Development and validation of a nomogram to predict chronic postoperative pain in elderly orthopedic patients
Introduction
Chronic postoperative pain (CPSP) refers to chronic pain that appears or increases in intensity after surgery and persists after the healing of tissue damage (generally believed to be more than 3 months) (1). Statistically, the incidence of CPSP ranges from 5% to 85% (2), according to the different types of surgery. Among these, orthopedic surgery is the highest (3-5), and severe CPSP accounts for 5–19% of CPSP patients (6). Studies have shown that CPSP will hinder the postoperative rehabilitation of patients, reduce their quality of life, and adversely affect their physical function (7). At the same time, CPSP will significantly increase the use of health care resources, with the associated costs exceeding those associated with heart disease, cancer, and diabetes in the United States (8).
At present, the average age of the world's population is increasing, with the proportion of people aged over 65 ranging from 12% in Ireland to 21% in countries such as Germany and Italy (9). As a country with the largest elderly population in the world, China is also one of the countries with the fastest aging population development. It is estimated that by 2025, the total number of people over 65 in China will be nearly 300 million. The aging problem is increasing and, more importantly, the number of elderly people undergoing surgery has increased faster than the aging of the population in the past 20 years (10). Elderly patients with age-related organ dysfunction and multiple complications of organ function impairment, resulting in the elderly patients with anesthesia and surgical trauma postoperative complications and mortality significantly higher than other adult patients.
Due to its high incidence in elderly patients and the difficulty of treatment (11), the prevention of CPSP has become one of the leading challenges faced by surgical clinical workers worldwide. The current study divided risk factors for CPSP into preoperative, operative and postoperative factors by time. Preoperative factors include preoperative pain history at surgical site, surgical history, etc. (12,13). The intraoperative factors leading to CPSP include type of surgery, anesthesia method, etc. Postoperative factors include acute postoperative pain (APSP), psychological vulnerability, etc. (14,15). Although some literatures have summarized the incidence of CPSP and related risk factors in different types of surgery, data reported by different studies are quite different, and there is no unified view on the establishment of risk factors for CPSP.
At present, the prevention and treatment of CPSP in elderly patients in China is still blank, especially the lack of reliable prediction model. Early identification of risk factors and preoperative screening of high-risk patients can facilitate prevention and early intervention for elderly patients, thereby reducing the surgery-related suffering of patients. Therefore, in this study, elderly patients undergoing orthopedic surgery were selected as a specific population to analyze the risk factors of CPSP, and to develop a predictive model, which was presented as a nomogram. Our nomogram was then internally and externally validated.
We present the following article in accordance with the TRIPOD reporting checklist (available at https://dx.doi.org/10.21037/apm-21-3041).
Methods
Research design and participants
This study was reviewed and approved by the Ethics and Research Committee of the General Hospital of Ningxia Medical University (No. 2020-559) and was conducted in accordance with the Declaration of Helsinki (as revised in 2013). Elderly patients (≥60 years) who underwent elective orthopedic surgery in our hospital from January 1, 2020 to January 31, 2021 were selected. Based on the inclusion and exclusion criteria, a total of 1,227 elderly orthopedic surgery patients were included. The study cohort was then divided into a model development cohort (2020.1.1–2020.10.19, n=815) and a model validation cohort (2020.10.20–2021.1.31, n=412) according to the date. The development cohort was used for both model development and internal validation, and the validation cohort was used for external validation of the model.
Data collection
The demographic and clinical characteristics of the subjects were obtained from the hospital’s electronic case system, including: age, sex, spouse or not, body mass index (BMI), education, smoking history, drinking history, preoperative pain history (at both the surgical site and non-surgical site), hypertension, diabetes, preoperative inflammatory state [defined as neutrophil-to-lymphocyte ratio (NLR) ≥2 (16)], American Society of Anesthesiologist (ASA) classification, type of surgery, anesthesia method, operation duration, intraoperative blood loss, postoperative analgesia, APSP or not, and postoperative hospital stay. The patients or their families were followed-up by telephone at 3 months postoperatively. The informed consent for the CPSP survey was read to the patients (or their families), and the questionnaire survey was conducted after obtaining their oral informed consent, which involved whether CPSP had occurred or not, and missing data such as education, pain history, and other data were supplemented.
Definition of CPSP
CPSP was defined by referring to the revised opinions of the International Association for the Study of Pain (IASP) on International Classification of Diseases 11th Revision (ICD-11) (6) as follows: (I) pain that develops or increases in intensity after a surgical procedure or a tissue injury and persists beyond the healing process, at least 3 months after the initiating event, with a numerical rating scale (NRS) score ≥1 point; (II) pain that is localized to the surgical field or area of injury, projected to the innervation territory of a nerve situated in this area, or referred to a dermatome or Head’s zone (after surgery/injury to deep somatic and visceral tissues); and (III) other causes of pain, such as pre-existing pain conditions or infections, or malignancy etc. were excluded in all cases of chronic post-traumatic and post-surgical pain.
