Systematic review and meta-analysis of the correlation between intestinal flora and gestational diabetes mellitus
Introduction
Gestational diabetes mellitus (GDM) is a disease unique to women during pregnancy. More than 80% of pregnant women with diabetes have GDM, and fewer than 20% of these women have diabetes before pregnancy (1). The incidence of GDM is reported to range from 1% to 14% worldwide (2). In China, the incidence is approximately 1% to 5%, but it has increased significantly in recent years (3). The glucose metabolism of most GDM patients returns to normal after delivery, but the chance of developing type II diabetes increases (4). Thus, the disease has a great effect on the outcome of a pregnancy and the health of the offspring.
During early and midterm pregnancy, the fetus’s demand for nutrients increases as the gestational weeks increase, and the fetus obtains glucose from the mother through the placenta, which is the main source of energy (5). The plasma glucose level of pregnant women decreases as the pregnancy progresses, and fasting blood glucose is reduced by approximately 10%. This is partly because estrogen and progesterone increase the utilization of glucose by the mother (6). Thus, the ability to clear glucose during pregnancy is stronger than that during nonpregnancy. The fasting blood sugar of pregnant women is lower than that of nonpregnant women, which is the pathological basis for the susceptibility to low blood sugar and ketoacidosis (7). During middle and late pregnancy, anti-insulin like substances, such as placental lactogen, estrogen, progesterone, cortisol, and placental insulinase, increase, which reduces the sensitivity of pregnant women to insulin. To maintain a normal level of glucose metabolism, there is an increased need for insulin. For pregnant women with restricted insulin secretion, the blood sugar level rises, which aggravates the original diabetes or leads to GDM. Additionally, women’s weight increases during pregnancy, and their resistance to insulin is raised. Once the body’s insulin level cannot maintain normal glucose metabolism, diabetes occurs. A comparison of the levels of intestinal flora during early and late pregnancy revealed that the occurrence of GDM was associated with the level of certain flora (8).
To further determine the correlation between the intestinal flora and GDM, randomized control trials (RCTs) involving intestinal flora and GDM were identified, and a systematic meta-analysis was conducted. We present the following article in accordance with the PRISMA reporting checklist (available at https://dx.doi.org/10.21037/apm-21-2061).
Methods
Literature inclusion and exclusion criteria
To be eligible for inclusion in the meta-analysis the, articles had to meet the following inclusion criteria: (I) involve female participants who were pregnant and who either had GDM (the experimental group) or normal glucose tolerance (the control group); (II) involve a RCT and be written in English; (III) in relation to the experimental group, include results related to fresh feces that had been collected in an aseptic anaerobic tank in the early morning to detect the intestinal flora, and 5 mL of fasting venous blood that had been drawn to detect inflammatory factors; (IV) in relation to the control group, include results related to fresh feces that had been collected in the early morning to detect intestinal flora and 3 mL of fasting venous blood that had been drawn to detect inflammatory factors (V) further, had comparable baseline data between the experimental group and the control group; and (VI) had outcome indicators that included advocacy strain and line tube inflammatory factors.
Conversely, articles were excluded from the meta-analysis if they met any of the following exclusion criteria: (I) were non-RCTs, such as retrospective studies, case reports, and cohort studies; (II) comprised subjects that were animals, cells, etc.; (III) had not been published, such as these, or were not in the English language; (IV) were irrelevant; and/or (V) contained incomplete research data.
Literature retrieval
The PubMed, Embase, MEDLINE, Ovid, Springer, and Web of Sciences databases were searched from the dates of establishment of the databases to December 31, 2020, using the following search terms: “Intestinal flora,” “Gestational diabetes mellitus,” and “Meta-analysis.” For each database, a joint search strategy of free words and subject words was adopted. After confirmation, the references were tracked using the search engine, and the latest research progress was obtained by contacting experts and researchers in the field.
Literature screening
The literature screening comprised three steps. First, studies that were not related to this area of research were excluded by reading the titles and abstracts. Second, studies that did not meet the inclusion or exclusion criteria were excluded through a reading of the full texts of the articles. Third, a quality evaluation was performed in which two senior experts independently screened the abstracts and full texts of the relevant articles. Three preliminary experiments were performed before the screening. Inconsistencies between the experts were resolved through discussion, and if a consensus could not be reached, a third expert was invited to arbitrate.
