Body fat percentage in adolescents is a better predictor of exercise effect on adiposity changes: a 4-year follow-up study
Introduction
The prevalence of obesity has reached an epidemic level in Western countries and is increasing in developing countries. In Asia, the prevalence of obesity has been increasing at an alarming rate in recent years, especially in China, Japan, and India (1). In 1975, the obese population in China was less than 0.1 million, which increased to 43.2 million by 2014, accounting for 16.3% of the obese population worldwide (2). Obesity in children and adolescents seems to be a major problem for the rich in low- and middle-income countries. Compared with young people of normal weight, the risk of obesity in adulthood is approximately five times higher among boys and nine times higher among girls who were obese at a young age (3). In addition, emerging evidence shows that obesity in children and adolescents is positively correlated with adult weight gain. This is problematic, as obesity in young people has been shown to increase the likelihood of overweight and obesity in adults (4), thereby increasing the risk of premature all-cause mortality (5). The increasing prevalence of obesity also increases the risk of many diseases, such as degenerative joint disease (6), type 2 diabetes (7), cardiovascular disease, and obesity-associated malignancies (8). Furthermore, obese adults who were also obese during adolescence have a higher chance of developing complications compared to those who became obese during adulthood but were of normal weight during adolescence (9,10). Without intervention, these comorbidities and obesity itself usually last into adulthood, which can lead to premature death (10,11). Given that the constellation of cardiac metabolic risk factors is considered to be changeable, the early identification and intervention for adolescents with metabolic syndrome is an important public health debate, in order to improve their status.
Exercise is a non-drug intervention, which can be used by the vast majority of the public, and may play a key role in the treatment of adolescent obesity (12,13). Body mass index (BMI) is still the most commonly used evaluation index, which can be easily identified and interpreted by practitioners. Some observational studies have shown that, relatively speaking, people who engage in more physical activity have a lower BMI than those who are less active (14,15). Unfortunately, other studies have shown that the effect of exercise on BMI is not significant. For example, except for a previous systematic review and meta-analysis focusing on exercise (16), previous studies showed that there was no significant change in the BMI of children and adolescents (17). Therefore, clarifying the influence of exercise on adolescents through other body fat indexes is crucial.
In view of the numerous factors related to obesity reported by previous studies, multiple confounding factors have not always been adjusted. Although most studies use BMI as the primary outcome, few large-scale epidemiological studies directly estimate obesity. A cross-sectional study showed that physical activity was negatively correlated with BMI and body fat percentage (BFP) in middle-aged people; for people with the same BMI, those who were more active had a lower BFP (18). Another study aimed to develop a multidisciplinary lifestyle intervention plan for 103 moderately and severely obese children and adolescents, and evaluate the additional effects of exercise intervention compared with routine care. After the intervention, the BMI z-score of the exercise group was 10% lower than the baseline score (P=0.03), however no interaction effects were observed between the groups. Meanwhile, significant intervention effects were observed in percentage of BF (%) (β=−1.52, 95% CI: −2.58 – −0.45) and lean body mass (LM) (β=1.20, 95% CI: 0.12–2.29) (19). A more comprehensive measurement method to check for obesity is appealing, in order to discover additional information beyond that provided by the BMI, especially among adolescents. Consequently, we conducted a prospective cohort study focused on the association between exercise and body mass and fat indexes, including BMI, BFP, and other indexes, in Shanghai freshmen, and aimed to identify sensitive indicators for potential intervention. We present the following article in accordance with the STROBE reporting checklist (available at https://dx.doi.org/10.21037/apm-21-1912).
Methods
Design and participants
This was a 4-year prospective follow-up study conducted in a multidisciplinary university in Shanghai, which recruited students nationwide. All freshmen in this university were enrolled in the first autumn semester of 2014. The purpose of this study was to describe and analyze the relationship between exercise and BMI, BFP, as well as other body mass and fat indexes in these college students. All students were enrolled in the study at baseline and were followed up every 2 years, so there were three visits altogether. Participants with abnormal medical examinations, including heart, liver and kidney dysfunctions, as well as those with missing visits, were excluded. Students recruited in this study came from 23 provinces and four municipalities in China, including the Han nationality and ethnic minorities. All procedures performed in this study involving human participants were in accordance with the Declaration of Helsinki (as revised in 2013). The study was approved by the Institutional Review Committee of Huashan Hospital, Fudan University (NO.: 2011-242) and informed consent was taken from all the participants.
Assessment of physical activity
The level of physical activity was evaluated by an effective questionnaire, and was updated every 2 years (20). The level of exercise was quantified synthetically according to the type, weekly frequency, and duration of physical activity. We estimated that the number of hours spent in moderate to intense activities (including brisk walking) per week requires at least three metabolic equivalent tasks (METs) per hour (21). We the classified high frequency and intensity exercise group as engaging moderate or vigorous exercise for at least 30 minutes daily (3.5 hours per week) (22).
Medical and anthropometric examination
Physical examinations were performed by experienced doctors in Huashan Hospital, and anthropometric evaluations were conducted by well-trained nurses in Huashan Hospital using standard methods in the school infirmary.