Statistical analysis
Regarding the statistical description of the demographic and clinical characteristic data of the subjects, counting data were described by frequency and percentage, and measuring data were described by mean ± standard deviation (SD) or median (interquartile distance).
Univariate analysis was employed for all involved independent variables. Variables with statistical significance (P<0.1: in order to avoid the relationship between some independent variables and dependent variables being concealed by confounding factors) were further included in the multivariate binary logistic regression analysis, adopting the backward stepwise method. The final independent variables that will be introduced into the prediction model were selected according to Akaike information criterion (AIC) (17). The multicollinearity analysis adopted collinearity diagnosis; a variance inflation factor (VIF) of less than 10 can be considered to indicate no multicollinearity (18,19). If there was no multicollinearity relationship among the variables, the nomogram model was developed according to the contribution degree (regression coefficient) of the final prediction variable to the outcome variable.
The area under the curve (AUC) was used to measure the discrimination of the nomogram. Generally, an AUC <0.60 indicates poor discrimination, an AUC between 0.60 and 0.75 indicates certain discrimination, and an AUC >0.75 indicates good discrimination. The calibration of nomogram was evaluated by calculating the Brier score and drawing a calibration curve. A calibration curve displays the comparison between actual and predicted risk; the closer the curve is to diagonal, the better the prediction effect is. However, the discrimination and calibration of the model only considered the diagnostic accuracy, while ignoring the clinical utility of specific models. In order to meet the actual needs of clinical decision-making, decision curve analysis (DCA) was carried out; with net benefit as ordinate and high-risk threshold as abscissa, the clinical practicability of nomogram was evaluated from the intuitive decision curve.
Internal validation of the model applied the enhanced bootstrap method (20,21), which involves constructing a bootstrap-resampling sample with the same sample size by sampling in the development cohort. The model performance was evaluated by taking this sample as the training dataset and the development cohort as validation dataset. This process was repeated N times, and the Optimism of the model performance was obtained. Subsequently, the average value of Optimism for the performance of N models was considered the Optimism adjustment, which was obtained by calculating the difference between the model performance in the training and validation sets. The performance of the model in the original data minus the Optimism adjustment was taken as the performance of the model in the final internal validation.
Temporal validation was used for external validation of the model, which involves validating the performance of the model by using data from the same source as the model development cohort but with different time periods. The performance of the model was verified by the AUC and Brier scores.
All data were analyzed using SPSS software (version 25.0; SPSS Inc., Chicago, IL, USA) and R software (version 4.0.2; http://www.r-project.org, Vienna, Austria).
Results
Demographic and clinical characteristics
A total of 2,318 elderly orthopedic surgery patients recorded in the hospital’s electronic medical record system were initially screened. Patients who did not meet the inclusion criteria (n=616) were excluded, and 1,702 patients were enrolled in the postoperative follow-up study. Finally, 1,227 patients were included and analyzed. The patient selection flowchart is shown in Figure 1. The three datasets in this study consisted of 1,227 patients: an original training dataset (n=815), an internal validation dataset (n=815, obtained via the model development cohort using the enhanced Bootstrap resampling method), and an external validation dataset (n=412). The demographic and clinical characteristics of the different datasets are shown in Table 1.
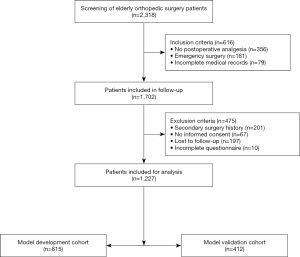