Data extraction
The two assessors independently used the self-developed data extraction table to extract data, which was then cross-checked after the extraction. The information extracted included: (I) the title of the article, first author (only 1 name included), the year of publication, and the publication journal; (II) the age, gender, sample size, and baseline comparability of the subjects; (III) the intervention measures and control measures; and (IV) the outcome indexes.
Quality evaluation
The bias risks of the included articles were assessed according to criteria provided in the Cochrane Handbook 5.0.2, which factored in selection bias, implementation bias, measurement bias, follow-up bias, and other biases including whether a random sequence had been used, whether allocation concealment had been adopted, whether a blind method had been used for the subjects; whether a blind method had been used for the outcome assessor; whether the data were complete; and whether there was selective reporting. Any inconsistency was solved by discussion or by asking another researcher to arbitrate.
Statistical methods
The Cochrane Handbook 5.0.2 was used to assess the bias risk of the articles, and Review Manager (RevMan) 5.3 was used for the meta-analysis. According to the analysis results, forest plots and funnel charts were output. In the meta-analysis, the literature was tested for heterogeneity, and the I2 test was used. If I2≥50% and P<0.05, there was homogeneity among the included articles, and the fixed-effects model (FEM) was used for the meta-analysis. If the I2<50% and P>0.05, there was heterogeneity among the included articles, and the random-effects models (REM) was used for the meta-analysis. The binary variables, including the incidence of adverse reactions, the relative risk (RR) of the effect size, and the 95% confidence intervals (95% CIs) were calculated. For continuous variables, such as conversion time, the weighted mean difference (WMD) was used as the effect size. If the units of the indicators were different, the standardized mean difference (SMD) was used as the effect size, and the 95% CI: was calculated. A U test (Z test) was used to judge whether there was statistical significance, and the P value was calculated according to the u value. α=0.05 was taken as the test standard, and a P value <0.05 was used as the threshold for significance. For binary variables, if the 95% CI did not contain 1 (i.e., 95% CI: >1 or <1), it was equivalent to P<0.05. For continuous variables, if the 95% CI did not contain 0 (i.e., 95% CI: >0 or <0), it was considered equivalent to P<0.05.
Results
Basic characteristics of the included literature
Initially, 764 documents were identified, including 133 from the PubMed database, 79 from the Embase database, 120 from the MEDLINE database, 101 from the Springer database, 156 from the Ovid database, and 175 from the Web of Science database. Next, 679 duplicates were eliminated, leaving 85 articles remaining. After a reading of the titles and abstracts, 44 articles were further eliminated according to the literature inclusion and exclusion criteria, and 39 were identified. Finally, after the 2 researchers read and cross-examined the full text, a total of 7 documents were included in the meta-analysis. They were all RCTs, published before 2020, and comprised a total of 665 participants. The baseline data, such as the age, of the experimental group and the control group were comparable (see Figure 1 and Table 1).
