±13.6 | 63.6±12.5 | 78±11.5 | <0.001 |
Gender (men), n (%) | 116 (67.1) | 91 (66.4) | 25 (69.4) | 0.843 |
Charlson score, mean ± SD | 3.49±2.5 | 2.9±2.1 | 5.4±2.8 | <0.001 |
Underlying medical conditions, n (%) | ||||
Arterial hypertension | 94 (54.3) | 56 (40.9) | 23 (63.9) | 0.015 |
Diabetes | 49 (28.3) | 31 (22.6) | 18 (50.0) | 0.003 |
Cardiovascular disease | 44 (25.4) | 25 (18.2) | 19 (52.8) | <0.001 |
Chronic lung disease | 31 (17.9) | 20 (14.6) | 11 (30.6) | 0.048 |
Neurological disease | 21 (12.1) | 10 (7.3) | 11 (30.6) | 0.001 |
Liver disease | 13 (7.5) | 5 (3.6) | 8 (22.2) | 0.001 |
Chronic kidney disease | 15 (8.7) | 6 (4.4) | 9 (25.0) | <0.001 |
Onco-hematologic | 8 (4.6) | 5 (3.6) | 3 (8.3) | 0.365 |
HIV | 3 (1.7) | 3 (2.2) | 0 | 1 |
Transplant (SOT/SCT) | 3 (1.7) | 1 (0.7) | 2 (5.6) | 0.110 |
Clinical characteristics, mean ± SD | ||||
SBP | 117±21 | 115±19 | 138±37 | 0.038 |
DBP | 68±11 | 68±11 | 66±13 | 0.654 |
Temperature | 37±1 | 37±1 | 37±1 | 0.111 |
Respiratory rate | 26±7 | 25±7 | 28±1 | 0.112 |
SapO2 | 93±4 | 93±3 | 92±6 | 0.097 |
FiO2 | 53±28 | 53±28 | 55±27 | 0.769 |
SapO2/FiO2 | 202±84 | 206±85 | 188±78 | 0.266 |
Treatment received, n (%) | ||||
Lopinavir/ritonavir | 143 (82.7) | 120 (83.9) | 23 (63.9) | 0.002 |
Hydroxychloroquine | 170 (98.3) | 135 (79.4) | 35 (93.2) | 0.506 |
Interferon | 102 (59.0) | 92 (67.2) | 10 (72.2) | 0.000 |
Azithromycin | 75 (43.4) | 54 (39.4) | 21 (58.3) | 0.058 |
Ceftriaxone | 84 (48.6) | 68 (49.6) | 16 (44.4) | 0.708 |
Levofloxacin | 24 (13.9) | 23 (16.8) | 1 (2.8) | 0.030 |
Steroids | 156 (90.2) | 124 (90.5) | 32 (88.9) | 0.757 |
Tocilizumab | 108 (62.4) | 93 (67.9) | 15 (41.7) | 0.006 |
Outcomes, n (%) | ||||
Infectious complications (confirmed) | 15 (8.7) | 12 (8.8) | 3 (8.3) | 1 |
SD, standard deviation; HIV, human immunodeficiency virus; SOT, solid organ transplantation; SCT, stem cell transplantation; SBP, systolic blood pressure; DBP, diastolic blood pressure; SapO2, saturation by pulse oximetry; FiO2, fraction of inspired oxygen; SapO2/FiO2, saturation by pulse oximetry/fraction of inspired oxygen ratio.
Comparison of biomarkers in survivors versus non-survivors
Blood count and biochemical parameters, in addition to coagulation tests at admission, at the moment of inclusion (day 0) and during admission (days 1, 3 and 7) were analyzed and compared according to death. RDW and IL-6 dynamics are shown in Figures 1,2, respectively, while the other parameters are illustrated in the Figures S1,S2.