Table 1
Characteristics | Original training dataset (n=815) | Internal validation dataset (n=815) | External validation dataset (n=412) |
---|---|---|---|
CPSP | |||
Yes | 251 (30.8) | 253 (31.0) | 132 (32.0) |
No | 564 (69.2) | 562 (69.0) | 280 (68.0) |
Age (y) | 68.4±6.4 | 68.24±6.4 | 68.2±6.3 |
Sex | |||
Male | 305 (37.4) | 300 (36.8) | 133 (32.3) |
Female | 510 (62.6) | 515 (63.2) | 279 (67.7) |
Spouse | |||
Yes | 625 (76.6) | 648 (79.5) | 316 (76.7) |
No | 190 (23.3) | 167 (20.5) | 96 (23.3) |
Education | |||
Primary school and below | 532 (65.3) | 525 (64.4) | 282 (68.4) |
Middle school | 213 (26.1) | 220 (27.0) | 101 (24.5) |
University and above | 70 (8.6) | 70 (8.6) | 29 (7.1) |
BMI | |||
≤18.5 | 32 (3.9) | 36 (4.4) | 15 (3.6) |
18.5–23.9 | 338 (41.5) | 340 (417) | 148 (36.0) |
24.0–27.9 | 328 (40.2) | 325 (39.9) | 164 (39.8) |
≥28.0 | 117 (14.4) | 114 (14.0) | 85 (20.6) |
Smoking history | |||
Yes | 94 (11.5) | 94 (11.5) | 143 (34.7) |
No | 721 (88.5) | 721 (88.5) | 269 (65.3) |
Drinking history | |||
Yes | 28 (3.4) | 35 (4.3) | 9 (2.2) |
No | 787 (96.6) | 780 (95.7) | 403 (97.8) |
Preoperative pain at surgical site | |||
Yes | 428 (52.5) | 417 (51.2) | 241 (58.5) |
No | 387 (47.5) | 398 (48.8) | 171 (41.5) |
Preoperative pain at non-surgical site | |||
Yes | 286 (35.1) | 294 (36.1) | 186 (45.1) |
No | 529 (64.9) | 521 (63.9) | 226 (54.9) |
Hypertension | |||
Yes | 405 (49.7) | 415 (52.9) | 183 (44.4) |
No | 410 (50.3) | 400 (49.1) | 229 (55.6) |
Diabetes | |||
Yes | 127 (15.6) | 125 (15.3) | 56 (13.6) |
No | 688 (84.4) | 690 (84.7) | 356 (86.4) |
Preoperative inflammatory state | |||
Yes | 407 (49.9) | 411 (50.4) | 176 (42.7) |
No | 408 (50.1) | 404 (49.6) | 236 (57.3) |
ASA class | |||
Class I | 1 (0.1) | 0 (0) | 0 (0) |
Class II | 380 (46.6) | 386 (47.4) | 236 (57.3) |
Class III | 434 (53.3) | 429 (52.6) | 176 (42.7) |
Type of surgery | |||
Spinal | 338 (41.5) | 329 (40.4) | 145 (35.2) |
Joint replacement | 225 (27.6) | 218 (26.7) | 157 (38.1) |
Limb | 252 (30.9) | 268 (32.9) | 110 (26.7) |
Anesthesia method | |||
General anesthesia | 715 (87.7) | 707 (86.7) | 331 (80.3) |
Intraspinal anesthesia | 16 (2.0) | 20 (2.5) | 8 (2.0) |
Nerve block/local anesthesia | 84 (10.3) | 88 (10.8) | 73 (17.7) |
Operation duration (min) | 122.4±64.9 | 122.2±66.1 | 117.6±62.9 |
Postoperative analgesia | |||
PCIA | 660 (81.0) | 659 (80.9) | 298 (72.3) |
PCEA | 2 (0.2) | 3 (0.4) | 4 (1.0) |
PCNA | 153 (18.8) | 153 (18.8) | 110 (26.7) |
Intraoperative bleeding (mL) | 207.3±275.4 | 200.9±271.9 | 189.0±231.4 |
Postoperative hospital stay (d) | 5.7±3.4 | 5.7±3.3 | 4.9±2.5 |
APSP | |||
Yes | 182 (22.3) | 189 (23.2) | 109 (26.5) |
No | 633 (77.7) | 626 (76.8) | 303 (73.5) |
CPSP, chronic postoperative pain; BMI, body mass index; ASA, American Society of Anesthesiologist; PCIA, Patients controlled intravenous analgesia; PCEA, Patient controlled epidural analgesia; PCNA, Patient controlled nerve analgesia; APSP, acute postoperative pain.
Selection of independent variables
CPSP occurred in 251 (30.8%) of the 815 patients in the model development cohort. Nine variables that are significantly related to the occurrence of CPSP were selected as potential predictors of the prediction model through univariate analysis of all variables, including spouse or not, sex, education, preoperative pain at the surgical site, preoperative pain at a non-surgical site, type of surgery, postoperative analgesia, postoperative hospital stay, and whether there was APSP (Table 2).
Table 2
Variable | Pain (n=251) | No pain (n=564) | χ2/Z | P |
---|---|---|---|---|
Age (years) | 68.0±6.3 | 68.6±6.5 | −1.15 | 0.252 |
Sex | 4.11 | 0.043 | ||
Male | 81 (32.3) | 224 (39.7) | ||
Female | 170 (67.7) | 340 (60.3) | ||
Spouse | 24.33 | <0.001 | ||
Yes | 165 (65.7) | 460 (81.6) | ||
No | 86 (34.3) | 104 (18.4) | ||
Education | 7.94 | 0.019 | ||
Primary school and below | 177 (70.5) | 355 (62.9) | ||
middle school | 62 (24.7) | 151 (26.8) | ||
University and above | 12 (4.8) | 58 (10.3) | ||
BMI | 0.03 | 0.999 | ||
≤18.5 | 10 (4.0) | 22 (3.9 | ||
18.5–23.9 | 105 (41.8) | 233 (41.3) | ||
24.0–27.9 | 100 (39.8) | 228 (40.4) | ||
≥28.0 | 36 (14.3 | 81 (14.4) | ||
Smoking history | 0.01 | 0.990 | ||