Table 1
The first author | Published year | Group | Sample size | Counter measure |
---|---|---|---|---|
Yu ( |
2018 | Experimental | 80 | Wet times + fasting venous blood |
Control | 60 | Feces + fasting venous blood | ||
Kuang ( |
2017 | Experimental | 43 | Wet times + fasting venous blood |
Control | 81 | Feces + fasting venous blood | ||
Festa ( |
2020 | Experimental | 14 | Wet times + fasting venous blood |
Control | 15 | Feces + fasting venous blood | ||
Ferrocino ( |
2018 | Experimental | 41 | Wet times + fasting venous blood |
Control | 41 | Feces + fasting venous blood | ||
Crusell ( |
2018 | Experimental | 50 | Wet times + fasting venous blood |
Control | 51 | Feces + fasting venous blood | ||
Xu ( |
2020 | Experimental | 30 | Wet times + fasting venous blood |
Control | 31 | Feces + fasting venous blood | ||
Hasan ( |
2018 | Experimental | 60 | Wet times + fasting venous blood |
Control | 68 | Feces + fasting venous blood |
Bias-risk assessment
The Cochrane Handbook for Systematic Reviews of Interventions 5.0.2 was used to evaluate the bias risk of the 7 articles included in this study, and RevMan 5.3 software was used to output the bias-risk map. The assessment items included the following: (I) random sequence generation—the 7 articles (9-15) all described the specific grouping method, suggesting a low risk; (II) allocation concealment—none of the 7 articles mentioned whether “allocation concealment” had been adopted, suggesting an unclear risk; (III) blinded method for the participants—3 of the 7 articles (11,13,15) mentioned that “the patient signed the informed consent form,” but did not mention whether the operator was blinded or not, suggesting an unclear risk; (IV) blind method for the outcome assessor—7 articles did not mention whether the outcome assessor was blinded, suggesting an unclear risk; (V) data integrity—the outcome data of the 7 articles were complete, suggesting a low risk; (VI) selective report—there was no selective report in the 7 articles, suggesting a low risk; (VII) other risks of bias—6 articles (9-11,13-15) had inconsistent numbers in the experimental and control groups, suggesting a high risk. It was undetermined whether the remaining 1 article (11) had other biases, suggesting an unclear risk. The results of the bias-risk assessment are shown in Figures 2 and 3.
Bifidobacterium
In this study, 5 studies (9,10,12,13,15), comprising 575 cases (274 in the experimental group and 301 in the control group), it compared the intestinal Bifidobacterium in the experimental group with that of control group patients. The heterogeneity test results (I2=99%; P<0.00001) suggested that there was a certain degree of heterogeneity among the studies; thus, the REM was used for the analysis. The analysis results are shown in Figure 4. The combined effect size of the meta-analysis was MD =–2.49 (95% CI: –3.54 to –1.45; Z=4.66; P<0.00001). The diamond in the forest plot was located on the left side of the vertical line, suggesting that the GDM patients in the experimental group had a lower level of Bifidobacterium in the intestine than did those in the control group.
Lactobacillus
In this study, 3 studies (9,11,13) comprising a total of 270 cases (144 in the experimental group and 126 in the control group), analyzed intestinal lactobacilli. The heterogeneity test results (I2=0%; P=0.69) suggested that there was no heterogeneity among the studies; thus, the FEM was used for the analysis. The analysis results are shown in Figure 5. The combined effect size of the meta-analysis was MD =–1.69 (95% CI: –1.84 to –1.53; Z=20.66; P<0.00001), and the diamond in the forest plot was located on the left side of the vertical line, suggesting that the GDM patients in the experimental group had a lower level of lactobacillus in the intestine than did those in the control group.
Bacteroides
In this study, 3 studies (9,10,15), comprising a total of 392 cases (183 in the experimental group and 209 in the control group), analyzed the intestinal Bacteroides of patients. The heterogeneity test results (I2=89%; P<0.0001) suggested that there was heterogeneity among the studies; thus, the REM was used for the analysis. The analysis results are shown in Figure 6. The combined effect size of the meta-analysis was MD =–1.17 (95% CI: –1.45 to –0.89; Z=8.15; P<0.00001). The diamond in the forest diagram was on the left side of the vertical line, suggesting that the GDM patients in the experimental group had a lower level of Bacteroides in the intestine than did those in the control group.
Enterobacter
In this study, 5 studies (9,11,12,14,15) comprising 440 cases (225 in the experimental group and 215 in the control group) analyzed intestinal Enterobacter. The heterogeneity test results (I2=92%; P<0.00001) indicated that there was heterogeneity among the studies; thus, the REM was used for the analysis. The analysis results are shown in Figure 7. The combined effect size of the meta-analysis was MD =1.79 (95% CI: 1.13 to 2.45; Z=5.3; P<0.00001), and the diamond in the forest plot was located on the right side of the vertical line, suggesting that the GDM patients in the experimental group had a higher level of Enterobacter than did those in the control group.
Enterococcus
In this study, 3 studies (12,13,15) comprising a total of 311 cases (151 in the experimental group and 160 in the control group) analyzed intestinal enterococci. The heterogeneity test results (I2=0%; P=0.65) suggested that there was no heterogeneity among the studies; thus, the FEM was used for the analysis. The analysis results are shown in Figure 8. The combined effect size of the meta-analysis was MD =1.29 (95% CI: 0.98 to 1.6; Z=8.06; P<0.00001). The diamond in the forest plot was located on the right side of the vertical line, suggesting that the GDM patients in the experimental group had a higher level of Enterococcus in the intestine than those in the control group.