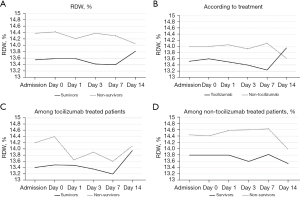
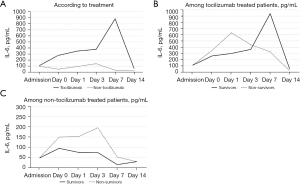
At day 0, the patients who ultimately died presented a higher RDW (13.60% vs. 14.42%, P=0.026). No significant differences were found when other parameters were analyzed at day 0 (Table 2). During overall admission, RDW at hospital admission (13.53% vs. 14.35%, P=0.0016), day 3 (13.43% vs. 14.36%, P<0.001) and day 7 (13.41% vs. 14.31%, P=0.046) was higher in non-survivors (Figure 1A). Otherwise, non-survivors presented higher admission CRP (105 vs. 136 mg/L, P=0.0021), day 1 CRP (128 vs. 176 mg/L, P=0.003), day 7 CRP (23 vs. 55 mg/L, P=0.01), day 1 IL-6 (243 vs. 454 pg/mL, P=0.046) and day 3 ferritin (1,444 vs. 1,934 ng/mL, P=0.029). Neither lymphocyte counts, neutrophil counts, NLR, platelet counts, hemoglobin, PA, fibrinogen, DD or LDH showed significant differences among survivors and non-survivors (Figures S1,S2).
Table 2
Laboratory values | Overall (n=173), mean ± SD | Survivors (n=137), mean ± SD | Non survivors (n=36), mean ± SD | P value |
---|---|---|---|---|
Lymphocytes/μL | 869±473 | 868±488 | 869±421 | 0.990 |
Neutrophils/μL | 6,381±3,588 | 6,477±3,520 | 6,028±3,864 | 0.520 |
N/L ratio | 9.43±7.95 | 9.55±7.80 | 8.98±8.59 | 0.715 |
Platelets (103/μL) | 228±85 | 235±85 | 204±82 | 0.06 |
Hb (g/dL) | 13.58±1.96 | 13.55±1.99 | 13.72±1.84 | 0.308 |
RDW (%) | 13.76±1.84 | 13.60±1.81 | 14.42±1.87 | 0.026 |
Prothrombin activity (%) | 87.01±16.50 | 86.74±18.20 | 86.53±13.40 | 0.954 |
Fibrinogen (mg/dL) | 676±143 | 682±148 | 653±113 | 0.399 |
DD (ng/mL) | 1,395±1,700 | 1,330±1,658 | 1,646±1,858 | 0.336 |
CRP (mg/L) | 144±82 | 142±86 | 154±67 | 0.462 |
AST (U/L) | 71±112 | 69±106 | 79±134 | 0.659 |
LDH (UI/L) | 404±174 | 396±178 | 437±155 | 0.213 |
IL-6 (pg/mL) | 199±228 | 186±221 | 293±274 | 0.286 |
Ferritin (ng/mL) | 1,478±1,638 | 1,394±834 | 1,933±3,793 | 0.344 |
SD, standard deviation; N/L, neutrophils to lymphocytes ratio; Hb, hemoglobin; RDW, red blood cell distribution width; DD, D-dimer; CRP, C reactive protein; AST, aspartate aminotransferase; LDH, lactate dehydrogenase; IL-6, interleukin-6.
In order to compare the performance of the different biomarkers associated with mortality, an ROC-AUC analysis was performed (Figures 3,4, Table 3). Admission, days 0, 3 and 7 RDW values showed AUC beyond 0.650, being day 3 RDW the best predictor with AUC 0.695. Both day 1 CRP and day 1 IL-6 also showed AUC under 0.650.
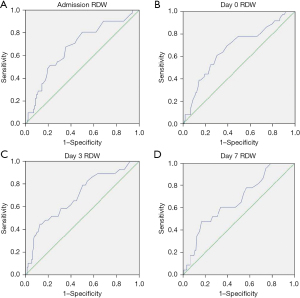
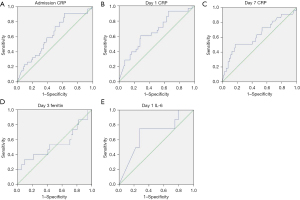
Table 3
Parameter | AUC-ROC | 95% confidence interval |
---|---|---|
Admission RDW | 0.668 | 0.567–0.769 |
Day 0 RDW | 0.680 | 0.574–0.785 |
Day 3 RDW | 0.695 | 0.587–0.803 |
Day 7 RDW | 0.666 | 0.548–0.783 |
Admission CRP | 0.625 | 0.523–0.727 |
Day 1 CRP | 0.662 | 0.549–0.775 |
Day 7 CRP | 0.633 | 0.494–0.773 |
Day 1 IL-6 | 0.681 | 0.471–0.892 |
Day 3 ferritin | 0.540 | 0.348–0.731 |
AUC-ROC, area under the curve receiving operating characteristic; RDW, red blood cell distribution width; CRP, C reactive protein; IL-6, interleukin-6.
Variation of biomarkers according to tocilizumab treatment.