Yes | 29 (11.6) | 65 (11.5) | ||
No | 222 (88.4) | 499 (88.5) | ||
Drinking history | 0.02 | 0.875 | ||
Yes | 9 (3.6) | 19 (3.4) | ||
No | 242 (96.4) | 545 (96.6) | ||
Preoperative pain at surgical site | 33.66 | <0.001 | ||
Yes | 170 (67.7) | 258 (45.7) | ||
No | 81 (32.3) | 306 (54.3) | ||
Preoperative pain at non-surgical site | 64.48 | <0.001 | ||
Yes | 137 (54.6) | 149 (26.4) | ||
No | 114 (45.4) | 415 (73.6) | ||
Hypertension | 0.17 | 0.679 | ||
Yes | 122 (48.6) | 283 (50.2) | ||
No | 129 (51.4) | 281 (49.8) | ||
Diabetes | 0.01 | 0.981 | ||
Yes | 39 (15.5) | 88 (15.6) | ||
No | 212 (84.5) | 476 (84.4) | ||
Preoperative inflammatory state | 0.31 | 0.579 | ||
Yes | 129 (51.4) | 278 (49.3) | ||
No | 122 (48.6) | 286 (50.7) | ||
ASA class | 0.53* | 0.875 | ||
Class I | 0 (0.0) | 1 (0.2) | ||
Class II | 119 (47.4) | 261 (46.3) | ||
Class III | 132 (52.6) | 302 (53.5) | ||
Type of surgery | 12.78 | 0.002 | ||
Spinal | 81 (32.3) | 257 (45.6) | ||
Joint replacement | 82 (32.7) | 143 (25.4) | ||
Limb | 88 (35.1) | 164 (29.1) | ||
Anesthesia method | 0.81* | 0.694 | ||
General anesthesia | 218 (86.9) | 497 (88.1) | ||
Intraspinal anesthesia | 4 (1.6) | 12 (2.1) | ||
Nerve block/local anesthesia | 29 (11.6) | 55 (9.8) | ||
Operation duration (min) | 118.0±63.2 | 124.3±65.6 | −1.28 | 0.201 |
Postoperative analgesia | 10.14* | 0.003 | ||
PCIA | 189 (75.3) | 471 (83.5) | ||
PCEA | 2 (0.8) | 0 (0.0) | ||
PCNA | 60 (23.9) | 93 (16.5) | ||
Intraoperative bleeding (mL) | 100 (50,300) | 100 (50,300) | −0.31 | 0.756 |
Postoperative hospital stay (d) | 6.1±3.6 | 5.5±3.3 | 2.37 | 0.018 |
APSP | 144.36 | <0.001 | ||
Yes | 122 (48.6) | 60 (10.6) | ||
No | 129 (51.4) | 504 (89.4) |
*, Fisher’s precision probability test. CPSP, chronic postoperative pain; BMI, body mass index; ASA, American Society of Anesthesiologist; PCIA, Patients controlled intravenous analgesia; PCEA, Patient controlled epidural analgesia; PCNA, Patient controlled nerve analgesia; APSP, acute postoperative pain.
We further carried out multivariate logistic regression analysis; variables with P<0.1 in the univariate analysis were included the into the multivariate analysis, and the backward stepwise method was adopted. The results showed that spouse or not, preoperative pain at the surgical site, preoperative pain at a non-surgical site, type of surgery, postoperative hospital stay, and APSP or not were independent risk factors associated with CPSP (Table 3).
Table 3
Variable | B | SE | Wald χ2 | df | OR | 95% CI | P value |
---|---|---|---|---|---|---|---|
Spouse (vs. not) | −0.894 | 0.204 | 19.230 | 1 | 0.409 | 0.274–0.610 | <0.0001 |
Preoperative pain at surgical site (vs. not) | 0.872 | 0.210 | 17.270 | 1 | 2.393 | 1.586–3.611 | <0.0001 |
Preoperative pain at non-surgical site (vs. not) | 1.383 | 0.196 | 50.015 | 1 | 3.987 | 2.718–5.850 | <0.0001 |
Type of surgery (vs. Spinal) | |||||||
Joint replacement | −0.073 | 0.238 | 0.094 | 1 | 0.929 | 0.675–1.714 | 0.759 |
Limb | 1.182 | 0.239 | 24.393 | 1 | 3.260 | 0.192–0.490 | <0.0001 |
Postoperative hospital stay (d) | 0.052 | 0.026 | 4.057 | 1 | 1.053 | 0.903–0.999 | 0.044 |
APSP (vs. not) | 2.098 | 0.214 | 96.570 | 1 | 8.151 | 5.364–12.386 | <0.0001 |
Constant | −2.417 | 0.309 | 57.304 | 0.089 | <0.0001 |
CPSP, chronic postoperative pain; APSP, acute postoperative pain.
According to the collinearity diagnosis of the above risk factors, the VIFs were 1.024, 1.298, 1.155, 1.438 (Joint replacement), 1.567 (Limb), 1.049, and 1.112, all of which were less than 10, indicating that there was no multicollinearity relationship among the seven independent risk factors.
Establishment and evaluation of the nomogram prediction model
The predictive nomogram of CPSP was established to predict CPSP by combining the above independent prediction variables (Figure 2).
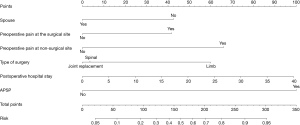
We performed a comprehensive evaluation of the nomogram. The AUC, which was used to determine the discrimination of the nomogram, showed that it had good diagnostic ability (AUC, 0.815, 95% CI: 0.783–0.847) (Figure 3). The calculated Brier score was 0.152, and the calibration curve was drawn (Figure 4), indicating that the nomogram had good calibration.