Bacteroidetes
In this study, 3 studies (10,11,13) comprising a total of 254 cases (107 in the experimental group and 147 in the control group) analyzed intestinal Bacteroidetes. The heterogeneity test results (I2=84%; P=0.002) suggested that there was a certain degree of heterogeneity among the studies; thus, the REM was used for the analysis. The analysis results are shown in Figure 9. The combined effect size of the meta-analysis was MD =–1.22 (95% CI: –1.71 to –0.72; Z=4.81; P<0.00001). The diamond in the forest plot was located on the left side of the vertical line, suggesting that the GDM patients in the experimental group had a lower level of Bacteroidetes in the intestine did than those in the control group.
Fusobacterium
In the study, 3 studies (12,13,15), comprising a total of 311 cases (151 in the experimental group and 160 in the control group), analyzed intestinal fusobacterium. The heterogeneity test results (I2=0%; P=0.86) suggested there to be no heterogeneity among the studies; thus, the FEM was used for the analysis. The analysis results are shown in Figure 10. The combined effect size of the meta-analysis was MD =0.03 (95% CI: –0.13 to 0.19; Z=0.37; P=0.71). The diamond in the forest plot was located on the right side of the vertical line, suggesting that the GDM patients in the experimental group had a lower level of Fusobacterium than did those in the control group.
Tumor necrosis factor alpha
In this study, 4 studies (9,11,12,14), comprising a total of 312 cases (165 in the experimental group and 147 in the control group), analyzed the level of tumor necrosis factor alpha (TNF-α). The heterogeneity test results (I2=100%; P<0.00001) suggested that there was a certain degree of heterogeneity among the studies; thus, the REM was used for the analysis. The analysis results are shown in Figure 11. The combined effect size of the meta-analysis was MD =113.66 (95% CI: 52.01 to 175.31; Z=3.61; P=0.0003). The diamond in the forest plot was located on the right side of the vertical line, suggesting that the GDM patients in the experimental group had a higher level of TNF-α in the intestine than did those in the control group.
Interleukin 17
In this study, 3 studies (9,10,13), comprising a total of 365 cases (173 in the experimental group and 132 in the control group) analyzed the level of interleukin (IL)-17. The heterogeneity test results (I2=99%; P<0.00001) suggested that there was heterogeneity among the studies; thus, the REM was used for the analysis. The analysis results are shown in Figure 12. The combined effect size of the meta-analysis was MD =37.92; 95% CI: 29.74 to 46.1; Z=9.09; P<0.00001]. The diamond in the forest plot was located on the right side of the vertical line, suggesting that the GDM patients in the experimental group had a higher level of IL-17 than did those in the control group.
Interleukin 6
In this study, 3 studies (9,10,12), comprising a total of 346 cases (164 in the experimental group and 182 in the control group), analyzed the level of IL-6. The heterogeneity test results (I2=100%; P<0.00001) suggested that there was heterogeneity among the studies; thus, the REM was used for the analysis. The analysis results are shown in Figure 13. The combined effect size of the meta-analysis was MD =66.38 (95% CI: 33.6 to 99.15; Z=3.97; P<0.0001). The diamond in the forest plot was located on the right side of the vertical line, suggesting that the GDM patients in the experimental group had a higher level of IL-6 than did those in the control group.
Publication bias
RevMan 5.3 software was used to evaluate the publication bias. The analysis results are shown in Figure 14. The points of Bifidobacterium, Lactobacillus, Enterococcus, Bacteroidetes, and Fusobacterium was basically distributed within the CI, and the literature bias was low, while the points of Bacteroides, Enterobacter, TNF-α, IL-17, and IL-6 were distributed out of the CI, and the distribution was scattered, indicating that there was a certain publication bias in the included literature.
