Development of SAB model for predicting mortality in intensive care unit after aortic aneurysm surgery
Introduction
Aortic aneurysm (AA) is a disease about the dilation of aorta, larger than one and a half times of normal vessel diameter. According to anatomical classification, it can be divided into abdominal aortic aneurysm (AAA), thoracic aortic aneurysm (TAA) and thoracoabdominal aortic aneurysm (TAAA). The in-hospital mortality rate of AA varies widely from 2 to 20 percent (1-4), while the mortality of TAA is the highest among these three types (5,6). Under normal circumstances, the treatment options for patients with AA include open repair surgery (ORS) and endovascular aneurysm repair (EVAR). After the operation, the patients will have many complications because of organ ischemia or aortic repair surgery (7). Common complications include acute renal failure, endoleaks, graft rupture, graft thrombosis and so on (8,9). Some patients suffered from more than one complication and some needed a secondary intervention (10). For example, a thrombus at the edge of the anastomosis after surgery can block the distal artery and cause organ ischemia. Some of the abdominal aorta aneurysm that needs to be cut above the level of the renal artery can cause kidney ischemia. Most seriously, anastomotic leakage may occur in artificial blood vessels, which cause internal hemorrhage. Once internal hemorrhage occurs, a second operation should be performed immediately or the patient will die (11,12). Due to these complications, the post-operative mortality rate is still between 5 to 10 percent (5,13).
Therefore, when postoperative AA patient’s condition is unstable, it is vital to transfer them to intensive care unit (ICU) for health support and observation. However, ICU admission can cost a lot and sometimes is a waste of hospital resource. A recent study indicated that ICU admission was often unnecessary after EVAR (14). If a patient’s mortality after operation is known before admission to ICU, surgeons can make a more suitable and accurate treatment plan and choose when to leave ICU. The establishment of predicting model can help doctors predict the ICU mortality rate quickly. Simplified acute physiology score II (SAPSII) and sequential organ assessment (SOFA) are widely used to measure the severity of ICU patients. It was proved that SAPSII and SOFA can indicate the mortality in ruptured AAA within 48 hours (15). But sometimes these models are cumbersome to use, model especially and easily measuring the mortality of AA patients after surgery is still to be built. We present the following article in accordance with the TRIPOD reporting checklist (available at https://dx.doi.org/10.21037/apm-21-1660).
Methods
Data source & ethical statement
We used the data obtained from the Medical Information Mart for Intensive Care III (MIMIC-III) database (16). The MIMIC-III database is open to the public free of charge and it uses international classification of diseases, 9th revision (ICD-9) diagnostic code for the determination of patients’ condition. This study was conducted in accordance with the Declaration of Helsinki (as revised in 2013).
Population selection
Firstly, we used ICD-9 code including 4411, 4412, 4413, 4414, 4416 and 4417 to select the study cohort diagnosed as AA (4411: thoracic aneurysm, ruptured; 4412: thoracic aneurysm without mention of rupture; 4413: abdominal aneurysm, ruptured; 4414: abdominal aneurysm without mention of rupture; 4416: thoracoabdominal aneurysm, ruptured; 4417: thoracoabdominal aneurysm, without mention of rupture). The studying cohort including rupture or non-rupture type, elective or urgent type. By using ICD-9 code 3844 (Resection of vessel with replacement, aorta, abdominal), 3845 (Resection of vessel with replacement, thoracic vessels), 3973 (Endovascular implantation of graft in thoracic aorta) and 3971 (Endovascular implantation of other graft in abdominal aorta), we selected the cohorts who received vascular surgery. Patients or the ICU admission were excluded meeting the following criteria: (I) who didn’t have surgery before admitting to ICU; (II) for those who had multiple ICU admissions, we only remained the first admission record; (III) of whom ICU stay were less than 24 hours; (IV) who lacked of anion gap record in the first day of ICU admission; (V) who were younger than 18.
Data extraction and data processing
We extracted the admission baseline data of selected study cohort, which included general condition, comorbidity, vital signs on admission, laboratory indicators, surgery-related indications and severity scores. In the vital signs on admission, heart rate, systolic blood pressure, diastolic blood pressure, respirate rate and percutaneous oxygen saturation (SpO2) were all the average values of the data collected on the first day of ICU after surgery. In laboratory indicators, the anion gap, bicarbonate, creatinine, blood urea nitrogen, partial thromboplastin time (PTT), international standard ratio (INR), prothrombin time (PT), white blood cell count and platelet count were defined as the maximum and minimum values of data collected on the first day. In the surgery-related indications, way of surgery (ORS, EVAR and mixed), extracorporeal circulation used, bypass surgery used, ventilation used in the first day of ICU, urine output in first day of ICU were also collected. Outcome was defined as in-ICU mortality. Above data extraction were conducted on PostgreSQL software (version 10.12, www.postgresql.org).
Statistical analysis
In the baseline data of patients, continuous variables with abnormal distribution of the two groups were represented by the median with interquartile range (IQR), compared with Kruskal Wallis test. The categorical variables were expressed as percentage, compared with Chi-square tests. Single imputation was then performed for the whole dataset based on the complete conditional specification and predictive mean matching method was used to fill the missing value.