The mentioned parameters were analyzed according to tocilizumab treatment to understand the influence of IL-6 blockade on them (Figures S3,S4). Patients who received tocilizumab presented a higher day 0 CRP (127 vs. 155 mg/L, P=0.038) but a lower day 3 CRP (92 vs. 43 mg/L, P<0.001) and day 7 CRP (55 vs. 12.5 mg/L, P<0.001). Furthermore, IL-6 levels were higher in the patients who received tocilizumab at day 0 (98 vs. 270 pg/mL, P=0.008), day 1 (95 vs. 346 pg/mL, P=0.004) and day 3 (141 vs. 379 pg/mL, P=0.016) (Figure 2A). There were no differences in RDW, ferritin, lymphocyte counts, neutrophil counts, NLR, platelet counts, hemoglobin, PA, fibrinogen, DD or LDH according to tocilizumab treatment (Figures S3,S4).
In addition, we performed different multivariable analyses according to tocilizumab treatment and hemoglobin levels to assess the independent prognostic role of the parameters associated with mortality in the univariate analysis. Admission RDW (OR =1.23, 95% CI: 1.01–1.49, P=0.041), day 0 RDW (OR =1.22, 95% CI: 1–1.49, P=0.05), day 3 RDW (OR =1.25, 95% CI: 1.01–1.56, P=0.047), day 0 CRP (OR =1.01, 95% CI: 1–1.01, P=0.043) and day 1 CRP (OR =1.01, 95% CI: 1.01–1.02, P=0.001) were independently prognostic of mortality, while day 7 CRP, day 1 IL-6 and day 3 ferritin were not.
Correlation between the inflammatory parameters
The correlation between the inflammatory parameters was analyzed to understand the physiopathology of the cytokine storm (Table S1). IL-6 at admission showed significant correlation with day 0 CRP (r=0.332, P=0.007) and day 1 CRP (r=0.303, P=0.015) while IL-6 at day 0 was correlated with day 0 CRP (r=0.297, P=0.003) and day 0 ferritin (r=0.539, P=0.008). In parallel, day 1 CRP was correlated with admission RDW (r=0.186, P=0.026), day 0 RDW (r=0.275, P=0.002), day 1 RDW (r=0.255, P=0.04) and day 3 RDW (r=0.277, P=0.001). Similarly, day 3 CRP was correlated with day 0 RDW (r=0.188, P=0.03), day 1 RDW (r=0.245, P=0.001) and day 3 RDW (r=0.266, P=0.001). Day 7 CRP showed correlation with day 7 RDW (r=0.218, P=0.017).
Despite the afore-mentioned data, no correlation was found between IL-6 and RDW in the overall cohort. However, in the patients not treated with tocilizumab, IL-6 levels at day 0 were strongly correlated with day 3 RDW (r=0.733, P=0.004) and with day 3 CRP (r=0.727, P=0.022) (Figure 5A,5B). In these patients, day 3 RDW and day 3 CRP also showed a significant correlation (r=0.358, P=0.005) (Figure 5C).
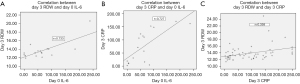
Discussion
Our results indicate that RDW may predict COVID-19-associated ARDS mortality with better discrimination ability than other parameters, reflecting the hyperinflammatory background and the effects of cytokines such as IL-6, irrespective of tocilizumab treatment.
RDW is a parameter routinely reported as part of a complete blood count, measuring the size variability of circulating erythrocytes (anisocytosis) (17). During the recent years, several studies have confirmed that RDW adequately assesses inflammation in diverse clinical scenarios (17-24). In this context, it has been hypothesized that anisocytosis reflects the dysregulation of the iron metabolism and the inhibition of erythropoiesis that result in the anemia of the chronic disease, which in turn is attributable to the effect of diverse cytokines, mainly IL-6 (13-16). Interestingly, other authors have found that inflammatory anemia-associated parameters changed significantly in parallel to RDW in patients with sepsis or heart failure, due to the effect of cytokines on erythropoiesis (16,21-23,28,29). Since IL-6 is one of the key molecules of the COVID-19 disease pathophysiology and because tocilizumab has demonstrated to improve survival in this setting, the aim of our study was to analyze the RDW prognostic role and to understand the effects of IL-6 blockade on this parameter.