The students’ height was accurately evaluated to within 0.1 cm using a precision stadiometer (SECA 225, Hamburg, Germany), and weight was accurately measured to within by 0.1 kg using a calibrated digital scale (SECA 861, Hamburg, Germany). The subjects only wore light underwear without shoes. Waist and hip circumferences were also measured to the nearest 0.1 cm. Waist circumference (WC) was determined at the midline from the lower rib margin to the iliac crest, and hip circumference was determined at the most convex part of pubic symphysis and gluteus maximus. The waist-hip ratio (WHR) was calculated by dividing waist circumference by hip circumference. The percentage of body fat, fat mass, and fat-free mass were measured using the sectional bioelectrical impedance analysis (BIA) scale (Tanita, BC-418, Japan).
Blood pressure was measured by trained medical staff using mercury sphygmomanometers (model XJ11D, Shanghai Medical Equipment Co., Ltd., China), stethoscopes (model TZ-1, Shanghai Medical Equipment Co., Ltd., China), and appropriate cuffs. Participants were asked to sit quietly for at least 5 minutes prior to the first reading. Systolic blood pressure (SBP) was determined at the beginning of the first Korotkoff sound, and diastolic blood pressure (DBP) was measured at the fifth Korotkoff sound. Blood pressure was measured twice, with a 5-minute interval between the two measurements, and the average values were calculated.
Other data collection
Smoking and drinking histories, as well as dietary habits were collected from the subjects at baseline using the standard operation protocol. Information on smoking was obtained separately for cigarettes, pipes, and cigars. The exposure variables related to cigarette smoking included history of smoking (never, past, current) and pack-years of smoking. An “ever smoker” was defined as an individual who self-reported smoking at least 100 cigarettes during the course of his/her lifetime or smoked pipes or cigars for 6 months or more. A “current smoker” was defined as an individual who self-reported smoking within the calendar year prior to joining the study. A “past smoker” was defined as an ever smoker who had quit smoking for more than one calendar year before joining the study. Cigarette equivalents were calculated using the conversion factor 1 cigarette =1/2 pipe =1/4 cigar; the average number of cigarette equivalents smoked/day was defined categorically as 0, 1–19, 20–39, and 40+ (23,24).
The consumption of alcoholic beverages was obtained separately for beer, wine, and hard liquor. An “ever drinker” was defined as a person who self-reported consuming at least 12 drinks of any alcoholic beverage type over his/her lifetime. A “current drinker” was defined as a person who reported drinking any type of alcoholic beverage within the calendar year prior to joining the study. A “past drinker” was defined as an ever drinker who had discontinued all drinking for more than one calendar year before joining the study. The average number of drinks consumed per week was calculated based upon the reported number of 12-ounce beers, 4-ounce glasses of wine, and 1.5-ounce shots of hard liquor consumed per week. The frequency of alcohol consumption was defined categorically as 0, >0–<7, 7–20, and >20 drinks/week (25,26). The “Dietary Habits Scale” developed by Huang (27) was used to measure the dietary habits with Cronbach’s α of 0.85. There were seven good dietary habits (GDHs) on the scale; to measure if respondents consumed a good diet, we used the variable labeled “good diet”. The main item was to ask respondents to self-report how often they ate “vegetables” over a 1-week period, and the response format was as follows: 1= almost never, 2= less than once a week, 3= every week, 4= once a day, and 5 = more than once a day.
Blood sample examination
Blood samples were collected in 2014 and 2018. After 12-hours overnight fasting, venous blood samples (5 mL) were obtained from the anterior elbow vein of each participant, and collected into ethylene diamine tetraacetic acid (EDTA) vacuum tubes between 7:00 and 9:00 a.m. Samples were centrifuged at 3,000 rpm, divided equally, and stored at −80 °C. The levels of total cholesterol (TC), low-density lipoprotein cholesterol (LDL), high-density lipoprotein cholesterol (HDL), and triglycerides (TG) were measured in the clinical lab of Huashan Hospital; TC and TG levels were determined by enzyme methods, and LDL and HDL levels were measured using the clearance method.
Study size and biases
To calculate the sample size, we collected the information of a small sample of the students. For students engaging in low and high frequency and intensity exercise, the prevalence of BFP reduction was approximately 25% and 37%, respectively. Thus, we determined that at least 390 students should be recruited in each cohort.
A potential for recall bias exists whenever historical self-report information is elicited from respondents, and thus, recall bias must have existed in the self-reported exercise information in this study. However, it is one of the most common ways to collect information, especially in epidemiological research with large samples. It offers advantages such as practicality and low cost, and is quick and easy to administer. To address the potential sources of bias, we used the blind method to collect data, and all participants were given standardized training and explanation prior to completing the questionnaire. In the whole research process, there also was lost to follow-up bias. To address this, we aimed to improve the compliance of the participants through timely health assistance and patient explanation. If participants could not be reached through all means, all information would be eliminated when analyzing and reporting the lost visitors.
Statistical analyses
Data were presented as means ± standard deviation (SD) for normally distributed continuous variables, and as medians with interquartile range (IQR) for non-normally distributed continuous variables. Categorical variables were presented as frequencies or percentages. The t-test or Chi-square test was used to evaluate the differences in general characteristics at baseline according to physical activity classification. An unadjusted model and a multivariate model were used to estimate the relationship between physical activity and body fat loss. Factors that exhibited significant differences in the univariate analysis were included in the multivariate analysis. The multivariate model used in this study was adjusted for age, sex, BMI, smoking, drinking, and eating. Since the variance inflation factor (VIF) was less than 10, there was no multicollinearity among the variables, which could be included in multivariate analysis. P<0.05 was considered to be statistically significant. Statistical analyses were carried out using the SPSS version 24.0 (SPSS Inc., Chicago, IL, USA).