Model building
Admission baseline variables [excluded length of stay (LOS) of hospital and ICU, type of AA] that had statistical significance in univariate analysis were then put into least absolute shrinkage and selection operator (LASSO) to select the final model according to the LASSO results and clinical significance. The selected variables were then put into logistic regression to build a new prediction model. After that, two widely used models (SAPSII and SOFA) and the newly built model were used to calculate the discrimination and calibration in the original data. Discrimination was measured by area under the curve (AUC) while calibration was measured by Brier score and calibration curve. The following formula was used to calculate Brier score:
In the formula, N represents the total number of predictions, ft represents the actual results and 0t presents the predicted probability of the model. Discrimination and calibration, also with net reclassification improvement (NRI) and integrated discrimination improvement (IDI) were used as indicators to compare the prediction ability of the newly built model with the other models. NRI could be measured as:
NRI can reflect the ability improvement in true classification of the new model compared to the old model. And IDI can be measured as:
Pnew, events and Pold, events represent mean probability of mortality (or outcome events) predicted by the new and old model in outcome-occurrence group, while Pnew, non-events and Pold, non-events represent mean probability of mortality (or outcome events) predicted by the new and old model in outcome-nonoccurrence group. IDI can reflect change of predicted probability between the new and old model, and a higher IDI means a better prediction ability of the new model. Then internal validation was conducted, optimism bootstrap method with 1,000 repetition was used to correct the AUC, brier score and calibration curve. In order to evaluate the risk stratification ability of the SAB model, the study cohort had been divided into 5 groups based on the predicted probability: (I) 0–20%; (II) 20–40%; (III) 40–60%; (IV) 60–80%; (V) 80–100%. And the actual number of deaths and death rates were counted in each group to show whether if SAB model can identify the high-risk group. Then a decision clinical analysis (DCA) curve was conducted to estimate the clinical usefulness and net benefit. For its convenient application in clinic, we presented the newly built model as a website. Finally, we also performed an external validation of SAB model in a large ICU cohort (31,645 patients) to investigate whether SAB model can also be equally applicable in non-aorta ICU patients. The result of external validation is not shown in text and many more regressions were run than can be included in the article. The interested reader can find them in Appendix 1. Above data were analyzed with R software (version.3.6.1; The R Project for Statistical Computing, TX, USA; http://www.r-project.org).
Results
At first, 609 patients with 667 ICU admission records were identified from MIMIC-III. After selection, 127 patients and 185 ICU records were excluded and at last, 482 AA patients [33 patients (6.85%) died in ICU] and 482 ICU records were enrolled in this study. The enrollment flowchart is shown in Figure 1. Rupture cases make up 14.5% of the entire cohort. Emergency and urgent type make up 31.3% of the entire cohort. Table 1 shows the baseline data of patients. In the univariate analysis of patients’ baseline data categorized according to clinical outcome, as for general condition, LOS of ICU, in-hospital death, type of AA, rupture of AA, age and gender had statistical significances. As for comorbidity, sepsis and coagulopathy disorders had statistical significances. As for vital signs on admission, the mean of heart rate, systolic blood pressure, respirate rate and SpO2 had statistical significances. In laboratory indicators, both maximum and minimum value of anion gap, bicarbonate, creatinine, blood urea nitrogen had statistical significances; maximum value of PTT, INR and white blood cell count had statistical significances; minimum value of PT and platelet count had statistical significances. In surgery-related indicators, extracorporeal circulation used and urine output in first day of ICU had statistical significances. In severity score, SAPSII and SOFA had statistical significances. To build a new