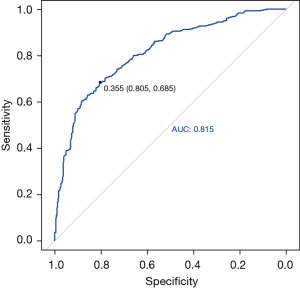
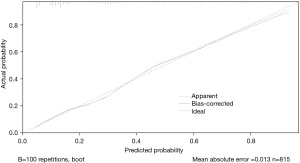
However, for clinical application, in addition to evaluating the discrimination and calibration of the model in mathematical statistics, we also need to evaluate its clinical practicability to determine whether patients can benefit from it. Therefore, we performed DCA, and the results showed that this nomogram has clinical practical value, and when the Threshold Probability (Pt) value is approximately 5–85%, patients can benefit from the predictive nomogram for the prediction of CPSP in elderly orthopedic patients (Figure 5).
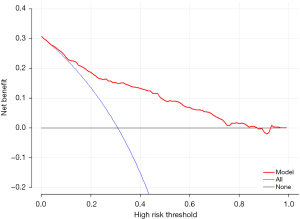
Internal and external validation of the nomogram prediction model
The enhanced Bootstrap method was adopted to obtain the internal validation cohort, which was repeated 100 times, and 100 internal validation iteration models were constructed. In the internal validation cohort, the mean AUC of the iterative models in the development cohort was 0.824, and the Optimism adjustment of the iterative models’ AUC was 0.010; thus, the final AUC of 0.814 indicated a good internal validation discrimination performance (Figure 6A). The average Brier score of the iterative models in the development cohort is 0.147, and the Optimism adjustment of iterative models’ Brier score was −0.005; therefore, the final Brier score was 0.152, which indicated a good calibration for internal validation with the calibration curve (Figure 6B,6C).
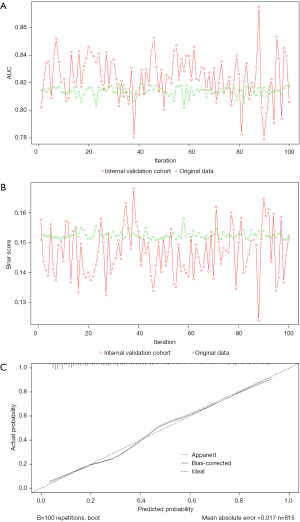
In the external validation cohort, the AUC was 0.798 (95% CI: 0.754–0.843), showing good discrimination (Figure 7A). The Brier score (0.165) and calibration curve (Figure 7B) also showed good calibration.
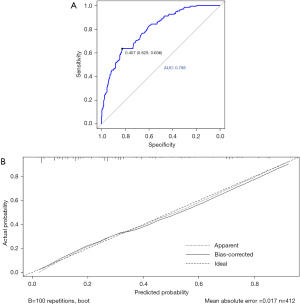
It can be seen from the DCA of patients in the internal and external validation cohorts that when the predictive nomogram is applied to the two validation cohorts, both groups of patients using this model can benefit from it, which has clinical application value (Figure 8A,8B).
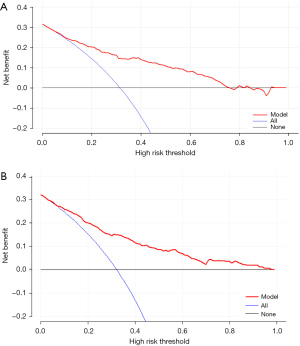
Discussion
As one of the most common complications after orthopedic surgery in the elderly, CPSP has various characteristics (including a long course of disease and complicated condition) and is difficult to completely cure by existing medical means, so prevention is the main focus at present (22,23). Therefore, doctors should carefully assess the risk of CPSP preoperatively, so as to make scientific clinical decisions and minimize the harm caused by CPSP (24,25). Unfortunately, there remains a lack of an individualized quantitative tool to accurately and effectively predict the probability of CPSP in elderly orthopedic patients. A clinical prediction model, as a quantitative tool for risk prediction and benefit evaluation, can provide more direct and rational information for individualized clinical decision-making between doctors and patients (25,26).
In this study, we developed and validated a nomogram for predicting the risk of CPSP in elderly orthopedic patients, and found that our predictive nomogram demonstrated excellent prediction ability, with an AUC of 0.815 (95% CI: 0.783–0.847), a Brier score of 0.152, and the calibration curve was close to diagonal. All of the above results indicated that this nomogram model can effectively and accurately predict the incidence of CPSP in elderly patients undergoing orthopedic surgery, and the DCA curve was higher than the two extreme curves (net benefit of 0 and net benefit of negative slope), suggesting that our nomogram has clinical practical value, which is beneficial for clinicians to prevent and treat CPSP early in elderly patients undergoing orthopedic surgery.