Results
A total of 2,000 students were enrolled in the study at baseline. Fifty-nine participants were excluded because they had abnormal physical examinations, including dysfunctions of the heart, liver, and kidney, or missed visits. Among these participants, 17 were excluded due to abnormal medical examinations at baseline, 18 participants were lost to follow-up in 2016, and 24 participants were lost to follow-up in 2018. All information was eliminated when analyzing and reporting the lost visitors.
As shown in Table 1, 1,941 participants (average age, 17.93±0.72 years) were recruited in this study, including 735 (37.87%) males and 1,206 (62.13%) females. The mean BMI of the total population was 20.73±3.02 kg/m2. The mean waist circumference and WHR were 69.01±10.95 cm and 0.76±0.06, respectively. The mean BFP was 22.17%±7.84%. Blood pressure measurement showed that the average SBP was 114.60±12.96 mmHg, and the average DBP was 67.19±8.98 mmHg. The entire cohort was divided into a low frequency and intensity exercise group (N=1,483, 76.40%) and a high frequency and intensity exercise group (N=458, 23.60%) based on the evaluated exercise amount. Compared to the low frequency and intensity exercise group, the proportion of males (29.33% vs. 65.50%, P<0.0001), average weight (56.37±10.67 vs. 62.35±11.86 kg, P<0.0001), BMI (20.54±2.99 vs. 21.34±3.01 kg/m2, P<0.0001), waist circumference (68.52±9.88 vs. 72.08±9.52 cm, P<0.0001), WHR (0.76±0.06 vs. 0.78±0.06, P<0.0001), and SBP (113.29±12.32 vs. 118.93±14.03 mmHg, P<0.0001) were greater in the high frequency and intensity exercise group; however, BFP (22.26%±6.12% vs. 20.60%±6.87%, P<0.0001) and HDL-C (1.60±0.39 vs. 1.50±0.38 mmol/L, P<0.0001) were lower in this group.
Table 1
Characteristic | Overall study population (N=1,941) | Grouped by exercise frequency | ||
---|---|---|---|---|
Low frequency and intensity exercise group (N=1,483) | High frequency and intensity exercise group (N=458) | P value | ||
Age (years) | 17.93±0.72 | 17.92±0.74 | 17.98±0.67 | 0.1469 |
Male, n (%) | 735 (37.87) | 435 (29.33) | 300 (65.50) | <0.0001 |
Weight (kg) | 57.59±11.46 | 56.37±10.67 | 62.35±11.86 | <0.0001 |
BMI (kg/m2) | 20.73±3.02 | 20.54±2.99 | 21.34±3.01 | <0.0001 |
Waist circumference (cm) | 69.01±10.95 | 68.52±9.88 | 72.08±9.52 | <0.0001 |
WHR | 0.76±0.06 | 0.76±0.06 | 0.78±0.06 | <0.0001 |
Body fat percentage (%) | 22.17±7.84 | 22.26±6.12 | 20.60±6.87 | <0.0001 |
SBP (mmHg) | 114.60±12.96 | 113.29±12.32 | 118.93±14.03 | <0.0001 |
DBP (mmHg) | 67.19±8.98 | 67.06±8.64 | 67.84±10.02 | 0.1044 |
TG (mmol/L) | 0.77±0.33 | 0.77±0.32 | 0.78±0.37 | 0.444 |
Chol (mmol/L) | 4.15±0.73 | 4.17±0.74 | 4.10±0.70 | 0.0648 |
LDL-C (mmol/L) | 2.23±0.64 | 2.22±0.65 | 2.24±0.61 | 0.5929 |
HDL-C (mmol/L) | 1.58±0.39 | 1.60±0.39 | 1.50±0.38 | <0.0001 |
BMI, body mass index; WHR, waist-hip ratio; SBP, systolic blood pressure; DBP, diastolic blood pressure; TG, triglyceride; Chol, cholesterol; LDL-C, low-density lipoprotein cholesterol; HDL-C, high-density lipoprotein cholesterol.
All students enrolled in the study were followed up every 2 years in 2016 and 2018. As depicted in Table 2 and Figure 1, follow-up in 2016 and 2018 showed that, compared to the low frequency and intensity exercise group, the weight (2016: 57.45±9.81 vs. 63.18±11.85 kg; 2018: 57.90±11.03 vs. 63.82±12.65 kg), BMI (2016: 20.89±3.11 vs. 21.60±3.39 kg/m2; 2018: 20.92±3.08 vs. 21.76±3.28 kg/m2), waist circumference (2016: 70.25±7.96 vs. 73.49±8.94 cm; 2018: 72.55±9.36 vs. 76.69±10.16 cm), and WHR (2016: 0.76±0.06 vs. 0.78±0.06; 2018: 0.77±0.07 vs. 0.80±0.07) of the high frequency and intensity exercise group remained higher (all P<0.0001), while the BFP was significantly lower (2016: 23.62%±6.07% vs. 21.44%±6.14%; 2018: 24.89%±5.13% vs. 22.07%±5.93%; P<0.0001). In 2016 and 2018, the difference in the SBP between the two groups at baseline disappeared (2016: 115.01±13.98 vs. 116.20±14.60 mmHg; P=0.0999, 2018: 117.95±14.24 vs. 119.23±14.38 mmHg, P=0.1073). However, in 2018, the DBP of the high frequency and intensity exercise group was significantly higher than that of the low frequency group (69.72±9.29 vs. 71.47±9.48 mmHg, P=0.0015) (Table 2 and Figure 2). In terms of the serum lipid spectrum, the TG of the high frequency and intensity exercise group in 2018 was markedly lower than that of the low frequency and intensity exercise group (0.74±0.32 vs. 0.78±0.34 mmol/L, P=0.0237), and the difference in HDL-C at baseline disappeared (1.58±0.41 vs. 1.58±0.38 mmol/L, P=0.9217) (Table 2 and Figure 3).