model, we used cross-validation of logistic regression of LASSO to screen all the statistically significant baseline variables (excluded LOS of hospital and ICU, type of AA) of 482 patients. Figure 2 shows the relationship between penalty coefficient λ and the continuous decrease of variables in the model. Finally, we got the final model with 3 variables and the penalty coefficient λ is 0.0545. The 3 variables included sepsis, anion gapmaximum and bicarbonateminimum. Then we put these variables into multivariable logistic regression to fit the model. Our final model was called SAB model based on initials of three items [Sepsis, Anion gap, Bicarbonate, (SAB)]. Table 2 shows the variables and coefficients included in SAB model. Then we conducted the receiver operating characteristic (ROC) curves to show the prediction abilities of SAB model, SOFA and SAPSII on the clinical outcome in AA patients, which are shown in Figure 3. Among these three indicators, the SAB model had the best prediction ability on the clinical outcome. The AUC of SAB was 0.904 [95% confidence interval (CI): 0.841–0.967]. The second was SAPSII of which the AUC was 0.823 (95% CI: 0.752–0.893). The SOFA had the worse ability and its AUC was 0.776 (95% CI: 0.686–0.867). Then, we used the original data to evaluate the calibration of SAB model, SOFA and SAPSII. The results showed that the Brier score of SAB model was 0.043 (95% CI: 0.028–0.057), which was smaller than that of SAPSII (0.057, 95% CI: 0.040–0.073) and SOFA (0.056, 95% CI: 0.039–0.072), indicating more accuracy prediction ability of SAB model. Calibration curve of SAB model is shown in Figure 4. For internal validation, optimism bootstrap method with 1,000 repetitions was conducted. The adjusted AUC of SAB model was 0.898 (0.822 for SAPSII and 0.775 for SOFA) and the adjusted Brier scores was 0.045 (0.057 for SAPSII and 0.057 for SOFA). Other results of parameters of calibration curve in original cohort and results of internal validation are showed in Table 3. In order to evaluate the improvement of prediction ability of SAB model compared with SAPSII and SOFA, NRI (continuous) and IDI were used. Compared to SAPSII, the NRI (continuous) of SAB model was 0.695 (95% CI: 0.369–1.020; P<0.001) and IDI was 0.217(95% CI: 0.090–0.345; P<0.001), which had statistical significances. While comparing to SOFA, the NRI (continuous) of SAB model was 0.744 (95% CI: 0.418–1.069; P<0.001) and IDI was 0.229 (95% CI: 0.118–0.340; P<0.001), which also had statistical significances. The results of NRI and IDI are showed in Table 4. The risk stratification ability of SAB model is shown in Table 5. It was shown that SAB model could stratified the high-risk group. The death rate was 66.7% in 40–60% group, 83.3% in 60–80% group and 62.5% in 80–100% group. If a patient’s predicted probability is higher than 40%, he/she should be paid more attention in clinic. To show the clinical usefulness of SAB model, the DCA (Figure 5) was built based on the continuum of potential thresholds of ICU-death (x axis) and the net benefit of using SAB model to risk stratify patients (y axis) relative to assuming that no AA patient will die in ICU. The curve of SAB model showed that SAB model provided a net benefit across the range of ICU-death compared with the positive group in which every patient underwent surgery. Finally, a website based on this model is showed in Figure 6. If the clinical doctor input the patients’ data of sepsis, anion gapmaximum and bicarbonateminimum, the website will calculate the ICU-mortality and show the results immediately.
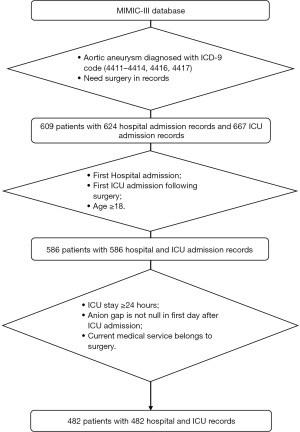
Table 1
Candidate variables | ICU-survival group (N=449) | ICU-death group (N=33) | P value |
---|---|---|---|
General condition | |||
Admission type | <0.001*** | ||
Elective | 321 (71.5%) | 10 (30.3%) | |
Emergency | 119 (26.5%) | 23 (69.7%) | |
Urgent | 9 (2.0%) | 0 (0.0%) | |
LOS of hospital | 8.83 (6.24, 14.15) | 9.92 (4.04, 17.61) | 0.763 |
LOS of ICU | 3.02 (1.83, 6.33) | 10.04 (2.59, 17.92) | <0.001*** |
In-hospital death | 3 (0.7%) | 33 (100.0%) | <0.001*** |
Type of aortic aneurysm | <0.001*** | ||
Abdominal aneurysm without mention of rupture | 124 (27.6%) | 10 (30.3%) | |
Abdominal aneurysm, ruptured | 38 (8.5%) | 12 (36.4%) | |
Thoracic aneurysm without mention of rupture | 238 (53.0%) | 5 (15.2%) | |
Thoracic aneurysm, ruptured | 7 (1.6%) | 2 (6.1%) | |
