Trajectories of posttraumatic growth in young and middle-aged stroke patients and their predictive effects on mental health
Introduction
According to the Chinese Stroke Prevention Report 2019, the incidence of stroke, which is a traumatic event, is on the rise in young and middle-aged adults (1). For young and middle-aged people who are at the most productive stage and have a long life expectancy, decline in social participation following a stroke incurs a considerable burden on patients, their families, and greater society. Patients can be exposed to negative emotional experiences, such as anxiety and depression, which undermine their physical and mental health and quality of life. Posttraumatic growth (PTG) refers to the positive psychological changes that can emerge as a result of struggling with adversity and trauma (2). Studies have shown that PTG improves health behaviors and promotes proactive coping, which helps to reduce stress and improve physical health (3-5). Most studies on PTG in young and middle-aged stroke patients have been cross-sectional, and few longitudinal studies have dynamically described PTG trajectories. Here, we attempted to investigate the effects of the developmental stage at the time of traumatic exposure and the developmental speed on the mental health of young and middle-aged stroke patients. It is speculated that the PTG stage and developmental rate can be used to predict the future mental health in this population.
We present the following article in accordance with the MDAR reporting checklist (available at https://dx.doi.org/10.21037/apm-21-1879).
Methods
Participants
A total of 170 patients treated at our center from January to December 2019 were enrolled via convenience sampling. The inclusion criteria were as follows: (I) aged 18 to 59 years, (II) diagnosed with acute ischemic stroke by cranial computed tomography (CT) or magnetic resonance imaging (MRI), (III) without mental disorder or severe physical disease and able to communicate smoothly, and (IV) with an informed and voluntary decision to participate in the survey. The exclusion criteria were as follows: (I) history any major life changes such as divorce and parental bereavement; (II) severe cognitive impairment; and (III) lost to two or more consecutive follow-up visits. The study was conducted in accordance with the Declaration of Helsinki (as revised in 2013). The study was approved by the medical ethics committee of Huizhou Municipal Central Hospital (approval No. 20190014).
Sample size calculation
According to the largest number of dimensions, three scales were included in this study. The Symptom Checklist-90 (SCL-90) had the largest number of dimensions, which was 10, and the sample size was 55–120. Because this study is a longitudinal design, considering the loss of follow-up rate of 20%, the sample size of this study should be more than 144 cases, and finally 165 cases were included.
Study methods
Survey tools
General data
The general data were collected using a self-designed general information questionnaire, which included variables including age, gender, education level, occupation, marital status, place of residence, mode of payment of medical fees, per capita monthly household income, and complications.
Posttraumatic Growth Inventory (PTGI)
The Chinese version (6) of the PTGI was used for assessing the PTG. The scale includes five dimensions (relating to others, new possibilities, personal strength, spiritual change, and appreciation of life) and 20 items. Each item is scored on a 6-point scale [0–5], with a score range of 0–100. A higher score indicates a higher level of trauma. The Cronbach’s α coefficient of PTGI was 0.886 in our current study.
PTGI is suitable for general trauma. It has the characteristics of multidimensional, simplicity, detailed and good reliability and validity indicators. It has good adaptability in basic and clinical application research.
Social Support Rating Scale (SSRS)
Social support was evaluated by the SSRS (7), which covers three dimensions (objective support, subjective support, and social support availability) and 10 items. Each item is scored on a 4-point scale [1–4], with a score range of 12–66 (although the score point is not limited for 2 items). A higher score indicates better social support. The Cronbach’s α coefficient of SSRS was 0.827 in our current study.
SCL-90
Mental health was evaluated by the SCL-90 Chinese version (8). The scale has 10 factors (including somatization, obsessive-compulsive, interpersonal sensitivity, depression, anxiety, hostility, phobic anxiety, paranoid ideation, psychoticism, and others) and 90 items, with a score range of 90–450. A higher score indicates more severe symptoms. According to the Chinese version, the SCL-90 score is considered positive if the total score is more than 160 points, if the total number of positive items is more than 43, or if any factor’s mean score is more than 2 points. The Cronbach’s α coefficient of SCL-90 was 0.814 in our current study.