Table 2
Characteristic | Low frequency and intensity exercise group (N=1,483) | High frequency and intensity exercise group (N=458) | P value |
---|---|---|---|
Weight (kg) | |||
2014 | 56.37±10.67 | 62.35±11.86 | <0.0001 |
2016 | 57.45±9.81 | 63.18±11.85 | <0.0001 |
2018 | 57.90±11.03 | 63.82±12.65 | <0.0001 |
BMI (kg/m2) | |||
2014 | 20.54±2.99 | 21.34±3.01 | <0.0001 |
2016 | 20.89±3.11 | 21.60±3.39 | <0.0001 |
2018 | 20.92±3.08 | 21.76±3.28 | <0.0001 |
Waist circumference (cm) | |||
2014 | 68.52±9.88 | 72.08±9.52 | <0.0001 |
2016 | 70.25±7.96 | 73.49±8.94 | <0.0001 |
2018 | 72.55±9.36 | 76.69±10.16 | <0.0001 |
WHR | |||
2014 | 0.76±0.06 | 0.78±0.06 | <0.0001 |
2016 | 0.76±0.06 | 0.78±0.06 | <0.0001 |
2018 | 0.77±0.07 | 0.80±0.07 | <0.0001 |
Body fat percentage (%) | |||
2014 | 22.26±6.12 | 20.60±6.87 | <0.0001 |
2016 | 23.62±6.07 | 21.44±6.14 | <0.0001 |
2018 | 24.89±5.13 | 22.07±5.93 | <0.0001 |
SBP (mmHg) | |||
2014 | 113.29±12.32 | 118.93±14.03 | <0.0001 |
2016 | 115.01±13.98 | 116.20±14.60 | 0.0999 |
2018 | 117.95±14.24 | 119.23±14.38 | 0.1073 |
DBP (mmHg) | |||
2014 | 67.06±8.64 | 67.84±10.02 | 0.1044 |
2016 | 69.29±9.80 | 68.78±10.38 | 0.3173 |
2018 | 69.72±9.29 | 71.47±9.48 | 0.0015 |
TG (mmol/L) | |||
2014 | 0.77±0.32 | 0.78±0.37 | 0.444 |
2018 | 0.78±0.34 | 0.74±0.32 | 0.0237 |
Chol (mmol/L) | |||
2014 | 4.17±0.74 | 4.10±0.70 | 0.0648 |
2018 | 4.16±0.73 | 4.14±0.72 | 0.5369 |
LDL-C (mmol/L) | |||
2014 | 2.22±0.65 | 2.24±0.61 | 0.5929 |
2018 | 2.22±0.65 | 2.22±0.61 | 0.9899 |
HDL-C (mmol/L) | |||
2014 | 1.60±0.39 | 1.50±0.38 | <0.0001 |
2018 | 1.58±0.38 | 1.58±0.41 | 0.9217 |
BMI, body mass index; WHR, waist-hip ratio; SBP, systolic blood pressure; DBP, diastolic blood pressure; TG, triglyceride; Chol, cholesterol; LDL-C, low-density lipoprotein cholesterol; HDL-C, high-density lipoprotein cholesterol.
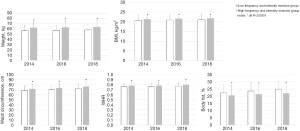
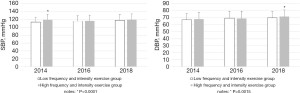
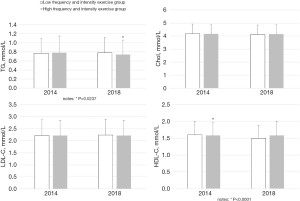
We further analyzed the changes of these body mass and fat indexes between the two groups from 2014 to 2018. As depicted in Table 3, there were no significant differences in change of weight, BMI, waist circumference, WHR and blood pressure between the two groups. However, the increase in BFP in the high frequency and intensity exercise group was notably lower than that in the low frequency and intensity exercise group [1.50 (−1.30–4.30) vs. 2.70 (−0.05–5.20), P=0.0289].