Thoracoabdominal aneurysm, ruptured | 9 (2.0%) | 2 (6.1%) | |
Thoracoabdominal aneurysm, without mention of rupture | 33 (7.3%) | 2 (6.1%) | |
Rupture of aortic aneurysm | 54 (12.0%) | 16 (48.5%) | <0.001*** |
Age (years) | 69.89 (60.42, 77.18) | 77.26 (70.76, 82.71) | <0.001*** |
Male | 289 (64.4%) | 15 (45.5%) | 0.047* |
Comorbidity | |||
Sepsis | 6 (1.3%) | 7 (21.2%) | <0.001*** |
Chronic pulmonary diseases | 105 (23.4%) | 8 (24.2%) | 1 |
Coagulopathy disorders | 73 (16.3%) | 12 (36.4%) | 0.007** |
Congestive heart failure | 9 (2.0%) | 1 (3.0%) | 1 |
Renal failure | 43 (9.6%) | 6 (18.2%) | 0.2 |
Fluid and electrolyte disorders | 96 (21.4%) | 9 (27.3%) | 0.567 |
Vital signs on admission | |||
Heart rate, mean (bpm) | 81.08 (73.55, 87.67) | 89.28 (79.36, 93.31) | 0.001** |
Systolic blood pressure, mean (mmHg) | 111.83 (106.55, 120.72) | 106.77 (103.78, 123.19) | 0.045* |
Diastolic blood pressure, mean (mmHg) | 57.09 (52.52, 61.98) | 59.56 (55.40, 64.51) | 0.09 |
Respirate rate, mean (1/min) | 16.45 (14.98, 18.40) | 18.61 (16.38, 20.14) | 0.002** |
SpO2, mean (%) | 98.04 (97.01, 98.94) | 97.53 (96.33, 98.35) | 0.01* |
Laboratory indicators | |||
Anion gap, maximum (mEq/L) | 12.00 (11.00, 14.00) | 17.00 (16.00, 22.00) | <0.001*** |
Anion gap, minimum (mEq/L) | 11.00 (9.00, 12.00) | 13.00 (12.00, 16.00) | <0.001*** |
Bicarbonate, maximum (mEq/L) | 25.00 (23.00, 27.00) | 22.00 (20.00, 25.00) | <0.001*** |
Bicarbonate, minimum (mEq/L) | 23.00 (21.00, 25.00) | 17.00 (15.00, 21.00) | <0.001*** |
Creatinine, maximum (mg/dL) | 1.00 (0.80, 1.30) | 1.80 (1.20, 2.10) | <0.001*** |
Creatinine, minimum (mg/dL) | 0.90 (0.70, 1.10) | 1.10 (0.80, 1.40) | 0.001** |
Blood urea nitrogen, maximum (mg/dL) | 17.00 (14.00, 22.00) | 27.00 (21.00, 35.00) | <0.001*** |
Blood urea nitrogen, minimum (mg/dL) | 15.00 (12.00, 19.00) | 18.00 (16.00, 29.00) | <0.001*** |
PTT, maximum (sec) | 40.70 (33.60, 51.70) | 64.80 (38.80, 113.00) | 0.001** |
PTT, minimum (sec) | 31.20 (28.00, 35.80) | 31.30 (25.10, 36.80) | 0.355 |
INR, maximum | 1.40 (1.30, 1.70) | 1.70 (1.30, 2.32) | 0.026* |
INR, minimum | 1.20 (1.10, 1.30) | 1.20 (1.08, 1.30) | 0.056 |
PT, maximum (sec) | 15.60 (14.40, 17.25) | 16.90 (14.57, 19.40) | 0.07 |
PT, minimum (sec) | 13.70 (13.10, 14.60) | 13.40 (12.47, 14.00) | 0.021* |
White blood cell count, maximum (K/ìL) | 12.40 (9.70, 15.50) | 14.60 (10.30, 17.60) | 0.034* |
White blood cell count, minimum (K/ìL) | 9.00 (6.80, 11.40) | 9.60 (5.30, 11.40) | 0.863 |
Platelet count, maximum (K/ìL) | 174.50 (136.00, 219.00) | 174.00 (138.00, 218.00) | 0.828 |
Platelet count, minimum (K/ìL) | 126.00 (94.00, 161.25) | 86.00 (61.00, 126.00) | <0.001*** |
Surgery-related indications | |||
Way of surgery | 0.79 | ||
Endovascular | 59 (13.1%) | 3 (9.1%) | |
Mixed | 15 (3.3%) | 1 (3.0%) | |
Open | 375 (83.5%) | 29 (87.9%) | |
Extracorporeal circulation used | 245 (54.6%) | 8 (24.2%) | 0.001** |
Bypass surgery used | 26 (5.8%) | 2 (6.1%) | 1 |
Ventilation used in first day of ICU | 408 (90.9%) | 29 (87.9%) | 0.795 |
Urine output in first day of ICU (mL) | 1,945.00 (1,250.00, 2,850.00) | 895.00 (311.00, 1,397.00) | <0.001*** |
Severity score | |||
GCS | 15.00 (14.00, 15.00) | 15.00 (15.00, 15.00) | 0.185 |
SAPSII | 34.00 (27.00, 43.00) | 51.00 (44.00, 66.00) | <0.001*** |
SOFA | 5.00 (3.00, 7.00) | 9.00 (7.00, 12.00) | <0.001*** |
0.6% of patients had unknown value for heart rate (mean); 1.0% for systolic blood pressure (mean) and diastolic blood pressure (mean); 0.6% for respirate rate (mean); 0.6% for SpO2 (mean); 0.2% for platelet count; 2.1% for PTT; 2.3% for PT; 2.3% for INR; 0.4% for white blood cell count; 1.7% for urine output on first day; 0.6% for GCS score. *, P<0.05; **, P<0.01; ***, P<0.001. AA, aortic aneurysm; ICU, intensive care unit; LOS, length of stay; SpO2, percutaneous oxygen saturation; PTT, partial thromboplastin time; INR, international standard ratio; PT, prothrombin time; GCS, Glasgow Coma Scale; SAPSII, simplified acute physiology score II; SOFA, sequential organ failure assessment.
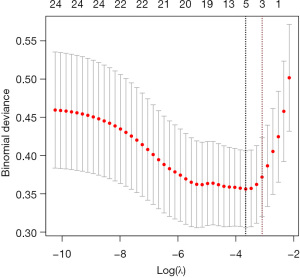
Table 2
Variables | Estimate | Standard error | Z value | P value |
---|---|---|---|---|
Intercept | −3.157 | 2.390 | −1.321 | 0.187 |
Sepsis | 2.385 | 0.733 | 3.253 | 0.001** |
Anion gap, maximum | 0.248 | 0.065 | 3.790 | <0.001*** |
Bicarbonate, minimum | −0.166 | 0.079 | −2.099 | 0.036* |
*, P<0.05; **, P<0.01; ***, P<0.001. SAB, Sepsis, Anion gap, Bicarbonate.