Survey method
Physicians who had undergone uniform training and passed the qualification examination served as investigators. The content, purpose, significance, and privacy of this survey were explained to the patients before the survey, and informed consent was obtained from all participants. The survey was conducted at 5 time points (T1 to T5) for each patient: 1 day before discharge (T1) and then every 3 months thereafter (T2–T5). The questionnaire form was completed by the patients themselves or with the assistance of an investigator. A total of 170 patients were enrolled in this survey. Because 5 patients were lost to two or more consecutive follow-up visits, 165 patients were entered into the final analysis.
Statistical analysis
SPSS 21.0 statistical software (IBM Corporation, Armonk, NY, USA) was used to perform variance and trend tests for PTG levels at different time points. Latent growth mixture modeling (LGMM), which consists of continuous and categorical latent variables, was performed using Mplus 8.3 software. The continuous latent variables include intercept and slope, whereas the categorical latent variables divide a population into mutually exclusive and exhaustive latent profiles (9). Akaike’s information criterion (AIC), Bayesian information criterion (BIC), and sample-size-adjusted BIC (SABIC) were used to evaluate the goodness of fit of the model, with a small value indicating good fit. The categorical latent variables were tested using linear multivariate regression (LMR) and the bootstrap likelihood ratio test (BLRT), with a P value <0.05 indicating that model k was significantly better than model k-1. Entropy was used for assessing the classification accuracy, with a larger value (range: 0–1) indicating a more accurate classification (10). A P value <0.05 was considered significantly different.
Results
Descriptive statistics
The PTG levels in young and middle-aged stroke patients showed a gradual increase from T1 to T5 (F=9.927; P<0.01). The PTG levels showed moderate stability in all five measurements, and the correlation coefficients of PTG levels among these five measurements ranged from 0.569 to 0.960. Both social support and SCL-90 score showed significant correlations with PTG levels measured at 5 time points (all P<0.01; Table 1).
Table 1
Variables | N | Score () | T1 (r) | T2 (r) | T3 (r) | T4 (r) | T5 (r) | T1 social support | T5 SCL-90 |
---|---|---|---|---|---|---|---|---|---|
T1 | 165 | 46.70±11.54 | 1.000 | ||||||
T2 | 165 | 47.75±12.92 | 0.960a | 1.000 | |||||
T3 | 163 | 48.83±15.62 | 0.848a | 0.925a | 1.000 | ||||
T4 | 161 | 50.72±18.64 | 0.663a | 0.783a | 0.801a | 1.000 | |||
T5 | 158 | 54.89±23.08 | 0.569a | 0.709a | 0.739a | 0.759a | 1.000 | ||
T1 social support | 165 | 28.09±5.44 | 0.620a | 0.746a | 0.754a | 0.726a | 0.659a | 1.000 | |
T5 SCL-90 | 165 | 217.68±39.87 | −0.650a | −0.784a | −0.799a | −0.750a | −0.715a | −0.806a | 1.000 |
a, P<0.01. T1–T5: levels of PTG at different time points. PTG, posttraumatic growth; SCL-90, Symptom Checklist-90.
PTG trajectories in young and middle-aged stroke patients
The LGMM model was used to fit the heterogeneous trajectories of PTG (1, 2, 3, 4, and 5 classes) in young and middle-aged stroke patients, and the fitting results are shown in Table 2. For class 1 to 4, the information index constantly dropped as the number of classes increased; for class 5, both the LMR and BLRT values were larger than 0.05, indicating that class 5 was poorly fitted compared with class 4. According to the values of the fitted indexes and the practical significance of the potential classes, it was feasible to divide the heterogeneous trajectories of PTG in young and middle-aged stroke patients into four classes. According to the relevant parameters of the trajectories, the participants were divided into the high PTG group (n=43, 26.06%), low PTG group (n=25, 15.15%), rapidly increasing PTG group (n=47, 28.48%), and constantly decreasing PTG group (n=50, 30.30%), as shown in Figure 1. In the high PTG group, the PTG scores in the middle-aged and young stroke patients were high at baseline and maintained at high levels during the follow-up period (intercept =62.492, P<0.01; slope =2.354, P<0.01). In the rapidly increasing PTG group, the PTG scores in the middle-aged and young stroke patients showed a rising trend during the follow-up period (intercept =41.142, P<0.01; slope =3.562, P<0.01). In the constantly decreasing PTG group, the PTG scores in the middle-aged and young stroke patients showed a decreasing trend during the follow-up period (intercept =41.562, P<0.01; slope =−3.741, P<0.01). In the low PTG group, the PTG scores in the middle-aged and young stroke patients maintained at low levels during the follow-up period (intercept =41.128, P<0.01; slope =−1.216, P<0.01; Table 3).