Table 3
Changes, median (interquartile range) | P value | ||
---|---|---|---|
Low frequency and intensity exercise group (N=1,483) | High frequency and intensity exercise group (N=458) | ||
Weight change (kg) | 2.00 (−1.00–4.00) | 2.00 (−1.00–5.00) | 0.1102 |
BMI change (kg/m2) | 0.38 (−0.56–1.21) | 0.40 (−0.47–1.32) | 0.9484 |
Waist circumference change (cm) | 4.00 (0.00–8.00) | 5.00 (1.00–8.00) | 0.0602 |
WHR change | 0.01 (−0.03–0.05) | 0.02 (−0.01–0.05) | 0.6581 |
Body fat change (%) | 2.70 (−0.05–5.20) | 1.50 (−1.30–4.30) | 0.0289 |
SBP change (mmHg) | 5.00 (−3.00–14.00) | 6.00 (−6.00–14.00) | 0.1010 |
DBP change (mmHg) | 3.00 (−3.00–10.00) | 4.00 (−2.00–11.00) | 0.5673 |
BMI, body mass index; WHR, waist-hip ratio; SBP, systolic blood pressure; DBP, diastolic blood pressure.
As detailed in Table 4, compared to the low frequency and intensity exercise group, the high frequency and intensity exercise group exhibited a higher likelihood of reducing BFP from 2014 to 2018 [odds ratio (OR) =1.68; 95% confidence interval (CI): 1.31–2.15). In fact, this association still existed even after adjusting for age, gender, BMI, as well as smoking, drinking, and dietary habits (OR =1.43; 95% CI: 1.09–1.86).
Table 4
OR (95% CI) | Adjusted factor | ||
---|---|---|---|
Low frequency and intensity exercise group (N=1,483) | High frequency and intensity exercise group (N=458) | ||
Model 1 | 1 | 1.68 (1.31–2.15) | Unadjusted |
Model 2 | 1 | 1.44 (1.10–1.87) | Age, gender |
Model 3 | 1 | 1.43 (1.09–1.86) | Age, gender, BMI, as well as smoking, drinking, and dietary habit |
OR, odds ratio.
In Table 5, we analyzed the changes between different genders in the high frequency and intensity exercise group. The increase in weight and waist circumference of female were lower than that of male, no difference in changes of BMI, WHR, body fat, SBP and DBP was found between the genders.
Table 5
Changes, median (interquartile range) | P value | ||
---|---|---|---|
Male (N=298) | Female (N=160) | ||
Weight change (kg) | 3.00 (0.00–6.00) | 1.00 (−1.00–3.00) | 0.0031 |
BMI change (kg/m2) | 0.67 (−0.33–1.66) | 0.12 (−0.70–0.82) | 0.1281 |
Waist circumference change (cm) | 6.00 (2.00–9.00) | 3.75 (−0.25–7.00) | 0.0161 |
WHR change | 0.03 (−0.00–0.06) | 0.00 (−0.03–0.04) | 0.1178 |
Body fat change (%) | 1.10 (−1.60–3.80) | 2.20 (−0.60–4.75) | 0.2452 |
SBP change (mmHg) | 6.00 (−6.00–14.00) | 5.00 (−5.00–15.00) | 0.6741 |
DBP change (mmHg) | 3.00 (−3.00–11.00) | 6.00 (0.00–11.00) | 0.8715 |
BMI, body mass index; WHR, waist-hip ratio; SBP, systolic blood pressure; DBP, diastolic blood pressure.
Discussion
In our research, high frequency and intensity exercise provided benefits in reducing BFP, and no other body mass or fat indexes showed any association. Our results also showed that most body mass and fat indexes exhibited an upward trend with age. SBP, TG, and HDL-C were also significantly improved in the high frequency and intensity exercise group.
The prevalence and severity of obesity have been increasing globally across different age groups, and have more than doubled since 1980. It has long been known that obesity is associated with numerous co-morbidities, including cardiovascular diseases, diabetes mellitus (7), musculoskeletal disorders (6), and several cancers (8). Given that the constellation of cardiac metabolic risk factors is considered to be changeable, early identification and intervention for adolescents with metabolic syndrome is an important public health debate so as to improve their status.
Adolescence is a crucial period for investing in health both in the present and into the future. Therefore, identifying areas to promote positive adolescent health behaviors and prevent negative behaviors is crucial. Although genetic factors play an important role in the pathogenesis of obesity, human behavior and lifestyle, such as physical activity (28) and eating habits (29), are also important factors in its clinical manifestations (30). In previous studies, BMI was often used to evaluate the effect of exercise on body weight. However, the effects of exercise on BMI have unfortunately been underwhelming. There have been few studies assessing the change in body composition, such as BFP. A previous cross-sectional analysis showed that individuals who were more active had a lower fat percentage than those with the same BMI as middle-aged people (18). Another recent study aimed to develop a multidisciplinary lifestyle intervention program for 103 moderately and severely obese children and adolescents, and evaluate the additional effects of exercise intervention compared with routine care. After the intervention, significant interaction effects were observed in the BFP, but no notable interaction effects were observed in the BMI z-score (19). These results suggest that body fat changes may be more sensitive than BMI in reflecting the effects of exercise. It is interesting to explore whether more comprehensive body fat measurement provides additional information beyond that captured by the BMI. Therefore, our group focused on the effects of exercise on the BMI, BFP, and other body mass and fat indicators in Shanghai university freshmen, and attempted to identify sensitive indicators for potential intervention. The freshmen recruited in this survey included various multi-ethnic and socio-economic diverse samples. As in our study, Chinese freshmen seem to be thinner than their corresponding partners in America and Europe, with a mean BMI 20.73±3.02 kg/m2 and BFP 22.17%±7.84% (31,32). At baseline, we found that the proportion of males in the high frequency and intensity exercise group was significantly higher than that in the low frequency and intensity exercise group (65.50% vs. 29.33%, P<0.0001), which was consistent with previous research (33). The body weight, BMI, waist circumference, WHR, and SBP of the high frequency and intensity exercise group were higher than those of the low frequency and intensity exercise group, which explained why they had a more urgent needs for exercise (34,35). However, the BFP in the high frequency and intensity exercise group was significantly lower than that in the low frequency and intensity exercise group (20.60%±6.87% vs. 22.26%±6.12%, P<0.0001). This may be due to the higher proportion of men in the high frequency and intensity exercise group, while the average BFP of men is significantly lower than that of women (36,37). Therefore, this 4-year follow-up observation was particularly important to assess the impact of exercise on body mass and fat indexes.