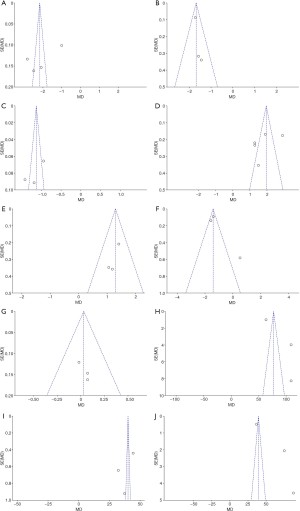
Discussion
With the shift in lifestyle of modern society, the intake of high-energy diets during pregnancy, and the changes in the metabolism of pregnant women during pregnancy, the incidence of GDM has increased year by year (16,17). Intestinal flora refers to the bacteria that inhabit the human gastrointestinal tract, which has done since the beginning of the human species, gradually developing into normal flora. Intestinal flora mainly colonizes the colon and depends on the digestion of food residues to meet the energy needs of survival (18-20). There are about 1014 microorganisms in the human intestine, which is about 10 times the total number of human cells, and they participate in the body’s carbohydrate metabolism, fat metabolism, and other physiological processes (21). Imbalance of the intestinal flora causes changes in the proportion of the flora, increases the permeability of the intestinal wall, and produces many inflammatory factors (22). In addition to having reasonable dietary structure, the main way for pregnant women to improve their intestinal flora is to adjust the intestinal environment and prevent problems by using dietary supplements (23). Probiotics have two basic functions: one is to balance the intestinal flora, maintain healthy active microorganisms in the intestine, and promote intestinal peristalsis; the other is to stimulate the intestinal immune function and enhance immunity. For pregnant women, studies have shown that probiotics can reduce the incidence of GDM, improve maternal immunity, and regulate the body’s absorption of sugars, lipids, and other substances in food (24). A previous endotoxin theory contended that physiological changes during pregnancy lead to changes of dietary habits to varying degrees. Dietary habits largely affect the composition of the intestinal flora, and the composition of the intestinal flora is associated with the intestinal barrier function (25-27). For example, a long-term high-fat diet during pregnancy decreases the expression of tight junction proteins in intestinal epithelial cells and increases the permeability of the intestinal mucosa so that a large amount of bacterial lipopolysaccharide is released into the blood, activating the low-grade chronic inflammation of islets. Inflammation can lead to the structural damage and dysfunction of pancreatic islet B cells, promote B cell apoptosis, and cause insufficient insulin secretion; long-term low-grade inflammation leads to weakened insulin signal transduction and sensitivity, which in turn triggers GDM (28).
To systematically analyze the relationship between intestinal flora and GDM, a total of 7 reports were included in this meta-analysis. It was found that, except for Bifidobacterium, Lactobacillus, Bacteroides, and Bacteroidetes, whose diamonds were located on the left side, the indicators had diamonds located on the right side, suggesting that the intestinal flora was associated with the occurrence of GDM. Bifidobacterium and Lactobacillus, as human probiotics, mainly regulate intestinal microbial disorders. When GDM occurs, the relative levels of the 2 decrease, while the relative levels of Enterobacter and Enterococcus increase. It has been speculated that changes in the level of probiotics, such as in Bifidobacterium and Lactobacillus, may be an important internal factor for the occurrence of GDM due to the intestinal flora (29). Additionally, the changes of Bacteroidetes and Fusobacteria did not show specificity in this meta-analysis; however, previous studies have found that the structural changes of Clostridium sphaericus and Bacteroidetes polymorpha are correlated with the occurrence of GDM (30). TNF-α, IL-17, and IL-6 are all inflammatory factors. Studies have shown that these inflammatory factors are directly related to inflammatory bowel disease (31). They can promote the shedding of colonic epithelial cells and participate in the inflammatory response of the lamina propria, thereby increasing the permeability of cells in the colon.
Conclusions
In this meta-analysis on the correlation between intestinal flora with GDM, a total of 7 studies were included, comprising 665 cases. It was found that changes in the intestinal microecology were closely related to the occurrence of GDM, which mainly manifested as a decrease in the level of probiotics, an increase in the level of intestinal bacteria and other strains, and an increase in the level of inflammatory factors. However, the limitations of this meta-analysis should be noted. Some studies had a large publication bias. Further, some analysis indicators contained a small number of samples, and the meta-analysis results were not sufficiently accurate. Thus, in follow-up research, studies with larger sample sizes and of a high quality need to be included to verify the association between GDM and intestinal flora.