Table 2
Model | AIC | BIC | aBIC | LMR | BLRT | Entropy |
---|---|---|---|---|---|---|
Linear models | ||||||
Class 1 | 5,768.629 | 5,799.689 | 5,768.029 | – | – | – |
Class 2 | 5,614.913 | 5,655.290 | 5,614.132 | 0.015 | 0.000 | 0.834 |
Class 3 | 5,559.180 | 5,608.875 | 5,558.219 | 0.033 | 0.005 | 0.852 |
Class 4 | 5,544.745 | 5,603.758 | 5,543.604 | 0.041 | 0.012 | 0.876 |
Class 5 | 5,550.177 | 5,618.508 | 5,578.856 | 0.371 | 0.114 | 0.841 |
Quadratic nonlinear models | ||||||
Class 1 | 5,765.906 | 5,809.390 | 5,765.066 | – | – | – |
Class 2 | 5,528.178 | 5,584.085 | 5,527.097 | 0.038 | 0.000 | 0.865 |
Class 3 | 5,377.880 | 5,446.211 | 5,376.559 | 0.026 | 0.002 | 0.874 |
Class 4 | 5,341.830 | 5,422.584 | 5,340.268 | 0.016 | 0.009 | 0.911 |
Class 5 | 5,391.203 | 5,454.381 | 5,369.401 | 0.077 | 0.104 | 0.892 |
Undefined curve models | ||||||
Class 1 | 5,772.340 | 5,809.611 | 5,771.619 | – | – | – |
Class 2 | 5,567.083 | 5,613.672 | 5,566.182 | 0.018 | 0.000 | 0.913 |
Class 3 | 5,561.229 | 5,617.136 | 5,560.148 | 0.032 | 0.005 | 0.930 |
Class 4 | 5,494.184 | 5,559.409 | 5,472.923 | 0.027 | 0.014 | 0.943 |
Class 5 | 5,487.449 | 5,561.992 | 5,486.008 | 0.547 | 0.230 | 0.934 |
LGMM, latent growth mixture modeling; AIC, Akaike’s information criterion; BIC, Bayesian information criterion; aBIC, adjusted BIC; LMR, linear multivariate regression; BLRT, bootstrap likelihood ratio test.
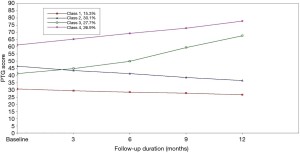
Table 3
Variables | Estimated value | Standard error |
|
P value |
---|---|---|---|---|
Class 1 (n=25) | ||||
Average value | ||||
Intercept | 41.128 | 1.045 | 39.365 | 0.000 |
Slope | −1.216 | 0.169 | 7.204 | 0.000 |
Var | ||||
Intercept | 74.692 | 11.970 | 6.240 | 0.000 |
Slope | 0.533 | 0.199 | 2.682 | 0.007 |
Class 2 (n=50) | ||||
Average value | ||||
Intercept | 41.562 | 5.816 | 7.147 | 0.000 |
Slope | −3.741 | 0.879 | 4.255 | 0.000 |
Var | ||||
Intercept | 59.142 | 9.164 | 6.454 | 0.000 |
Slope | 0.486 | 0.114 | 4.263 | 0.000 |
Class 3 (n=47) | ||||
Average value | ||||
Intercept | 41.142 | 1.236 | 33.290 | 0.000 |
Slope | 3.562 | 0.530 | 6.722 | 0.000 |
Var | ||||
Intercept | 64.508 | 10.206 | 6.320 | 0.000 |
Slope | 0.718 | 0.167 | 4.299 | 0.000 |
Class 4 (n=43) | ||||
Average value | ||||
Intercept | 62.492 | 1.261 | 49.541 | 0.000 |
Slope | 2.354 | 0.375 | 6.278 | 0.000 |
Var | ||||
Intercept | 71.336 | 10.755 | 6.633 | 0.000 |
Slope | 0.782 | 0.184 | 4.250 | 0.000 |
PTG, posttraumatic growth.