During the follow-up period, we observed that the body mass and fat indexes and blood pressure of both groups exhibited an upward trend with age. At the end of the follow-up period, the SBP, TG, and HDL-C in the high frequency and intensity exercise group were significantly improved compared to the baseline levels. The TG level of the high frequency and intensity exercise group was markedly lower than that in low frequency and intensity exercise group, and the differences in SBP and HDL-C between two groups at baseline disappeared at the follow-up visit. These results showed that, compared with low frequency and intensity exercise, high frequency and intensity exercise could provide benefits for controlling blood pressure (38,39) and serum lipids (40,41). Along with the growth of the age, the average level of most body mass and fat indexes was still higher in the high frequency and intensity exercise group in the follow-up visit. Thus, we further compared whether there were any differences in the changes of body mass and fat indexes from 2014 to 2018, and found that the increase in BFP was significantly lower in the high frequency and intensity exercise group, while changes in other body mass and fat indexes were not notably different. This suggested that BFP could more sensitively reflect the changes in human body mass and fat content.
In order to eliminate the influence of other interfering factors on the data analysis, we conducted logistic regression analysis. The results showed that high frequency and intensity exercise was an independent influencing factor for the decline of BFP, regardless of age, gender, BMI, as well as smoking, drinking, and dietary habits. Therefore, BFP is the most sensitive indicator to reflect the effect of exercise on adolescents.
This was a prospective cohort study based on real-world evidence (RWE). The strengths of our research include a large sample size, well-trained interviewers, and the valuable data pool. We collected the data of all freshmen in this multidisciplinary university, and described their body mass and fat indexes, blood pressure, and blood lipid characteristics. Although this study was conducted in Shanghai, the included college students came from 23 provinces and four municipalities of China, including the Han nationality and ethnic minorities, and thus, could be used as a rough representation of the national data to a certain extent. Adjustment for multiple confounding factors reduced the potential impact of variable statistics. Our results support the conclusion that the BFP is a more sensitive indicator to reflect the exercise effect in Chinese adolescents.
Limitations
There are also some limitations in our research that should be noted. Firstly, the use of self-reports for exercise habits, as opposed to motion equivalent, may lead to systematic bias, as previous studies have shown that exercise time by self-reports appear to be longer than objective measurements (42). However, other studies have shown a reasonably close agreement between self-reports and objective measurements of exercise activity (43,44). Secondly, not all essential parameters are included in our research, such as sedentary behavior (28) and mental state. In the future, more prospective studies are needed to clarify the impact of the above factors on youth obesity.
Conclusions
The medical, financial, and social consequences of adolescent obesity, which is a new epidemic, are enormous, and require an urgent response from health professionals and policy makers. Prevention seems to be a promising strategy among high-risk populations (45). More sensitive indicators reflecting body mass and fat changes could help us to observe the benefits of intervention factors on body shape at an early stage. To the best of our knowledge, prospective cohort studies investigating the characteristics of different body mass and fat indexes and their relationship with exercise in Chinese Mainland freshmen are rare. BFP seems to be a more sensitive indicator of body fat status among freshmen, even after controlling for multiple confounding factors. Therefore, we suggest that young people should pay more attention to BFP in order to better prevent and manage obesity. High frequency and intensity exercise should be encouraged to benefit public health. In future research, BFP, as a sensitive index, could be helpful in controlling obesity in adolescents.
Acknowledgments
We would like to thank all of the students who participated in this study.
Funding: We acknowledge financial support from the National Natural Science Foundation of China (81800691, 81670751, 81970711, 81770840), the National Key R&D Program of China (2016YFC1305105), and the Shanghai Municipal Commission of Health and Family Planning [20164Y0041, 20144Y0070, ZY(2018-2020)-FWTX-1002], China Postdoctoral Science Foundation (2021M690680).
Footnote
Reporting Checklist: The authors have completed the STROBE reporting checklist. Available at https://dx.doi.org/10.21037/apm-21-1912
Data Sharing Statement: Available at https://dx.doi.org/10.21037/apm-21-1912
Conflicts of Interest: All authors have completed the ICMJE uniform disclosure form (available at https://dx.doi.org/10.21037/apm-21-1912). The authors have no conflicts of interest to declare.
Ethical Statement: The authors are accountable for all aspects of the work in ensuring that questions related to the accuracy or integrity of any part of the work are appropriately investigated and resolved. All procedures performed in this study involving human participants were in accordance with the Declaration of Helsinki (as revised in 2013). The study was approved by the Institutional Review Committee of Huashan Hospital, Fudan University (NO.:2011-242) and informed consent was taken from all the participants.