To date, several publications support that the RDW is higher in more severe COVID-19 patients and those with worse outcomes (25,26,30-35). In fact, Hornick et al confirmed that RDW was associated with mortality after adjusting for age, sex, race, cardiovascular disease or hemoglobin (36). In addition, this interesting report identified that RDW was associated with certain pro-inflammatory cytokines such as TNF-α or IL-6. Similarly, Martínez-Urbistondo et al. noticed higher RDW values, among other inflammatory markers, in the patients with higher IL-6 levels (37). However, there are still important unanswered questions about the mechanisms or the usefulness of this parameter. We believe our study helps to clarify the previous findings but additionally presents some strengths and novelties that, to our knowledge, have not been previously mentioned by others. On the one hand, we evaluated not only RDW but other additional biomarkers such as ferritin, CRP, IL-6 and LDH, among others, both during the course of the disease and after tocilizumab treatment. Accordingly, we studied their role as biomarkers, their dynamics and relations, providing a more comprehensive picture of the inflammatory environment during the cytokine storm. In addition, we considered the effect of tocilizumab treatment and its potential role as a confounding factor of the prognostic ability of the mentioned parameters. This point is particularly important in the case of IL-6, which is directly altered by the use of tocilizumab. Finally, we would like to highlight that we evaluated all these biomarkers in a homogeneous population with severe disease, with less selection bias when compared to other cohorts including a wide array of patients with different degrees of clinical severity, from outpatients to patients admitted to ICU. Besides, the analysis in this homogenous cohort, while limiting the possibility to identify or compare to other prognostic markers, would yield more strong and robust results once the differences and statistical significances would be found.
In our cohort, we found that the prognostic value of RDW was dynamic, useful both from hospital admission to seven days after the respiratory worsening, irrespective of hemoglobin values and tocilizumab treatment. In addition, RDW showed better discrimination ability for mortality than other inflammatory parameters such as lymphocyte count, DD, LDH or ferritin. Besides, some authors have described that RDW constantly increases from symptom onset in COVID-19 disease while Kim et al. and Ku et al. identified a higher mortality risk in the patients whose RDW increased within 72 hours after the admission because of sepsis or after the onset of gram-negative bacteremia, respectively (22,38-40). Similarly, in our cohort, day 3 RDW after respiratory worsening was the best mortality predictor, beyond CRP, ferritin or IL-6. As a matter of fact, day 3 RDW was strongly correlated with IL-6 values at inclusion but only in the patients not treated with tocilizumab, suggesting that the IL-6 blockade prevented the IL-6 inflammatory effects and dysregulation of the red blood cells synthesis and therefore there were no changes on day 3 RDW values after tocilizumab treatment. In the same way, CRP, a clearly identified marker and prognostic in COVID-19 disease, was the only other parameter independently associated with mortality. At the same time, day 3 CRP was also strongly determined by IL-6 at inclusion and was correlated with RDW during the study period. Therefore, we believe that RDW, and mainly day 3 RDW after the respiratory worsening, reflects the hyperinflammatory state and the cytokine storm that leads to COVID-19 severe disease, in parallel to CRP, being a surrogate marker of the pleiotropic effects of IL-6. In addition, these findings suggest that RDW could be useful to monitor the inflammatory environment and the effect of the active IL-6, even when IL-6 levels are increased as a result of the detection of blocked IL-6 after tocilizumab administration, and no longer reflect inflammation.
However, there are recent reports that have called into question the lead role of IL-6 in COVID-19.
In the interesting report from Leisman et al, the elevations of IL-6 were remarkably lower than those reported in patients with ARDS unrelated to COVID-19, sepsis, and the chimeric antigen receptor (CAR) T cell-induced cytokine release syndrome (CRS) (41). However, several biomarkers, including DD, CRP, ferritin or LDH were elevated to a similar or greater extent in patients with COVID-19 than in patients with the compared disorders. These findings are in the same direction of the report from Zizzo et al., who alternatively proposes IL-33 as the key player in driving all stages of COVID-19 (42). From this perspective, IL-6 as well as other cytokines such as IL-1b, IL-7 or IL-2, would be mediators in the damage produced by IL-33. We consider that our results do not contradict these hypotheses but rather reinforce the concept that COVID-19 pathophysiology differs from the classical hyperinflammatory states as sepsis, CRS, macrophage activation syndrome (MAS) or hemophagocytic lymphohistiocytosis (43). Consequently, and despite the previous reports, IL-6 in our cohort was not an independent prognostic factor of mortality. On the one hand, this result confirms that IL-6 levels after tocilizumab treatment do not reflect inflammation or activity once the receptor is blocked. On the other hand, reinforces the hypothesis that IL-6 is probably not the only and initial factor but could be a key mediator that, along with other cytokines, determines the cytokine storm. As a result, these findings provide more consistency to the utility and applicability of RDW as a surrogate marker of the cytokine storm and not only of the IL-6 effects. All the same, further studies are needed to characterize and determine COVID-19 complex pathophysiology that not necessarily has to be parallel to the hyperinflammatory syndromes aforesaid.