Predictive effects of initial level and developmental speed of PTG on mental health
The predictive roles of the initial level and developmental speed of PTG on mental health in young and middle-aged stroke patients was examined by using the SCL-90 score at T5 as the outcome variable and by adjusting for patients’ social support. The prediction model is shown in Figure 2. Model fitting results showed that the model provided an adequate fit to the data [χ2=36.282, degrees of freedom =18, P=0.624, χ2/df =2.016, comparative-fit-index (CFI) =0.935, Tucker-Lewis-index (TLI) =0.952, root mean square error of approximation (RMSEA) =0.285 (90% CI: 0.124, 0.389), and standardized root mean square residual (SRMR) =0.023]. Both the initial level and developmental rate of PTG had significantly negative predictive effects on SCL-90 score (B=−0.46, P<0.01; B=−0.31, P<0.01). Among the adjusted variables, social support had a significantly negative predictive effect on SCL-90 score (B=−0.34, P<0.01).
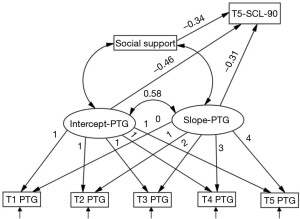
Discussion
PTG in young and middle-aged stroke patients
In our current study, the PTG scores were at low levels in young and middle-aged stroke patients (ranging from 46.70±11.54 to 54.89±23.08), which was consistent with the findings of Cui et al. (11) (PTG scores: 53.83±16.48) and Zhang et al. (12) (PTG scores: 54.79±18.99), indicating that the PTG levels in young and middle-aged stroke patients need to be further increased. PTG is a positive psychological change that can improve health behaviors, promote positive coping, and reduce negative emotions, which is beneficial to recovery (13). Young and middle-aged stroke patients need continuous care after discharge from hospital. They are often preoccupied with treatment and have no time to think about other things, such as looking into opportunities, re-establishing life goals, and trying new things. In addition, even if their clinical symptoms have been resolved, some patients still believe that they are seriously ill and have difficulty adapting to life after stroke. The absence of positive coping skills is not conducive to PTG. Therefore, the caregivers should help young and middle-aged patients to correctly understand stroke treatment and help them to improve their social support system, so that the patients can recognize the positive changes in themselves, others, and the outside society after the disease, which can promote their PTG in turn.
PTG trajectories in young and middle-aged stroke patients
PTG is a continuous process of development and change, as indicated by the different PTG scores of young and middle-aged stroke patients at the five different time points in our current survey. As shown by LGMM, the trajectories of PTG in young and middle-aged stroke patients could be divided into four qualitatively different potential classes: a high PTG group, low PTG group, rapidly increasing PTG group, and constantly decreasing PTG group. This distribution aligns with other results reported in the literature (14,15). The possible explanation for this may be as follows: patients in the high PTG group and the rapidly increasing PTG group tend to accept reality and adapt to the changes brought by the disease; they perceive the disease more rationally and consider the follow-up stroke treatment as an opportunity to extend their lives; they also actively contemplate the meaning of the stroke treatment event for themselves and focus more on positive emotions and growth gains. These features facilitate the patients to recover from the trauma and achieve higher levels of PTG (16). Long-term treatment increases the psychological stress of patients, resulting in many mental disorders in the low PTG group, which severely affects the PTG scores. Thus, special attention should be paid to patients with low PTG scores. Patients in the constantly decreasing PTG group need support and care from their families during disease treatment and in their daily lives, which makes them prone to a feeling of being a burden to their families and others (17). In addition, complications, medical expenses, and changes in living habits make them prone to anxiety, guilt, self-blame, and other adverse psychological reactions, which lower PTG (18). Therefore, individualized interventions should be offered for young and middle-aged stroke patients with different developmental trajectories. For patients in the high PTG group and rapidly increasing PTG group, high-level goals should be developed; in contrast, for patients in the low PTG group and constantly decreasing PTG group, small goals that are easier to achieve should be set for them to promote maximum personal growth.