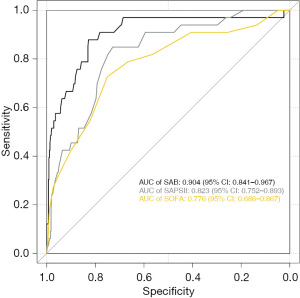
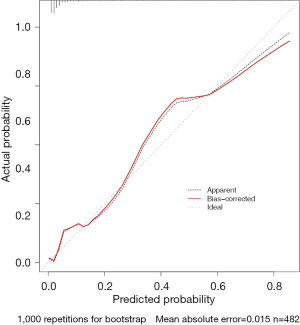
Table 3
Evaluation index | SAB model | SAPSII | SOFA |
---|---|---|---|
Discrimination | |||
AUC (95% CI) | 0.904 (0.841–0.967) | 0.823 (0.752–0.893) | 0.776 (0.686–0.867) |
Adjusted AUC† | 0.898 | 0.822 | 0.775 |
Calibration | |||
Brier score (95% CI) | 0.043 (0.028–0.057) | 0.057 (0.040–0.073) | 0.056 (0.039–0.072) |
Adjusted brier score† | 0.045 | 0.057 | 0.057 |
Intercept (95% CI) | 4.914E-09 (−1.628E-07 to 1.332E-08) | −1.018E-10 (−6.683E-08 to 1.159E-08) | 5.859E-12 (−6.422E-08 to 3.814E-08) |
Adjusted intercept† | −0.081 | 0.035 | 0.061 |
Slope (95% CI) | 1.000 (1.000–1.000) | 1.000 (1.000–1.000) | 1.000 (1.000–1.000) |
Adjusted slope† | 0.953 | 1.008 | 1.018 |
†, corrected indexes were calculated with optimism bootstrap method with 1,000 repetitions. SAB, Sepsis, Anion gap, Bicarbonate; AUC, area under the curve; CI, confidence interval; SAPSII, simplified acute physiology score II; SOFA, sequential organ failure assessment.
Table 4
Evaluation items | Compared to SAPSII | P value | Compared to SOFA | P value |
---|---|---|---|---|
NRI (continuous) (95% CI) | 0.695 (0.369–1.020) | <0.001*** | 0.744 (0.418–1.069) | <0.001*** |
IDI (95% CI) | 0.217 (0.090–0.345) | <0.001*** | 0.229 (0.118–0.340) | <0.001*** |
***, P<0.001. SAB, Sepsis, Anion gap, Bicarbonate; SAPSII, simplified acute physiology score II; SOFA, sequential organ failure assessment; NRI, net reclassification improvement; IDI, integrated discrimination improvement; CI, confidence interval.
Table 5
Prediction result interval of SAB model | 0–20% | 20–40% | 40–60% | 60–80% | 80–100% |
---|---|---|---|---|---|
Number | 445 | 17 | 6 | 6 | 8 |
In-ICU death | 14 (3.1%) | 5 (29.4%) | 4 (66.7%) | 5 (83.3%) | 5 (62.5%) |
In-hospital death | 17 (3.8%) | 5 (29.4%) | 4 (66.7%) | 5 (83.3%) | 5 (62.5%) |
SAB, Sepsis, Anion gap, Bicarbonate; ICU, intensive care unit.
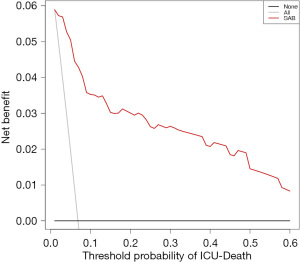
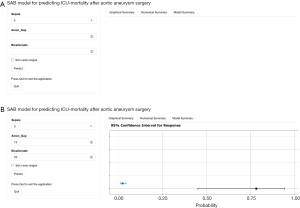
Discussion
This research used the recorded data in MIMIC-III to develop SAB model by using logistic regression with LASSO. SAB model was proved to have good prediction ability for ICU-death of AA patients (AUC higher than 0.8 in the original data and after internal validation), good calibration (Brier score was 0.043 in original data and 0.045 after internal validation), improvement in prediction ability compared to SOFA and SAPSII scores [both NRI (continuous) >0; both IDI >0; both P<0.05]. This research also validated better clinical usefulness across the range of ICU-death. This model only included 3 variables, which made it easy to obtain data. Besides, the website based on this model made it easy for clinical doctors in ICU to make rapid decision.