Nevertheless, we have to consider some limitations. Our study was a single-center, observational, retrospective study, where the population size was relatively small. Therefore, the present data need confirmation in other populations. Second, the homogeneity of the population, since all patients presented severe disease, can limit the generalizability of the study to mild or non-severe COVID-19. Another pitfall of the study is the fact that over 90% of the patients in our cohort received steroids. Therefore, our data represent mainly what occurs under steroid treatment, but, given the present evidence and recommendations (44,45), that will probably be the most frequent scenario in patients with severe COVID-19 ARDS and is not necessarily a confounding factor.
In summary, RDW may predict mortality in severe COVID-19 pneumonia and reflects the hyperinflammatory background and the effect of cytokines such as IL-6. This readily available parameter could help clinicians to identify patients at risk, those who are potential candidates to IL-6 blockade and to monitor treatment response to tocilizumab, discerning ongoing inflammation among patients who present evenly elevated IL-6.
Acknowledgments
We thank the members of the COVID-19 working group for their contribution: Múñez Rubio, Elena; Malo de Molina Ruiz, Rosa; Pintos Pascual, Ilduara; Callejas Díaz, Alejandro; Díaz De Santiago, Alberto; Ramos Martínez, Antonio; De La Fuente Moral, Sara; Laguna Del Estal, Pedro; Vázquez Contreras, Gema; Valle Falcones, Manuel; Muñoz Serrano, Alejandro; Cantos Sánchez De Ibarguen, Blanca; Calderón Parra, Jorge; Ángel-Moreno Maroto, Alfonso; Baños Pérez, Isolina; Máinez Sáiz, Mª Carmen; Montero Hernández, Esther; Carreño Hernández, Mª Cruz; Romero Pizarro, Yolanda; Muñoz De Benito, Rosa; Durán Del Campo, Pedro; Mellor Pita, Susana; Tutor De Ureta, Pablo; Aguilar Pérez, Miriam; Díaz Nuevo, Guadalupe Gema; García Fadul, Christian; Jara Chinarro, Beatriz; Laporta Hernández, Rosalía; Lázaro Carrasco de la Fuente, María Teresa; López García Gallo, Cristina María; Mínguez Clemente, Patricia; Trisán Alonso, Andrea; Carabias Arca, Roberto; Erro Iribarren, Marta; Agudo Castillo, Belén; Aller Pardo, Javier; Benlloch Rodríguez, Raquel; Blasco Quílez, Mª Rosario; Brito Sanfiel, Miguel Ángel; Calvo De Juan, Virginia; Calvo Moya, Marta; Campos Esteban, José; Cazorla Calleja, Rosario; Cea Soriano, Matías, Cembrero Saralegui, Hirune; Colino Alcol, Esmeralda; Córdoba Largo, Sofía; Cruz Melguizo, Sara; Del Pozo Jiménez, Gema; Del Pozo Martín, Cristina; Elosua González, Marta; Espinosa Malpartida, María; Fernández Manzano, Cristóbal; Ferre Aracil, Carlos; García-Espantaleón Navas, Manuel; García-Izquierdo Jaén, Eusebio Alejandro; Gil Haro, Beatriz; Gómez-Porro Sánchez, Pablo; González López, Sofía; González Partida, Irene; Hernández López, Roberto; Hernández Pérez, Francisco José; Huerta Arroyo, Ana Mª; Iglesias Escalera, Gema; López Llorca, Ana Isabel; Losa Maroto, Azucena; Marín Martínez, Mª Eugenia; Martínez Badas, Itziar; Martínez Muñoz, Mª Esther; Maximiano Alonso, Constanza; Méndez García, Miriam; Mingo Santos, Susana; Mitroi, Cristina; Núñez García, Beatriz; Ortega García, Paula; Oteo Domínguez, Juan Francisco; Pérez Fernández, Natalia; Prieto Coca, Lucía; Relea Pérez, Lucía; Rodríguez Reina, Gabriel; Sabín Muñoz, Julia; Sáenz Medina, Javier; Sánchez Aparicio, Alejandra; Sánchez Ruiz, Antonio; Sanz Sanz, Jesús; Segovia Cubero, Javier; Silva Hernández, Lorenzo; Toquero Ramos, Jorge; Velasco Martínez, Mª Eugenia; Villaverde González, Serena; Andrés Eisenhofer, Ane; Blanco Alonso, Silvia; Diego Yagüe, Itziar; Donate Velasco, Ignacio; Escudero López, Gabriela; Expósito Palomo, Esther; Galán Gómez, Amy; García Prieto, Sonia; Gómez Irusta, Javier; Gutiérrez Abreu, Edith Vanessa; Gutiérrez Martín, Isabel; Gutiérrez Rojas, Ángela; Gutiérrez Villanueva, Andrea; Herráiz Jiménez, Jesús; Martínez Urbistondo, María; Martínez Vera, Fernando; Mora Vargas, Alberto; Morrás De La Torre, Ignacio; Muñoz Gómez, Ana; Valencia Alijo, Ángela; Vázquez Comendador, J. Manuel; Arias Milla, Ana; Bilbao Garay, Javier; Duca, Ana María; García Viejo, Miguel Ángel; Palau Fayós, José Mª; Roldán Montaud, Alberto; Castejón Díaz, Raquel; Citores Sánchez, María Jesús; Rosado García, Silvia; de Mendoza Fernández, Carmen; Vargas Núñez, Juan Antonio; Ussetti Gil, Piedad; Cuervas-Mons Martínez, Valentín.