Acknowledgments
Funding: None.
Footnote
Reporting Checklist: The authors have completed the PRISMA reporting checklist. Available at https://dx.doi.org/10.21037/apm-21-2061
Conflicts of Interest: All authors have completed the ICMJE uniform disclosure form (available at https://dx.doi.org/10.21037/apm-21-2061). The authors have no conflicts of interest to declare.
Ethical Statement: The authors are accountable for all aspects of the work in ensuring that questions related to the accuracy or integrity of any part of the work are appropriately investigated and resolved.
Open Access Statement: This is an Open Access article distributed in accordance with the Creative Commons Attribution-NonCommercial-NoDerivs 4.0 International License (CC BY-NC-ND 4.0), which permits the non-commercial replication and distribution of the article with the strict proviso that no changes or edits are made and the original work is properly cited (including links to both the formal publication through the relevant DOI and the license). See: https://creativecommons.org/licenses/by-nc-nd/4.0/.
References
- Johns EC, Denison FC, Norman JE, et al. Gestational Diabetes Mellitus: Mechanisms, Treatment, and Complications. Trends Endocrinol Metab 2018;29:743-54. [Crossref] [PubMed]
- Yuan K, Wang H, Chen Y, et al. A 12-hour comprehensive nutrition care benefits blood glucose level and weight gain and improves outcomes in pregnant women with gestational diabetes mellitus. Ann Palliat Med 2020;9:661-70. [Crossref] [PubMed]
- ACOG Practice Bulletin No. 190 Summary: Gestational Diabetes Mellitus. Obstet Gynecol 2018;131:406-8. [Crossref] [PubMed]
- Homayouni A, Bagheri N, Mohammad-Alizadeh-Charandabi S, et al. Prevention of Gestational Diabetes Mellitus (GDM) and Probiotics: Mechanism of Action: A Review. Curr Diabetes Rev 2020;16:538-45. [Crossref] [PubMed]
- Immanuel J, Simmons D. Screening and Treatment for Early-Onset Gestational Diabetes Mellitus: a Systematic Review and Meta-analysis. Curr Diab Rep 2017;17:115. [Crossref] [PubMed]
- Agha-Jaffar R, Oliver N, Johnston D, et al. Gestational diabetes mellitus: does an effective prevention strategy exist? Nat Rev Endocrinol 2016;12:533-46. [Crossref] [PubMed]
- Świrska J, Zwolak A, Dudzińska M, et al. Gestational diabetes mellitus - literature review on selected cytokines and hormones of confirmed or possible role in its pathogenesis. Ginekol Pol 2018;89:522-7. [Crossref] [PubMed]
- Cortez RV, Taddei CR, Sparvoli LG, et al. Microbiome and its relation to gestational diabetes. Endocrine 2019;64:254-64. [Crossref] [PubMed]
- Yu H, Liu Z, Dong S. Changes in Intestinal Flora, TNF-α, L-17, and IL-6 Levels in Patients with Gestational Diabetes Mellitus. European Journal of Inflammation 2018;16:2058739218793550 [Crossref]
- Kuang YS, Lu JH, Li SH, et al. Connections between the human gut microbiome and gestational diabetes mellitus. Gigascience 2017;6:1-12. [Crossref] [PubMed]
- Festa C, Drago L, Martorelli M, et al. Flash on gut microbiome in gestational diabetes: a pilot study. New Microbiol 2020;43:195-7. [PubMed]
- Ferrocino I, Ponzo V, Gambino R, et al. Changes in the gut microbiota composition during pregnancy in patients with gestational diabetes mellitus (GDM). Sci Rep 2018;8:12216. [Crossref] [PubMed]
- Crusell MKW, Hansen TH, Nielsen T, et al. Gestational diabetes is associated with change in the gut microbiota composition in third trimester of pregnancy and postpartum. Microbiome 2018;6:89. [Crossref] [PubMed]