Open Access Statement: This is an Open Access article distributed in accordance with the Creative Commons Attribution-NonCommercial-NoDerivs 4.0 International License (CC BY-NC-ND 4.0), which permits the non-commercial replication and distribution of the article with the strict proviso that no changes or edits are made and the original work is properly cited (including links to both the formal publication through the relevant DOI and the license). See: https://creativecommons.org/licenses/by-nc-nd/4.0/.
References
- Chakraborty C, Das S. Dynamics of Diabetes and Obesity: An Alarming Situation in the Developing Countries in Asia. Mini Rev Med Chem 2016;16:1258-68. [Crossref] [PubMed]
- Fan JG, Kim SU, Wong VW. New trends on obesity and NAFLD in Asia. J Hepatol 2017;67:862-73. [Crossref] [PubMed]
- Venn AJ, Thomson RJ, Schmidt MD, et al. Overweight and obesity from childhood to adulthood: a follow-up of participants in the 1985 Australian Schools Health and Fitness Survey. Med J Aust 2007;186:458-60. [Crossref] [PubMed]
- Singh AS, Mulder C, Twisk JW, et al. Tracking of childhood overweight into adulthood: a systematic review of the literature. Obes Rev 2008;9:474-88. [Crossref] [PubMed]
- Danaei G, Ding EL, Mozaffarian D, et al. The preventable causes of death in the United States: comparative risk assessment of dietary, lifestyle, and metabolic risk factors. PLoS Med 2009;6:e1000058 [Crossref] [PubMed]
- Thijssen E, van Caam A, van der Kraan PM. Obesity and osteoarthritis, more than just wear and tear: pivotal roles for inflamed adipose tissue and dyslipidaemia in obesity-induced osteoarthritis. Rheumatology (Oxford) 2015;54:588-600. [Crossref] [PubMed]
- Lee KW, Ching SM, Devaraj NK, et al. Genetic polymorphisms in neuroendocrine disorder-related candidate genes associated with pre-pregnancy obesity in gestational diabetes mellitus patients by using a stratification approach. Ann Transl Med 2020;8:1060. [Crossref] [PubMed]
- Qiang JK, Lipscombe LL, Lega IC. Association between diabetes, obesity, aging, and cancer: review of recent literature. Transl Cancer Res 2020;9:5743-59. [Crossref]
- GBD 2015 Obesity Collaborators; Afshin A, Forouzanfar MH, et al. Health Effects of Overweight and Obesity in 195 Countries over 25 Years. N Engl J Med 2017;377:13-27.
- Twig G, Yaniv G, Levine H, et al. Body-Mass Index in 2.3 Million Adolescents and Cardiovascular Death in Adulthood. N Engl J Med 2016;374:2430-40. [Crossref] [PubMed]
- Biro FM, Wien M. Childhood obesity and adult morbidities. Am J Clin Nutr 2010;91:1499S-505S. [Crossref] [PubMed]
- Lipnowski S, Leblanc CMCanadian Paediatric Society, Healthy Active Living and Sports Medicine Committee. Healthy active living: Physical activity guidelines for children and adolescents. Paediatr Child Health 2012;17:209-12. [Crossref] [PubMed]
- Kelley GA, Kelley KS, Pate RR. Effects of exercise on BMI z-score in overweight and obese children and adolescents: a systematic review with meta-analysis. BMC Pediatr 2014;14:225. [Crossref] [PubMed]
- Besson H, Ekelund U, Luan J, et al. A cross-sectional analysis of physical activity and obesity indicators in European participants of the EPIC-PANACEA study. Int J Obes (Lond) 2009;33:497-506. [Crossref] [PubMed]
- Orsini N, Bellocco R, Bottai M, et al. Correlates of total physical activity among middle-aged and elderly women. Int J Behav Nutr Phys Act 2007;4:16. [Crossref] [PubMed]
- Lavelle HV, Mackay DF, Pell JP. Systematic review and meta-analysis of school-based interventions to reduce body mass index. J Public Health (Oxf) 2012;34:360-9. [Crossref] [PubMed]
- Atlantis E, Barnes EH, Singh MA. Efficacy of exercise for treating overweight in children and adolescents: a systematic review. Int J Obes (Lond) 2006;30:1027-40. [Crossref] [PubMed]
- Bradbury KE, Guo W, Cairns BJ, et al. Association between physical activity and body fat percentage, with adjustment for BMI: a large cross-sectional analysis of UK Biobank. BMJ Open 2017;7:e011843 [Crossref] [PubMed]
- Seo YG, Lim H, Kim Y, et al. The effect of a multidisciplinary lifestyle intervention on obesity status, body composition, physical fitness, and cardiometabolic risk markers in children and adolescents with obesity. Nutrients 2019;11:137. [Crossref] [PubMed]
- Wolf AM, Hunter DJ, Colditz GA, et al. Reproducibility and validity of a self-administered physical activity questionnaire. Int J Epidemiol 1994;23:991-9. [Crossref] [PubMed]
- Ainsworth BE, Haskell WL, Whitt MC, et al. Compendium of physical activities: an update of activity codes and MET intensities. Med Sci Sports Exerc 2000;32:S498-504. [Crossref] [PubMed]
- Li Y, Schoufour J, Wang DD, et al. Healthy lifestyle and life expectancy free of cancer, cardiovascular disease, and type 2 diabetes: prospective cohort study. BMJ 2020;368:l6669. [Crossref] [PubMed]
- Morse DE, Psoter WJ, Cleveland D, et al. Smoking and drinking in relation to oral cancer and oral epithelial dysplasia. Cancer Causes Control 2007;18:919-29. [Crossref] [PubMed]
- Morse DE, Psoter WJ, Baek LS, et al. Smoking and drinking in relation to depressive symptoms among persons with oral cancer or oral epithelial dysplasia. Head Neck 2010;32:578-87. [PubMed]
- Hayes RB, Bravo-Otero E, Kleinman DV, et al. Tobacco and alcohol use and oral cancer in Puerto Rico. Cancer Causes Control 1999;10:27-33. [Crossref] [PubMed]
- Li L, Psoter WJ, Buxó CJ, et al. Smoking and drinking in relation to oral potentially malignant disorders in Puerto Rico: a case-control study. BMC Cancer 2011;11:324. [Crossref] [PubMed]
- Huang CT. Study on the Healthy Lifestyle and Related Factors of Police in Taipei City. Taipei: National Taiwan Normal University, 2009.