Our nomogram model consists of six variables (spouse or not, preoperative pain at surgical site, preoperative pain at a non-surgical site, type of surgery, postoperative hospital stay, and APSP or not), which were readily available in our clinical work. Therefore, this model is not only effective, but also a very practical forecasting tool. Even in basic hospitals with relatively limited medical resources, it can be used conveniently.
According to the strength of action, we found that the risk factors for increasing CPSP in elderly orthopedic surgery patients were as follows: APSP (OR 8.151), preoperative pain at non-surgical site (OR 3.987), limb surgery (OR 3.260), preoperative pain at surgical site (OR 2.393), long-term hospitalization (OR 1.053), and no spouse (OR 0.409). Numerous studies have demonstrated a strong correlation between APSP and CPSP (27-29); APSP occurring immediately after surgery is an important risk factor for the development of chronic pain and a key target of intervention measures to reduce the risk of CPSP (28). Buvanendran et al. (29) found that APSP was still a risk factor for CPSP after total knee arthroplasty (TKA), even after adjusting for confounding variables such as pain mutation, anxiety, depression, and functional status. This may be because severe early postoperative pain is a manifestation of a large amounts of nociceptive stimuli afferent to the central nervous system, resulting in central sensitization. Thus, the pain can last for a long time even after the peripheral nociceptive stimuli are weakened or even subside (28,30). Therefore, adequate control of APSP is an effective measure to reduce the occurrence of CPSP.
Similar findings have been found in some related studies regarding the history of preoperative pain history (2,31-33). However, most of the current studies have confirmed the correlation between the preoperative pain at surgical site and CPSP, excluding the preoperative pain at a non-surgical site (32-34). On the one hand, the reasons for this result may be that many studies have not included this risk factor in the analysis (32,33), and the types of surgeries studied were different (33,34). On the other hand, it is likely that these studies focused on elderly patients as a specific population, and the characteristic of combined preoperative pain at a non-surgical site is often seen in elderly patients, unlike previous studies. This also provides indirect evidence for the pathogenesis of CPSP; increasing research tends to suggest that CPSP is related to “central sensitization caused by inflammation of the nervous system” (35).
In this study, spouse status was included as a factor, and the results showed that the incidence of CPSP in patients without spouse was significantly higher than that in patients with spouse, and being without spouse was also an independent risk factor for the occurrence of CPSP. It is well known that the support of family and social relationships can facilitate rapid recovery after surgery. With the assistance of family members, especially spouses, such as undertaking more family affairs and providing emotional support to postoperative patients, the impact of surgical trauma on patients can be alleviated. Similar findings were found in previous studies by some scholars (36).
Due to its complexity, CPSP still cannot be prevented by a single measure. When CPSP occurs, pain management should be initiated as early as possible. At present, the treatment of CPSP is not optimistic. In addition to drug therapy (37), some case reports suggest the use novel ultrasound-guided blocks in the management of CPSP can obtain satisfactory results. In a pilot study following breast surgery, the authors investigated the analgesic effect of an ultrasound-guided pectoral nerve block with 20 mL of bupivacaine 0.25%. It was found that all eight patients reported pain relief and reduced sleep interference, up to 1 week after the block (38). In a 2017 case-series of patients with secondary chronic abdominal wall pain after surgery, ultrasound-guided transversus abdominis plan (TAP) block with local anesthetics and steroids provided more than 50% pain relief (39). While promising, all these findings need to be confirmed in randomized controlled trials. Therefore, we absolutely agree with the conclusion of Wylde et al. that “high-quality trials of multimodal interventions matched to pain characteristics are needed to provide robust evidence to guide management of CPSP” (40).
Limitations
Our research has several limitations that should be noted. Firstly, all independent variables in this study were collected from medical records rather than real-time collection, which did not include other important variables for analysis, such as perioperative social psychological state and neurocognitive function of patients. Secondly, due to the limited data sources of external validation, the external validation in this study was performed according to temporal validation (same source as model development cohort, but different time period), which is weaker than geographical validation and domain validation in terms of the ability to test the model’s transportability and generalizability (41). These deficiencies need to be improved in subsequent studies to improve the predictive ability of our nomogram.
Conclusions
Our study established a nomogram that can simply and effectively predict the occurrence of CPSP in elderly patients undergoing orthopedic surgery. In future, more detailed and complete prospective cohort studies with large samples should be designed to further improve and confirm the accuracy of our predictive nomogram.
Acknowledgments
Funding: This work was supported by grants from the Key Research & Developing Program of Ningxia Hui Autonomous Region, China (grant No. 2021BEG02036).
Footnote
Reporting Checklist: The authors have completed the TRIPOD reporting checklist. Available at https://dx.doi.org/10.21037/apm-21-3041
Data Sharing Statement: Available at https://dx.doi.org/10.21037/apm-21-3041
Conflicts of Interest: All authors have completed the ICMJE uniform disclosure form (available at https://dx.doi.org/10.21037/apm-21-3041). All authors report that this work was supported by grants from the Key Research & Developing Program of Ningxia Hui Autonomous Region, China (grant No. 2021BEG02036). The authors have no other conflicts of interest to declare.