AA is a disease about pathological dilation of aorta. Different types of AA have different mortality. For AAA, it ranges from 2 to 10 percent (1,2); for TAAA, it ranges from 5 to 20 percent (4); while for TAA, it ranges from 5 to 11 percent (6). If AA ruptured, the 30-day mortality might rise over 30 percent (17,18) and the prognosis of aortic rupture is quite bad. For the patients who were more than 80 years old, more than 60% of EVAR patients sustained a complication (19). Renal failure is one of the most life-threatening complications. After receiving an urgent ruptured EVAR, the incidence rate of postoperative acute kidney injury (AKI) was more than 70% (20) and most occurred on the first day of ICU admission. In addition to renal failure, graft rupture is a common complication after repair surgery. According to EUROSTAR registry, the presence of graft migration, type 1 and type 3 endoleaks, and graft kink indicates graft rupture. Once the graft ruptures, the patient will bleed internally, which is life-threatening (8,9). Open repair is more dangerous than EVAR since the prognosis is worse. Most patients underwent open AAA repair had systemic inflammatory response syndrome (SIRS) (21). If these patients occurred infection, SIRS may develop into sepsis, and even worse when patients developed systemic hypoperfusion (22). Besides, there was a 3.8%, 38% and 64% incidence of multiorgan failure in the elective open AAA repair group, the urgent open AAA repair group and the ruptured AAA group (21). 5% of AA patients with multiorgan failure died before hospital discharge, which had higher incidence than the patients without organ dysfunction (23). The ICU-mortality of AAA patients was 2.36%, while TAA patients had the incidence of 9.43% (24). When patients are in unstable situation after surgery, surgeons will routinely transfer them to ICU. Therefore, it’s important for doctors to evaluate the risk of mortality of AA patient in ICU in order to make a more suitable treatment plan. A scoring system such as SOFA and SAPSII can help doctors make the best decision. This study showed that both SOFA, SAPSII and SAB model had the prediction ability of mortality of AA patient in ICU, but SAB had the best discrimination (AUC =0.904, 95% CI: 0.841–0.967). Both SAPSII and SOFA scores require more than 10 variables, which makes it inconvenient to use. While SAB model only requires 3 indicators. What’s more, the website has the advantages of visualization and quickness for calculate. In summary, we recommend that clinical doctors refer to our website to quickly assess the mortality of AA patients in ICU.
SAB model includes sepsis, the maximum of anion gap and the minimum of bicarbonate. Sometimes the treatment of infection is insufficient, which may lead to organ dysfunction or sepsis (25,26). Sepsis significantly increases the length of hospital stay and mortality in ICU patients. The reason for the significant increase in mortality due to sepsis is circulatory and cellular metabolic abnormalities (27). The mortality is related to the occurrence of septic shock. The mortality of septic shock was 27.9%, while those with non-septic shock was 16.0% (28). During follow-up or hospitalization, some AA patients died of sepsis (29,30). Because the AA repair surgery needs to create an opening in the blood vessel, this will lead to an increase in the rate of thrombosis. Besides, many studies prove that there is a strong relationship between sepsis and thrombosis, which significantly increases mortality (31).
Laboratory indicators can help doctors better understand the state of patients and help to accurately judge the prognosis. SAB model includes two indicators: the maximum of anion gap and the minimum of bicarbonate. Both the anion gap and bicarbonate in the serum is useful in the interpretation of acid-base disorders. This study proves that the maximum of anion gap has a positive correlation with ICU mortality. According to a multivariate analysis, the risk of ICU mortality may increase by 38% per 1 mEq/L increase in serum anion gap at hospital admission (32). Many patients underwent selective EVAR have AKI which has close relationship with mortality, and high dose of bicarbonate is proved to be an important preventive method (33,34). After AAA repair, bicarbonate can be used as a free radical scavenger and reduce renal tubular ischemia, which reduces the incidence of AKI (35,36).
Our study has several strengths. Firstly, we used machine learning LASSO to simplify the model and ensure the predictive ability. SAB model derived in this study only needs three variables, which is more convenient and quicker to use than SOFA and SAPSII. Secondly, SAB model is tested to have better prediction ability in both original data and internal validation. The discrimination and calibration of SAB model are better than the other two scoring systems (SAPSII and SOFA). Thirdly, we used DCA to test whether this model had clinical usefulness, and the result was quite good. Therefore, SAB model is convenient and has clinical application.
However, research also has limitations. Firstly, this study design was a retrospective study and it can’t include more sensitivity or specialist-recommending factor for predicting mortality. And the clinical outcome was set as all-cause mortality other than death directly related to AA, other complications associated with AA or non-return to independent living because we couldn’t have access to the patient’s clinical end point and do further follow up due to the limitation of database. Secondly, our study lacked data of blood gas analysis because there was too much lacking data for them, which may damage the sake and accuracy of the model. As a result, we didn’t include data of blood gas analysis though there were many variables that had value to predict the outcome. Thirdly, due to the limitation of the database, we couldn’t include preoperative variables which we thought can make our model more diversified and had more predictive power. Fourthly, we only included AA patients from single center, which needs more external validation.
Conclusions
We developed SAB model for in-ICU mortality of AA after surgery using the data from MIMIC-III database. SAB model had better predictive ability than SOFA and SAPSII. Clinical doctors can use our website to predict the mortality rate and choose a more suitable treatment plan.
Acknowledgments
Funding: This work was supported by the National Nature Science Foundation of China (No. 81800420).