Funding: This work was supported by a grant from Instituto de Salud Carlos III (Expedient number CM19/00223).
Footnote
Reporting Checklist: The authors have completed the STROBE reporting checklist. Available at https://apm.amegroups.com/article/view/10.21037/apm-22-119/rc
Data Sharing Statement: Available at https://apm.amegroups.com/article/view/10.21037/apm-22-119/dss
Conflicts of Interest: All authors have completed the ICMJE uniform disclosure form (available at https://apm.amegroups.com/article/view/10.21037/apm-22-119/coif). The authors have no conflicts of interest to declare.
Ethical Statement: The authors are accountable for all aspects of the work in ensuring that questions related to the accuracy or integrity of any part of the work are appropriately investigated and resolved. The study was approved by the Research Ethics Committee of Hospital Puerta de Hierro Majadahonda (No. FIB-TOC-2020-01) and a waiver for informed consent was granted. The study conformed to the provisions of the Declaration of Helsinki (as revised in 2013).
Open Access Statement: This is an Open Access article distributed in accordance with the Creative Commons Attribution-NonCommercial-NoDerivs 4.0 International License (CC BY-NC-ND 4.0), which permits the non-commercial replication and distribution of the article with the strict proviso that no changes or edits are made and the original work is properly cited (including links to both the formal publication through the relevant DOI and the license). See: https://creativecommons.org/licenses/by-nc-nd/4.0/.
References
- Zhou F, Yu T, Du R, et al. Clinical course and risk factors for mortality of adult inpatients with COVID-19 in Wuhan, China: a retrospective cohort study. Lancet 2020;395:1054-62. [Crossref] [PubMed]
- Wu C, Chen X, Cai Y, et al. Risk Factors Associated With Acute Respiratory Distress Syndrome and Death in Patients With Coronavirus Disease 2019 Pneumonia in Wuhan, China. JAMA Intern Med 2020;180:934-43. [Crossref] [PubMed]
- Hu B, Guo H, Zhou P, et al. Characteristics of SARS-CoV-2 and COVID-19. Nat Rev Microbiol 2021;19:141-54. [Crossref] [PubMed]
- Moreno-Torres V, Muñoz-Serrano A, Calderón-Parra J, et al. Mortality by COVID-19 Before Vaccination - One Year Experience of Hospitalized Patients in Madrid. Int J Infect Dis 2022;116:339-43. [Crossref] [PubMed]
- Moreno-Torres V, de la Fuente S, Mills P, et al. Major determinants of death in patients hospitalized with COVID-19 during the first epidemic wave in Madrid, Spain. Medicine (Baltimore) 2021;100:e25634. [Crossref] [PubMed]
- McGonagle D, Sharif K, O'Regan A, et al. The Role of Cytokines including Interleukin-6 in COVID-19 induced Pneumonia and Macrophage Activation Syndrome-Like Disease. Autoimmun Rev 2020;19:102537. [Crossref] [PubMed]
- Yuan X, Huang W, Ye B, et al. Changes of hematological and immunological parameters in COVID-19 patients. Int J Hematol 2020;112:553-9. [Crossref] [PubMed]
- Chen G, Wu D, Guo W, et al. Clinical and immunological features of severe and moderate coronavirus disease 2019. J Clin Invest 2020;130:2620-9. [Crossref] [PubMed]
- Ruiz-Antorán B, Sancho-López A, Torres F, et al. Combination of Tocilizumab and Steroids to Improve Mortality in Patients with Severe COVID-19 Infection: A Spanish, Multicenter, Cohort Study. Infect Dis Ther 2021;10:347-62. [Crossref] [PubMed]