Ethical Statement: The authors are accountable for all aspects of the work in ensuring that questions related to the accuracy or integrity of any part of the work are appropriately investigated and resolved. This study was conducted in accordance with the Declaration of Helsinki (as revised in 2013), and was reviewed and approved by the Ethics and Research Committee of General Hospital of Ningxia Medical University (No. 2020-559). Oral informed consent was obtained from all included patients during telephone follow-up.
Open Access Statement: This is an Open Access article distributed in accordance with the Creative Commons Attribution-NonCommercial-NoDerivs 4.0 International License (CC BY-NC-ND 4.0), which permits the non-commercial replication and distribution of the article with the strict proviso that no changes or edits are made and the original work is properly cited (including links to both the formal publication through the relevant DOI and the license). See: https://creativecommons.org/licenses/by-nc-nd/4.0/.
References
- Treede RD, Rief W, Barke A, et al. Chronic pain as a symptom or a disease: the IASP Classification of Chronic Pain for the International Classification of Diseases (ICD-11). Pain 2019;160:19-27. [Crossref] [PubMed]
- Schug SA, Bruce J. Risk stratification for the development of chronic postsurgical pain. Pain Rep 2017;2:e627 [Crossref] [PubMed]
- List EB, Krijgh DD, Martin E, et al. Prevalence of residual limb pain and symptomatic neuromas after lower extremity amputation: a systematic review and meta-analysis. Pain 2021;162:1906-13. [Crossref] [PubMed]
- Pinedo-Villanueva R, Khalid S, Wylde V, et al. Identifying individuals with chronic pain after knee replacement: a population-cohort, cluster-analysis of Oxford knee scores in 128,145 patients from the English National Health Service. BMC Musculoskelet Disord 2018;19:354. [Crossref] [PubMed]
- Echeverria-Villalobos M, Mitchell J, Fiorda-Diaz J, et al. Effects of Dorsal Column Spinal Cord Stimulation on Neuroinflammation: Revisiting Molecular Mechanisms and Clinical Outcomes on Chronic Lumbar/Leg Pain and Failed Back Surgery Syndrome. J Pain Res 2021;14:2337-45. [Crossref] [PubMed]
- Schug SA, Lavand'homme P, Barke A, et al. The IASP classification of chronic pain for ICD-11: chronic postsurgical or posttraumatic pain. Pain 2019;160:45-52. [Crossref] [PubMed]
- Gan TJ. Poorly controlled postoperative pain: prevalence, consequences, and prevention. J Pain Res 2017;10:2287-98. [Crossref] [PubMed]
- Institute of Medicine (US) Committee on Advancing Pain Research, Care, and Education. Relieving Pain in America: A Blueprint for Transforming Prevention, Care, Education, and Research. Washington (DC): National Academies Press (US); 2011.
- Robinson TN, Walston JD, Brummel NE, et al. Frailty for Surgeons: Review of a National Institute on Aging Conference on Frailty for Specialists. J Am Coll Surg 2015;221:1083-92. [Crossref] [PubMed]
- Kulason K, Nouchi R, Hoshikawa Y, et al. Indication of Cognitive Change and Associated Risk Factor after Thoracic Surgery in the Elderly: A Pilot Study. Front Aging Neurosci 2017;9:396. [Crossref] [PubMed]
- Esses GJ, Liu X, Lin HM, et al. Preoperative frailty and its association with postsurgical pain in an older patient cohort. Reg Anesth Pain Med 2019; Epub ahead of print. [Crossref] [PubMed]
- Hetmann F, Kongsgaard UE, Sandvik L, et al. Prevalence and predictors of persistent post-surgical pain 12 months after thoracotomy. Acta Anaesthesiol Scand 2015;59:740-8. [Crossref] [PubMed]
- Kampe S, Geismann B, Weinreich G, et al. The Influence of Type of Anesthesia, Perioperative Pain, and Preoperative Health Status on Chronic Pain Six Months After Thoracotomy-A Prospective Cohort Study. Pain Med 2017;18:2208-13. [PubMed]
- Springer JS, Karlsson P, Madsen CS, et al. Functional and structural assessment of patients with and without persistent pain after thoracotomy. Eur J Pain 2017;21:238-49. [Crossref] [PubMed]
- Richez B, Ouchchane L, Guttmann A, et al. The Role of Psychological Factors in Persistent Pain After Cesarean Delivery. J Pain 2015;16:1136-46. [Crossref] [PubMed]
- Turgut HC, Alkan M, Ataç MS, et al. Neutrophil lymphocyte ratio predicts postoperative pain after orthognathic surgery. Niger J Clin Pract 2017;20:1242-5. [Crossref] [PubMed]
- Aho K, Derryberry D, Peterson T. Model selection for ecologists: the worldviews of AIC and BIC. Ecology 2014;95:631-6. [Crossref] [PubMed]
- Bayman EO, Dexter F. Multicollinearity in Logistic Regression Models. Anesth Analg 2021;133:362-5. [Crossref] [PubMed]
- Kim JH. Multicollinearity and misleading statistical results. Korean J Anesthesiol 2019;72:558-69. [Crossref] [PubMed]
- Steyerberg EW, Harrell FE Jr. Prediction models need appropriate internal, internal-external, and external validation. J Clin Epidemiol 2016;69:245-7. [Crossref] [PubMed]
- Harrell FE. Regression Modeling Strategies: With Applications to Linear Models, Logistic and Ordinal Regression, and Survival Analysis. Springer, 2015.