Footnote
Reporting Checklist: The authors have completed the TRIPOD reporting checklist. Available at https://dx.doi.org/10.21037/apm-21-1660
Conflicts of Interest: All authors have completed the ICMJE uniform disclosure form (available at https://dx.doi.org/10.21037/apm-21-1660). The authors have no conflicts of interest to declare.
Ethical Statement: The authors are accountable for all aspects of the work in ensuring that questions related to the accuracy or integrity of any part of the work are appropriately investigated and resolved. This study was conducted in accordance with the Declaration of Helsinki (as revised in 2013).
Open Access Statement: This is an Open Access article distributed in accordance with the Creative Commons Attribution-NonCommercial-NoDerivs 4.0 International License (CC BY-NC-ND 4.0), which permits the non-commercial replication and distribution of the article with the strict proviso that no changes or edits are made and the original work is properly cited (including links to both the formal publication through the relevant DOI and the license). See: https://creativecommons.org/licenses/by-nc-nd/4.0/.
References
- Kabbani LS, Escobar GA, Knipp B, et al. APACHE III score on ICU admission predicts hospital mortality after open thoracoabdominal and open abdominal aortic aneurysm repair. Ann Vasc Surg 2010;24:1060-7. [Crossref] [PubMed]
- Thomas D, Anderson D, Hulten E, et al. Open versus endovascular repair of abdominal aortic aneurysm: Incidence of cardiovascular events in 632 patients in a department of defense cohort over 6-year follow-up. Vascular 2015;23:234-9. [Crossref] [PubMed]
- Gombert A, Barbati M, Kotelis D, et al. In-hospital mortality and organ failure after open and endovascular thoraco-abdominal aortic surgery can be predicted by increased levels of circulating dipeptidyl peptidase 3. Eur J Cardiothorac Surg 2021;59:750-7. [Crossref] [PubMed]
- Schepens MA, Dekker E, Hamerlijnck RP, et al. Survival and aortic events after graft replacement for thoracoabdominal aortic aneurysm. Cardiovasc Surg 1996;4:713-9. [Crossref] [PubMed]
- McClure RS, Brogly SB, Lajkosz K, et al. Epidemiology and management of thoracic aortic dissections and thoracic aortic aneurysms in Ontario, Canada: A population-based study. J Thorac Cardiovasc Surg 2018;155:2254-64.e4. [Crossref] [PubMed]
- Karimi A, McCord MR, Beaver TM, et al. Operative and Mid-Term Outcomes of Thoracic Aortic Operation in Octogenarians and Beyond. J Card Surg 2016;31:334-40. [Crossref] [PubMed]
- Lederle FA, Kyriakides TC, Stroupe KT, et al. Open versus Endovascular Repair of Abdominal Aortic Aneurysm. N Engl J Med 2019;380:2126-35. [Crossref] [PubMed]
- Brown LC, Greenhalgh RM, Powell JT, et al. Use of baseline factors to predict complications and reinterventions after endovascular repair of abdominal aortic aneurysm. Br J Surg 2010;97:1207-17. [Crossref] [PubMed]
- Harris PL, Vallabhaneni SR, Desgranges P, et al. Incidence and risk factors of late rupture, conversion, and death after endovascular repair of infrarenal aortic aneurysms: the EUROSTAR experience. European Collaborators on Stent/graft techniques for aortic aneurysm repair. J Vasc Surg 2000;32:739-49. [Crossref] [PubMed]
- EVAR trial participants. Endovascular aneurysm repair versus open repair in patients with abdominal aortic aneurysm (EVAR trial 1): randomised controlled trial. Lancet 2005;365:2179-86. [Crossref] [PubMed]
- Powell JT, Wanhainen A. Analysis of the Differences Between the ESVS 2019 and NICE 2020 Guidelines for Abdominal Aortic Aneurysm. Eur J Vasc Endovasc Surg 2020;60:7-15. [Crossref] [PubMed]
- Erbel R, Aboyans V, Boileau C, et al. 2014 ESC Guidelines on the diagnosis and treatment of aortic diseases: Document covering acute and chronic aortic diseases of the thoracic and abdominal aorta of the adult. The Task Force for the Diagnosis and Treatment of Aortic Diseases of the European Society of Cardiology (ESC). Eur Heart J 2014;35:2873-926. [Crossref] [PubMed]
- Chen Z, Ma Y, Li Q, et al. The application of thromboelastography in risk stratification for selective thromboembolism prophylaxis after total joint arthroplasty in Chinese: a randomized controlled trial. Ann Palliat Med 2020;9:2498-507. [Crossref] [PubMed]
- Hicks CW, Alshaikh HN, Zarkowsky D, et al. Intensive care unit admission after endovascular aortic aneurysm repair is primarily determined by hospital factors, adds significant cost, and is often unnecessary. J Vasc Surg 2018;67:1091-1101.e4. [Crossref] [PubMed]