- RECOVERY Collaborative Group. Tocilizumab in patients admitted to hospital with COVID-19 (RECOVERY): a randomised, controlled, open-label, platform trial. Lancet 2021;397:1637-45. [Crossref] [PubMed]
- Stone JH, Frigault MJ, Serling-Boyd NJ, et al. Efficacy of Tocilizumab in Patients Hospitalized with Covid-19. N Engl J Med 2020;383:2333-44. [Crossref] [PubMed]
- Veiga VC, Prats JAGG, Farias DLC, et al. Effect of tocilizumab on clinical outcomes at 15 days in patients with severe or critical coronavirus disease 2019: randomised controlled trial. BMJ 2021;372: [Crossref] [PubMed]
- Weiss G, Goodnough LT. Anemia of chronic disease. N Engl J Med 2005;352:1011-23. [Crossref] [PubMed]
- Weiss G, Ganz T, Goodnough LT. Anemia of inflammation. Blood 2019;133:40-50. [Crossref] [PubMed]
- Knovich MA, Storey JA, Coffman LG, et al. Ferritin for the clinician. Blood Rev 2009;23:95-104. [Crossref] [PubMed]
- Wang W, Knovich MA, Coffman LG, et al. Serum ferritin: Past, present and future. Biochim Biophys Acta 2010;1800:760-9. [Crossref] [PubMed]
- Salvagno GL, Sanchis-Gomar F, Picanza A, et al. Red blood cell distribution width: A simple parameter with multiple clinical applications. Crit Rev Clin Lab Sci 2015;52:86-105. [Crossref] [PubMed]
- Danese E, Lippi G, Montagnana M. Red blood cell distribution width and cardiovascular diseases. J Thorac Dis 2015;7:E402-11. [PubMed]
- He Y, Liu C, Zeng Z, et al. Red blood cell distribution width: a potential laboratory parameter for monitoring inflammation in rheumatoid arthritis. Clin Rheumatol 2018;37:161-7. [Crossref] [PubMed]
- Zou XL, Lin XJ, Ni X, et al. Baseline Red Blood Cell Distribution Width Correlates with Disease Activity and Therapeutic Outcomes in Patients with Systemic Lupus Erythematosus, Irrespective of Anemia Status. Clin Lab 2016;62:1841-50. [Crossref] [PubMed]
- Sadaka F, O'Brien J, Prakash S. Red cell distribution width and outcome in patients with septic shock. J Intensive Care Med 2013;28:307-13. [Crossref] [PubMed]
- Kim CH, Park JT, Kim EJ, et al. An increase in red blood cell distribution width from baseline predicts mortality in patients with severe sepsis or septic shock. Crit Care 2013;17:R282. [Crossref] [PubMed]
- Jiang Y, Jiang FQ, Kong F, et al. Inflammatory anemia-associated parameters are related to 28-day mortality in patients with sepsis admitted to the ICU: a preliminary observational study. Ann Intensive Care 2019;9:67. [Crossref] [PubMed]
- Wang B, Gong Y, Ying B, et al. Relation between Red Cell Distribution Width and Mortality in Critically Ill Patients with Acute Respiratory Distress Syndrome. Biomed Res Int 2019;2019:1942078. [Crossref] [PubMed]
- Henry BM, Benoit JL, Benoit S, et al. Red Blood Cell Distribution Width (RDW) Predicts COVID-19 Severity: A Prospective, Observational Study from the Cincinnati SARS-CoV-2 Emergency Department Cohort. Diagnostics (Basel) 2020;10:618. [Crossref] [PubMed]
- Lippi G, Henry BM, Sanchis-Gomar F. Red Blood Cell Distribution Is a Significant Predictor of Severe Illness in Coronavirus Disease 2019. Acta Haematol 2021;144:360-4. [Crossref] [PubMed]
- Foca E. Linee guida sulla gestione terapeutica e di supporto per pazienti con infezione da coronavirus COVID-19. 2020; Marzo. Available online: http://www.fvcalabria.unicz.it/COVID-19/LINEE-GUIDA/linee-guida-SIMIT-marzo-2020.pdf. Accessed 1 Oct 2020.