- Must A, Tybor DJ. Physical activity and sedentary behavior: a review of longitudinal studies of weight and adiposity in youth. Int J Obes (Lond) 2005;29:S84-96. [Crossref] [PubMed]
- Sun J, Cheng W, Fan Z, et al. Influence of high-intensity intermittent training on glycolipid metabolism in obese male college students. Ann Palliat Med 2020;9:2013-9. [Crossref] [PubMed]
- Ulijaszek SJ, Pentecost M, Marcus C, et al. Inequality and childhood overweight and obesity: a commentary. Pediatr Obes 2017;12:195-202. [Crossref] [PubMed]
- Kalak N, Brand S, Beck J, et al. Association between subjective actual sleep duration, subjective sleep need, age, body mass index, and gender in a large sample of young adults. Neuropsychiatr Dis Treat 2015;11:107-13. [PubMed]
- Ludy MJ, Tan SY, Leone RJ, et al. Weight gain in first-semester university students: Positive sleep and diet practices associated with protective effects. Physiol Behav 2018;194:132-6. [Crossref] [PubMed]
- Molina AJ, Varela V, Fernández T, et al. Unhealthy habits and practice of physical activity in Spanish college students: the role of gender, academic profile and living situation. Adicciones 2012;24:319-27. [Crossref] [PubMed]
- Thom G, Lean M. Who wants weight loss? What do they need? Time to re-think non-surgical approaches in obesity management. Clin Obes 2016;6:361-4. [Crossref] [PubMed]
- Taylor RW, Williams SM, Dawson AM, et al. Parental motivation to change body weight in young overweight children. Public Health Nutr 2015;18:1807-14. [Crossref] [PubMed]
- Campisi J, Finn KE, Bravo Y, et al. Sex and age-related differences in perceived, desired and measured percentage body fat among adults. J Hum Nutr Diet 2015;28:486-92. [Crossref] [PubMed]
- Jackson AS, Stanforth PR, Gagnon J, et al. The effect of sex, age and race on estimating percentage body fat from body mass index: The Heritage Family Study. Int J Obes Relat Metab Disord 2002;26:789-96. [Crossref] [PubMed]
- Avila-Palencia I, Laeremans M, Hoffmann B, et al. Effects of physical activity and air pollution on blood pressure. Environ Res 2019;173:387-96. [Crossref] [PubMed]
- Igarashi Y, Nogami Y. The effect of regular aquatic exercise on blood pressure: A meta-analysis of randomized controlled trials. Eur J Prev Cardiol 2018;25:190-9. [Crossref] [PubMed]
- Aadahl M, von Huth Smith L, Pisinger C, et al. Five-year change in physical activity is associated with changes in cardiovascular disease risk factors: the Inter99 study. Prev Med 2009;48:326-31. [Crossref] [PubMed]
- Zhang J, Chen G, Lu W, et al. Effects of physical exercise on health-related quality of life and blood lipids in perimenopausal women: a randomized placebo-controlled trial. Menopause 2014;21:1269-76. [Crossref] [PubMed]
- Lee YY, Kamarudin KS, Wan Muda WAM. Associations between self-reported and objectively measured physical activity and overweight/obesity among adults in Kota Bharu and Penang, Malaysia. BMC Public Health 2019;19:621. [Crossref] [PubMed]
- Chu AH, Ng SH, Koh D, et al. Reliability and Validity of the Self- and Interviewer-Administered Versions of the Global Physical Activity Questionnaire (GPAQ). PLoS One 2015;10:e0136944 [Crossref] [PubMed]
- Thyregod M, Bodtger U. Coherence between self-reported and objectively measured physical activity in patients with chronic obstructive lung disease: a systematic review. Int J Chron Obstruct Pulmon Dis 2016;11:2931-8. [Crossref] [PubMed]
- Reinehr T. Long-term effects of adolescent obesity: time to act. Nat Rev Endocrinol 2018;14:183-8. [Crossref] [PubMed]
(English Language Editor: A. Kassem)