Predictive effects of initial level and developmental speed of PTG on mental health in young and middle-aged stroke patients
As shown in our survey, both the initial level and developmental speed of PTG had positive predictive effects on mental health in young and middle-aged stroke patients. After adjusting for social support in young and middle-aged stroke patients, we found the PTG level at discharge (i.e., the initial level) was able to predict patients’ mental health 1 year later, which is consistent with other reports in the literature (19). The possible reason for this is that young and middle-aged stroke patients with high PTG levels are able to actively address various psychological stresses and difficulties brought about by the disease and treatment, try hard to adapt to their new life on their own, cope effectively with the disease with more mature developmental goals and meaningful coping styles, and thus optimize the physical and psychological outcomes (20). LGMM in our current study revealed that the developmental speed of PTG also significantly predicted the mental health of young and middle-aged stroke patients, suggesting that the developmental potential of PTG is a valid predictor of the level of mental health in young and middle-aged stroke patients and that PTG is a protective factor of mental health (21). PTG is a positive psychological change experienced after a struggle with stress. The continuous advances in stroke treatment technology have dramatically improved the outcomes of stroke in young and middle-aged patients. Meanwhile, the increased awareness and knowledge of stroke treatment among these patients, to a certain extent, lead to positive psychological changes, thus allowing patients to achieve significant mental health benefits.
In summary, there are different trajectories of PTG in young and middle-aged stroke patients, and the initial level and developmental rate of PTG have positive predictive effects on mental health in these patients. Caregivers should develop targeted and individualized interventions corresponding to these PTG trajectories. To help young and middle-aged stroke patients develop their self-awareness, encourage them to accept and face their own vulnerability, observe their own changes and consciously carry out cognitive processing, so as to stimulate the power of self-awareness and promote PTG. To encourage the growth of interpersonal experience of young and middle-aged stroke patients, in the later rehabilitation process of patients, medical staff can organize stroke patients to carry out some group communication activities in community or hospital, such as psychological games or lectures. Promote the growth of life value of young and middle-aged stroke patients, help them think about the significance of trauma, and make them face the future life with a more objective and positive attitude. Despite the interesting findings gleaned from our survey, some limitations to are study are worth noting: the sample size of the study was small and could not fully reflect the trajectories of PTG in young and middle-aged stroke patients; furthermore, only the trajectories of PTG in young and middle-aged stroke patients within 1 year were observed, and the dynamic changes after 1 year should be further observed in our future studies.
Acknowledgments
Funding: Supported by Huizhou Municipal Science and Technology Program (No. 2020Y095).
Footnote
Reporting Checklist: The authors have completed the MDAR reporting checklist. Available at https://dx.doi.org/10.21037/apm-21-1879
Data Sharing Statement: Available at https://dx.doi.org/10.21037/apm-21-1879
Conflicts of Interest: All authors have completed the ICMJE uniform disclosure form (available at http://dx.doi.org/10.21037/apm-21-1879). The authors have no conflicts of interest to declare.
Ethical Statement: The authors are accountable for all aspects of the work in ensuring that questions related to the accuracy or integrity of any part of the work are appropriately investigated and resolved. The study was conducted in accordance with the Declaration of Helsinki (as revised in 2013). The study was approved by the medical ethics committee of Huizhou Municipal Central Hospital (approval No. 20190014) and informed consent was taken from all the participants.