- Allen LA, Felker GM, Mehra MR, et al. Validation and potential mechanisms of red cell distribution width as a prognostic marker in heart failure. J Card Fail 2010;16:230-8. [Crossref] [PubMed]
- Miyamoto K, Inai K, Takeuchi D, et al. Relationships among red cell distribution width, anemia, and interleukin-6 in adult congenital heart disease. Circ J 2015;79:1100-6. [Crossref] [PubMed]
- Lee JJ, Montazerin SM, Jamil A, et al. Association between red blood cell distribution width and mortality and severity among patients with COVID-19: A systematic review and meta-analysis. J Med Virol 2021;93:2513-22. [Crossref] [PubMed]
- Wang C, Zhang H, Cao X, et al. Red cell distribution width (RDW): a prognostic indicator of severe COVID-19. Ann Transl Med 2020;8:1230. [Crossref] [PubMed]
- Lorente L, Martín MM, Argueso M, et al. Association between red blood cell distribution width and mortality of COVID-19 patients. Anaesth Crit Care Pain Med 2021;40:100777. [Crossref] [PubMed]
- Taneri PE, Gómez-Ochoa SA, Llanaj E, et al. Anemia and iron metabolism in COVID-19: a systematic review and meta-analysis. Eur J Epidemiol 2020;35:763-73. [Crossref] [PubMed]
- Zinellu A, Mangoni AA. Red Blood Cell Distribution Width, Disease Severity, and Mortality in Hospitalized Patients with SARS-CoV-2 Infection: A Systematic Review and Meta-Analysis. J Clin Med 2021;10:286. [Crossref] [PubMed]
- Yağcı S, Serin E, Acicbe Ö, et al. The relationship between serum erythropoietin, hepcidin, and haptoglobin levels with disease severity and other biochemical values in patients with COVID-19. Int J Lab Hematol 2021;43:142-51. [Crossref] [PubMed]
- Hornick A, Tashtish N, Osnard M, et al. Anisocytosis is Associated With Short-Term Mortality in COVID-19 and May Reflect Proinflammatory Signature in Uninfected Ambulatory Adults. Pathog Immun 2020;5:312-26. [Crossref] [PubMed]
- Martinez-Urbistondo M, Mora-Vargas A, Expósito-Palomo E, et al. Inflammatory-Related Clinical and Metabolic Outcomes in COVID-19 Patients. Mediators Inflamm 2020;2020:2914275. [Crossref] [PubMed]
- Soni M, Gopalakrishnan R. Significance of RDW in predicting mortality in COVID-19-An analysis of 622 cases. Int J Lab Hematol 2021;43:O221-3. [Crossref] [PubMed]
- Lanini S, Montaldo C, Nicastri E, et al. COVID-19 disease-Temporal analyses of complete blood count parameters over course of illness, and relationship to patient demographics and management outcomes in survivors and non-survivors: A longitudinal descriptive cohort study. PLoS One 2020;15:e0244129. [Crossref] [PubMed]
- Ku NS, Kim HW, Oh HJ, et al. Red blood cell distribution width is an independent predictor of mortality in patients with gram-negative bacteremia. Shock 2012;38:123-7. [Crossref] [PubMed]
- Leisman DE, Ronner L, Pinotti R, et al. Cytokine elevation in severe and critical COVID-19: a rapid systematic review, meta-analysis, and comparison with other inflammatory syndromes. Lancet Respir Med 2020;8:1233-44. [Crossref] [PubMed]
- Zizzo G, Cohen PL. Imperfect storm: is interleukin-33 the Achilles heel of COVID-19? Lancet Rheumatol 2020;2:e779-90. [Crossref] [PubMed]
- Elahi R, Karami P, Heidary AH, et al. An updated overview of recent advances, challenges, and clinical considerations of IL-6 signaling blockade in severe coronavirus disease 2019 (COVID-19). Int Immunopharmacol 2022;105:108536. [Crossref] [PubMed]
- RECOVERY Collaborative Group. Dexamethasone in Hospitalized Patients with Covid-19. N Engl J Med 2021;384:693-704. [Crossref] [PubMed]
- Fernández-Cruz A, Ruiz-Antorán B, Muñoz-Gómez A, et al. A Retrospective Controlled Cohort Study of the Impact of Glucocorticoid Treatment in SARS-CoV-2 Infection Mortality. Antimicrob Agents Chemother 2020;64:e01168-20. [Crossref] [PubMed]