- Krenzien F, Matia I, Wiltberger G, et al. Early prediction of survival after open surgical repair of ruptured abdominal aortic aneurysms. BMC Surg 2014;14:92. [Crossref] [PubMed]
- Johnson AE, Pollard TJ, Shen L, et al. MIMIC-III, a freely accessible critical care database. Sci Data 2016;3:160035 [Crossref] [PubMed]
- Pires Coelho A, Lobo M, Brandão JP, et al. Prediction of Survival after 48 Hours of Intensive Unit Care following Repair of Ruptured Abdominal Aortic Aneurysm-Multicentric Study for External Validation of a New Prediction Score for 30-Day Mortality. Ann Vasc Surg 2019;60:95-102. [Crossref] [PubMed]
- Healey CT, Neilson M, Clark D, et al. Predicting Mortality of Ruptured Abdominal Aortic Aneurysms in the Era of Endovascular Repair. Ann Vasc Surg 2017;38:59-63. [Crossref] [PubMed]
- Raju S, Eisenberg N, Montbriand J, et al. Endovascular repair of abdominal aortic aneurysm in octogenarians: clinical outcomes and complications. Can J Surg 2020;63:E329-37. [Crossref] [PubMed]
- Kopolovic I, Simmonds K, Duggan S, et al. Risk factors and outcomes associated with acute kidney injury following ruptured abdominal aortic aneurysm. BMC Nephrol 2013;14:99. [Crossref] [PubMed]
- Bown MJ, Nicholson ML, Bell PR, et al. The systemic inflammatory response syndrome, organ failure, and mortality after abdominal aortic aneurysm repair. J Vasc Surg 2003;37:600-6. [Crossref] [PubMed]
- Rodríguez-Gaspar M, Santolaria F, Jarque-López A, et al. Prognostic value of cytokines in SIRS general medical patients. Cytokine 2001;15:232-6. [Crossref] [PubMed]
- Bisgaard J, Gilsaa T, Rønholm E, et al. Aortic aneurysm disease vs. aortic occlusive disease: differences in outcome and intensive care resource utilisation after elective surgery: an observational study. Eur J Anaesthesiol 2013;30:65-72. [Crossref] [PubMed]
- Chen Q, Chen Q, Ye Y, et al. Characteristics and Prognosis of Abdominal or Thoracic Aortic Aneurysm Patients Admitted to Intensive Care Units After Surgical Treatment: A Multicenter Retrospective Observational Study. Int J Gen Med 2021;14:475-86. [Crossref] [PubMed]
- Rello J, Valenzuela-Sánchez F, Ruiz-Rodriguez M, et al. Sepsis: A Review of Advances in Management. Adv Ther 2017;34:2393-411. [Crossref] [PubMed]
- Shen R, Gao M, Tao Y, et al. Prognostic nomogram for 30-day mortality of deep vein thrombosis patients in intensive care unit. BMC Cardiovasc Disord 2021;21:11. [Crossref] [PubMed]
- Singer M, Deutschman CS, Seymour CW, et al. The Third International Consensus Definitions for Sepsis and Septic Shock (Sepsis-3). JAMA 2016;315:801-10. [Crossref] [PubMed]
- Abe T, Ogura H, Shiraishi A, et al. Characteristics, management, and in-hospital mortality among patients with severe sepsis in intensive care units in Japan: the FORECAST study. Crit Care 2018;22:322. [Crossref] [PubMed]
- Becquemin JP, Lapie V, Favre JP, et al. Mid-term results of a second generation bifurcated endovascular graft for abdominal aortic aneurysm repair: the French Vanguard trial. J Vasc Surg 1999;30:209-18. [Crossref] [PubMed]
- Carpenter JP, Anderson WN, Brewster DC, et al. Multicenter pivotal trial results of the Lifepath System for endovascular aortic aneurysm repair. J Vasc Surg 2004;39:34-43. [Crossref] [PubMed]
- Iba T, Levy JH. Inflammation and thrombosis: roles of neutrophils, platelets and endothelial cells and their interactions in thrombus formation during sepsis. J Thromb Haemost 2018;16:231-41. [Crossref] [PubMed]
- Chen Q, Chen Q, Li L, et al. Serum anion gap on admission predicts intensive care unit mortality in patients with aortic aneurysm. Exp Ther Med 2018;16:1766-77. [Crossref] [PubMed]
- Saratzis A, Chiocchia V, Jiffry A, et al. HYDration and Bicarbonate to Prevent Acute Renal Injury After Endovascular Aneurysm Repair With Suprarenal Fixation: Pilot/Feasibility Randomised Controlled Study (HYDRA Pilot Trial). Eur J Vasc Endovasc Surg 2018;55:648-56. [Crossref] [PubMed]
- Brulotte V, Leblond FA, Elkouri S, et al. Bicarbonates for the prevention of postoperative renal failure in endovascular aortic aneurysm repair: a randomized pilot trial. Anesthesiol Res Pract 2013;2013:467326 [Crossref] [PubMed]
- Merten GJ, Burgess WP, Rittase RA, et al. Prevention of contrast-induced nephropathy with sodium bicarbonate: an evidence-based protocol. Crit Pathw Cardiol 2004;3:138-43. [Crossref] [PubMed]
- Wong PC, Li Z, Guo J, et al. Pathophysiology of contrast-induced nephropathy. Int J Cardiol 2012;158:186-92. [Crossref] [PubMed]