- Xu Y, Zhang M, Zhang J, et al. Differential intestinal and oral microbiota features associated with gestational diabetes and maternal inflammation. Am J Physiol Endocrinol Metab 2020;319:E247-53. [Crossref] [PubMed]
- Hasan S, Aho V, Pereira P, et al. Gut microbiome in gestational diabetes: a cross-sectional study of mothers and offspring 5 years postpartum. Acta Obstet Gynecol Scand 2018;97:38-46. [Crossref] [PubMed]
- Filardi T, Panimolle F, Crescioli C, et al. Gestational Diabetes Mellitus: The Impact of Carbohydrate Quality in Diet. Nutrients 2019;11:1549. [Crossref] [PubMed]
- Moon JH, Kwak SH, Jang HC. Prevention of type 2 diabetes mellitus in women with previous gestational diabetes mellitus. Korean J Intern Med 2017;32:26-41. [Crossref] [PubMed]
- Law KP, Zhang H. The pathogenesis and pathophysiology of gestational diabetes mellitus: Deductions from a three-part longitudinal metabolomics study in China. Clin Chim Acta 2017;468:60-70. [Crossref] [PubMed]
- Buckley BS, Harreiter J, Damm P, et al. Gestational diabetes mellitus in Europe: prevalence, current screening practice and barriers to screening. A review. Diabet Med 2012;29:844-54. [Crossref] [PubMed]
- Abell SK, De Courten B, Boyle JA, et al. Inflammatory and Other Biomarkers: Role in Pathophysiology and Prediction of Gestational Diabetes Mellitus. Int J Mol Sci 2015;16:13442-73. [Crossref] [PubMed]
- Juan J, Yang H. Prevalence, Prevention, and Lifestyle Intervention of Gestational Diabetes Mellitus in China. Int J Environ Res Public Health 2020;17:9517. [Crossref] [PubMed]
- Edwards SM, Cunningham SA, Dunlop AL, et al. The Maternal Gut Microbiome During Pregnancy. MCN Am J Matern Child Nurs 2017;42:310-7. [Crossref] [PubMed]
- Gomez-Arango LF, Barrett HL, Wilkinson SA, et al. Low dietary fiber intake increases Collinsella abundance in the gut microbiota of overweight and obese pregnant women. Gut Microbes 2018;9:189-201. [Crossref] [PubMed]
- Yang H, Guo R, Li S, et al. Systematic analysis of gut microbiota in pregnant women and its correlations with individual heterogeneity. NPJ Biofilms Microbiomes 2020;6:32. [Crossref] [PubMed]
- Moreno-Castilla C, Mauricio D, Hernandez M. Role of Medical Nutrition Therapy in the Management of Gestational Diabetes Mellitus. Curr Diab Rep 2016;16:22. [Crossref] [PubMed]
- Xu T, He Y, Dainelli L, et al. Healthcare interventions for the prevention and control of gestational diabetes mellitus in China: a scoping review. BMC Pregnancy Childbirth 2017;17:171. [Crossref] [PubMed]
- Pan J, Pan Q, Chen Y, et al. Efficacy of probiotic supplement for gestational diabetes mellitus: a systematic review and meta-analysis. J Matern Fetal Neonatal Med 2019;32:317-23. [Crossref] [PubMed]
- Hod M, Kapur A, McIntyre HD, et al. Evidence in support of the International Association of Diabetes in Pregnancy study groups' criteria for diagnosing gestational diabetes mellitus worldwide in 2019. Am J Obstet Gynecol 2019;221:109-16. [Crossref] [PubMed]
- Blumberg J, Ballares V, Durbin JL. Ethnic variations on gestational diabetes mellitus and evidence-based first-line interventions. J Matern Fetal Neonatal Med 2018;31:2641-7. [Crossref] [PubMed]
- Li G, Yin P, Chu S, et al. Correlation Analysis between GDM and Gut Microbial Composition in Late Pregnancy. J Diabetes Res 2021;2021:8892849 [Crossref] [PubMed]
- Hu P, Chen X, Chu X, et al. Association of Gut Microbiota during Early Pregnancy with Risk of Incident Gestational Diabetes Mellitus. J Clin Endocrinol Metab 2021:dgab346.
(English Language Editor: L. Huleatt)