- Steyaert A, Lavand'homme P. Prevention and Treatment of Chronic Postsurgical Pain: A Narrative Review. Drugs 2018;78:339-54. [Crossref] [PubMed]
- Richebé P, Capdevila X, Rivat C. Persistent Postsurgical Pain: Pathophysiology and Preventative Pharmacologic Considerations. Anesthesiology 2018;129:590-607. [Crossref] [PubMed]
- Weinrib AZ, Azam MA, Birnie KA, et al. The psychology of chronic post-surgical pain: new frontiers in risk factor identification, prevention and management. Br J Pain 2017;11:169-77. [Crossref] [PubMed]
- Montes A, Roca G, Cantillo J, et al. Presurgical risk model for chronic postsurgical pain based on 6 clinical predictors: a prospective external validation. Pain 2020;161:2611-8. [Crossref] [PubMed]
- Wang Y, Zhu Y, Xue Q, et al. Predicting chronic pain in postoperative breast cancer patients with multiple machine learning and deep learning models. J Clin Anesth 2021; Epub ahead of print. [Crossref] [PubMed]
- Liu YM, Feng Y, Liu YQ, et al. Chinese Association for the Study of Pain: Expert consensus on chronic postsurgical pain. World J Clin Cases 2021;9:2090-9. [Crossref] [PubMed]
- Fregoso G, Wang A, Tseng K, et al. Transition from Acute to Chronic Pain: Evaluating Risk for Chronic Postsurgical Pain. Pain Physician 2019;22:479-88. [PubMed]
- Buvanendran A, Della Valle CJ, Kroin JS, et al. Acute postoperative pain is an independent predictor of chronic postsurgical pain following total knee arthroplasty at 6 months: a prospective cohort study. Reg Anesth Pain Med 2019;44:e100036 [Crossref] [PubMed]
- Blichfeldt-Eckhardt MR. From acute to chronic postsurgical pain: the significance of the acute pain response. Dan Med J 2018;65:B5326. [PubMed]
- Skrejborg P, Petersen KK, Kold S, et al. Presurgical Comorbidities as Risk Factors For Chronic Postsurgical Pain Following Total Knee Replacement. Clin J Pain 2019;35:577-82. [Crossref] [PubMed]
- Yin Y, Zhang L, Xiao H, et al. The pre-amputation pain and the postoperative deafferentation are the risk factors of phantom limb pain: a clinical survey in a sample of Chinese population. BMC Anesthesiol 2017;17:69. [Crossref] [PubMed]
- Liu Y, Zhou M, Zhu X, et al. Risk and protective factors for chronic pain following inguinal hernia repair: a retrospective study. J Anesth 2020;34:330-7. [Crossref] [PubMed]
- Bayman EO, Parekh KR, Keech J, et al. A Prospective Study of Chronic Pain after Thoracic Surgery. Anesthesiology 2017;126:938-51. [Crossref] [PubMed]
- Mustonen L, Aho T, Harno H, et al. What makes surgical nerve injury painful? A 4-year to 9-year follow-up of patients with intercostobrachial nerve resection in women treated for breast cancer. Pain 2019;160:246-56. [Crossref] [PubMed]
- Mathes T, Pape-Köhler C, Moerders L, et al. External Validation and Update of the RICP-A Multivariate Model to Predict Chronic Postoperative Pain. Pain Med 2018;19:1674-82. [Crossref] [PubMed]
- Patterson TJ, Beck J, Currie PJ, et al. Meta-analysis of patient-reported outcomes after laparoscopic versus open inguinal hernia repair. Br J Surg 2019;106:824-36. [Crossref] [PubMed]
- Wijayasinghe N, Andersen KG, Kehlet H. Analgesic and Sensory Effects of the Pecs Local Anesthetic Block in Patients with Persistent Pain after Breast Cancer Surgery: A Pilot Study. Pain Pract 2017;17:185-91. [Crossref] [PubMed]
- Baciarello M, Migliavacca G, Marchesini M, et al. Transversus Abdominis Plane Block for the Diagnosis and Treatment of Chronic Abdominal Wall Pain Following Surgery: A Case Series. Pain Pract 2018;18:109-17. [Crossref] [PubMed]
- Wylde V, Dennis J, Beswick AD, et al. Systematic review of management of chronic pain after surgery. Br J Surg 2017;104:1293-306. [Crossref] [PubMed]
- Moons KG, Kengne AP, Grobbee DE, et al. Risk prediction models: II. External validation, model updating, and impact assessment. Heart 2012;98:691-8. [Crossref] [PubMed]
(English Language Editor: A. Kassem)