Open Access Statement: This is an Open Access article distributed in accordance with the Creative Commons Attribution-NonCommercial-NoDerivs 4.0 International License (CC BY-NC-ND 4.0), which permits the non-commercial replication and distribution of the article with the strict proviso that no changes or edits are made and the original work is properly cited (including links to both the formal publication through the relevant DOI and the license). See: https://creativecommons.org/licenses/by-nc-nd/4.0/.
References
- Report on Stroke Prevention and Treatment in China Writing Group. Brief report on stroke prevention and treatment in China, 2019. Chinese Journal of Cerebrovascular Diseases 2020;17:272-81.
- Qu JR, Zhang ZH, Zhao HY, et al. Research progress on posttraumatic growth of soldiers in war. Occupation and Health 2020;36:710-5.
- Yao C, Gao CY, Li LL, et al. Correlation between post-traumatic growth, rumination and social support in stroke survivors. Chinese Nursing Research 2019;33:3641-4.
- Liu LL, Li X, Lu XL, et al. Mediating role of resilience between hope level and post-traumatic growth in patients with acute myocardial infarction. Modern Preventive Medicine 2019;46:483-6.
- Lu CX, Lu LH. Correlation among psychological resilience, cognitive evaluation and post-traumatic growth in postoperative patients with liver cancer. Nursing Journal of Chinese People’s Liberation Army 2019;36:37-40, 44.
- Wang J, Chen Y, Wang YB, et al. Revision of the Posttraumatic Growth Inventory and testing its reliability and validity. Journal of Nursing Science 2011;26:26-8.
- Xiao SY. Theoretical basis and research application of social support rating scale. Journal of Clinical Psychological Medicine 1994;4:98-100.
- Wu WY, Jin H, Zhang MY. Application of symptom checklist-90 in neurosis evaluation. Chinese Journal of Psychiatry 1986;19:291-3.
- Yu JH, Chen XN, Gao YH, et al. The application of latent growth mixture modeling in the medical research. Chinese Journal of Health Statistics 2018;35:496-9.
- Wang MC. Latent variable modeling and Mplus application. Chongqing: Chongqing University Press, 2014.
- Cui CY, Jin CD. Correlation research among social support, coping style and posttraumatic growth for maintenance hemodialysis patients. Journal of Zunyi Medical University 2017;40:323-6, 332.
- Zhang RZ, Tao WW. The mediating role of experiential avoidance in the posttraumatic growth, self-consistency and congruence in hemodialysis patients. Chinese Journal of Blood Purification 2019;18:650-62.
- Qin RM, Lu CY, Zhou LH, et al. Current status of post-traumatic growth of gynecological cancer patients and its influence factors. Journal of Nursing 2019;26:9-13.
- Tan Y, Cui DS. Developmental trajectory of post traumatic growth in patients with bladder cancer: a latent growth mixture model. Chinese Journal of Practical Nursing 2020;36:1927-32.
- Ruiz de Alegría B, Basabe N, De Lorenzo E. Evolution of post-traumatic growth during the first 12 months of dialysis: a longitudinal study. J Ren Care 2017;43:108-13. [Crossref] [PubMed]
- Liu X, Li Q. Application of group psychotherapy in young and middle-aged patients undergoing maintenance hemodialysis. Chinese Journal of Modern Nursing 2019;25:3946-9.
- Zhang P, Gao P, Song XY, et al. Influencing factors of gratitude in hemodialysis patients with end-stage renal disease. Guangxi Medical Journal 2019;41:2800-3.
- Li T, Wang AM, Li ZY, et al. Correlation analysis of posttraumatic growth, resilience and rumination in hemodialysis patients. Journal of Nursing 2016;23:15-8.
- Chen Y. Effect of attentional bias and rumination on posttraumatic growth of people living with maintenance hemodialysis. Modern Clinical Nursing 2020;19:12-8.
- Li JJ, Liang XR, Xu QH, et al. Investigation on post-traumatic growth and social support in caregivers of maintenance hemodialysis patients. Chinese Journal of Blood Purification 2019;18:304-7.
- Hou WJ, Fan XG, Zhao FC. Research progress on clinical application of posttraumatic growth. Journal of International Psychiatry 2019;46:406-8, 416.
(English Language Editor: J. Gray)